Compare commits
189 Commits
kernel_dis
...
master
Author | SHA1 | Date | |
---|---|---|---|
|
391473adf3 | ||
|
0d8db31ff2 | ||
|
89b96f0a98 | ||
|
ee4cf583e3 | ||
|
6ed1b9a832 | ||
|
4a7d4a3d99 | ||
|
0626af207f | ||
|
7b23983887 | ||
|
0649d5bb45 | ||
|
339316aa7e | ||
|
2a85c94b55 | ||
|
6714cb7915 | ||
|
a501ab6c3b | ||
|
37add944b1 | ||
|
0d10fc7e25 | ||
|
71a2e74eff | ||
|
85f75bb28c | ||
|
46ff1c4eb1 | ||
|
ed5b9b6c62 | ||
|
08b3f9bbb9 | ||
|
784a963527 | ||
|
236cbbc4d2 | ||
|
695559fd4a | ||
|
a54acdef22 | ||
|
bebd13868f | ||
|
62df3c0457 | ||
|
cce76c7940 | ||
|
ca24422ab0 | ||
|
a28601751e | ||
|
07a2d6caaa | ||
|
3d3d27fbab | ||
|
b49b7a2d41 | ||
|
b6e8242383 | ||
|
cd616d11b9 | ||
|
afcfcb8973 | ||
|
bf03a45475 | ||
|
083b5c1597 | ||
|
7f0a8e9bce | ||
|
bf09ff8f7f | ||
|
c1d7cfee8f | ||
|
99be965581 | ||
|
fdb9a7c66d | ||
|
eb79b703d8 | ||
|
bc9a826b7d | ||
|
cfe09ec06b | ||
|
3d76dffe3c | ||
|
597c9fc1ee | ||
|
a8c74a1a6f | ||
|
f78ff1a464 | ||
|
5a3dbfac2e | ||
|
478a3c2cfe | ||
|
4520fdde8e | ||
|
b90044b86c | ||
|
a1310df4ee | ||
|
5dc66494ea | ||
|
74d420a77d | ||
|
6ffd14e85c | ||
|
40c1021c20 | ||
|
acf3272fd7 | ||
|
c73f8e7a28 | ||
|
de61bf7bca | ||
|
ae11fedbf3 | ||
|
11cd1b0032 | ||
|
7a6da0c5fc | ||
|
bf23d5f7f8 | ||
|
bcde3f6ac8 | ||
|
d5229b1750 | ||
|
fc4b143fbb | ||
|
11cfa79746 | ||
|
d0ae94f2af | ||
|
2c908a8361 | ||
|
c95f91cc29 | ||
|
7763a57058 | ||
|
454718cdf5 | ||
|
70b4fa07e6 | ||
|
3a0e4a081e | ||
|
42eb53d73a | ||
|
6e8a52e371 | ||
|
0f450ed8a0 | ||
|
1f458ac0cc | ||
|
d45e71256c | ||
|
fc9edeaa97 | ||
|
549e6a10c1 | ||
|
9241475570 | ||
|
083cc929be | ||
|
668c9a1fb7 | ||
|
d2d6f31e7b | ||
|
6ad665f8c2 | ||
|
2af1da7f23 | ||
|
84e08955f7 | ||
|
935d9fa7ad | ||
|
d26a626677 | ||
|
b4ad870b7c | ||
|
38244f6691 | ||
|
1ba7f5c4f7 | ||
|
a30672b932 | ||
|
093a79d533 | ||
|
25dbde4e43 | ||
|
5dddb39ec4 | ||
|
b8969347b1 | ||
|
dfefd128c4 | ||
|
5e72fd8187 | ||
|
4a99bcbf0d | ||
|
44e4709387 | ||
|
0b2aaa42b8 | ||
|
abae72d624 | ||
|
92b8d1785c | ||
|
56d554ed83 | ||
|
396d569351 | ||
|
24903b761c | ||
|
e4257ec1f1 | ||
|
aaad2b8626 | ||
|
c0c0044a42 | ||
|
47d7f5831f | ||
|
4f1c879528 | ||
|
2272c55092 | ||
|
b03c9b1d3c | ||
|
0c28eda706 | ||
|
7bc0bfa3ab | ||
|
827958a28a | ||
|
8200e1d3d8 | ||
|
729b20e9ab | ||
|
ca8ac7a43b | ||
|
b724a28a6f | ||
|
1e0a8392a2 | ||
|
2eb7b05653 | ||
|
d8a0b2dfcc | ||
|
2a7394b593 | ||
|
b1e64c8b8b | ||
|
70cf17607e | ||
|
b1568a550a | ||
|
e8e803e8ef | ||
|
2c453265fe | ||
|
7336d35fee | ||
|
bc18952c05 | ||
|
8e8d0b9c2c | ||
|
5a7da2b40b | ||
|
b6d38f442b | ||
|
8e8851d962 | ||
|
27b43b06a7 | ||
|
ff69eb1256 | ||
|
4ca581909a | ||
|
2722d976f5 | ||
|
946cda00d2 | ||
|
8227525c82 | ||
|
e61ae73749 | ||
|
040d1ee9e8 | ||
|
7f0da894fa | ||
|
62726df278 | ||
|
0ba09db6fe | ||
|
87334c11e6 | ||
|
40ef3aeda2 | ||
|
94fe4435a8 | ||
|
c204bc8e1f | ||
|
00615ae837 | ||
|
9f5f0d12dd | ||
|
8a291f7bfb | ||
|
21e3e3b82d | ||
|
a6bd6e130a | ||
|
fcdfa52892 | ||
|
73e6fe384e | ||
|
aff7a385a3 | ||
|
1e23ba05fa | ||
|
ee30d4da5b | ||
|
14508f0600 | ||
|
e3f8828da4 | ||
|
30adbf705c | ||
|
ee42fd68b1 | ||
|
736d9a6349 | ||
|
0055e15bc1 | ||
|
b2e1df7308 | ||
|
b935e9caf3 | ||
|
503ef0e05f | ||
|
dc6248413c | ||
|
e73b70ceb7 | ||
|
639198e774 | ||
|
768d969f89 | ||
|
aec422c277 | ||
|
6c14170de6 | ||
|
36a330aa66 | ||
|
acd4ac6a86 | ||
|
abe64cfe8f | ||
|
caae95d01d | ||
|
088429a16a | ||
|
b6145223c8 | ||
|
09256956f3 | ||
|
0ca90fdcee | ||
|
be21412f8a | ||
|
ae6bc47f87 |
@ -1,10 +1,10 @@
|
|||||||
[bumpversion]
|
[bumpversion]
|
||||||
current_version = 0.4.2
|
current_version = 0.7.6
|
||||||
commit = True
|
commit = True
|
||||||
tag = True
|
tag = True
|
||||||
parse = (?P<major>\d+)\.(?P<minor>\d+)\.(?P<patch>\d+)
|
parse = (?P<major>\d+)\.(?P<minor>\d+)\.(?P<patch>\d+)
|
||||||
serialize =
|
serialize = {major}.{minor}.{patch}
|
||||||
{major}.{minor}.{patch}
|
message = build: bump version {current_version} → {new_version}
|
||||||
|
|
||||||
[bumpversion:file:setup.py]
|
[bumpversion:file:setup.py]
|
||||||
|
|
||||||
|
15
.codacy.yml
15
.codacy.yml
@ -1,15 +0,0 @@
|
|||||||
# To validate the contents of your configuration file
|
|
||||||
# run the following command in the folder where the configuration file is located:
|
|
||||||
# codacy-analysis-cli validate-configuration --directory `pwd`
|
|
||||||
# To analyse, run:
|
|
||||||
# codacy-analysis-cli analyse --tool remark-lint --directory `pwd`
|
|
||||||
---
|
|
||||||
engines:
|
|
||||||
pylintpython3:
|
|
||||||
exclude_paths:
|
|
||||||
- config/engines.yml
|
|
||||||
remark-lint:
|
|
||||||
exclude_paths:
|
|
||||||
- config/engines.yml
|
|
||||||
exclude_paths:
|
|
||||||
- 'tests/**'
|
|
@ -1,2 +0,0 @@
|
|||||||
comment:
|
|
||||||
require_changes: yes
|
|
19
.github/ISSUE_TEMPLATE/bug_report.md
vendored
19
.github/ISSUE_TEMPLATE/bug_report.md
vendored
@ -10,21 +10,28 @@ assignees: ''
|
|||||||
**Describe the bug**
|
**Describe the bug**
|
||||||
A clear and concise description of what the bug is.
|
A clear and concise description of what the bug is.
|
||||||
|
|
||||||
**To Reproduce**
|
**Steps to reproduce the behavior**
|
||||||
Steps to reproduce the behavior:
|
1. ...
|
||||||
1. Install Prototorch by running '...'
|
2. Run script '...' or this snippet:
|
||||||
2. Run script '...'
|
```python
|
||||||
|
import prototorch as pt
|
||||||
|
|
||||||
|
...
|
||||||
|
```
|
||||||
3. See errors
|
3. See errors
|
||||||
|
|
||||||
**Expected behavior**
|
**Expected behavior**
|
||||||
A clear and concise description of what you expected to happen.
|
A clear and concise description of what you expected to happen.
|
||||||
|
|
||||||
|
**Observed behavior**
|
||||||
|
A clear and concise description of what actually happened.
|
||||||
|
|
||||||
**Screenshots**
|
**Screenshots**
|
||||||
If applicable, add screenshots to help explain your problem.
|
If applicable, add screenshots to help explain your problem.
|
||||||
|
|
||||||
**Desktop (please complete the following information):**
|
**System and version information**
|
||||||
- OS: [e.g. Ubuntu 20.10]
|
- OS: [e.g. Ubuntu 20.10]
|
||||||
- Prototorch Version: [e.g. v0.4.0]
|
- ProtoTorch Version: [e.g. 0.4.0]
|
||||||
- Python Version: [e.g. 3.9.5]
|
- Python Version: [e.g. 3.9.5]
|
||||||
|
|
||||||
**Additional context**
|
**Additional context**
|
||||||
|
68
.github/workflows/pythonapp.yml
vendored
68
.github/workflows/pythonapp.yml
vendored
@ -5,33 +5,71 @@ name: tests
|
|||||||
|
|
||||||
on:
|
on:
|
||||||
push:
|
push:
|
||||||
branches: [ master, dev ]
|
|
||||||
pull_request:
|
pull_request:
|
||||||
branches: [master]
|
branches: [master]
|
||||||
|
|
||||||
jobs:
|
jobs:
|
||||||
build:
|
style:
|
||||||
|
|
||||||
runs-on: ubuntu-latest
|
runs-on: ubuntu-latest
|
||||||
|
|
||||||
steps:
|
steps:
|
||||||
- uses: actions/checkout@v2
|
- uses: actions/checkout@v3
|
||||||
- name: Set up Python 3.8
|
- name: Set up Python 3.11
|
||||||
uses: actions/setup-python@v1
|
uses: actions/setup-python@v4
|
||||||
with:
|
with:
|
||||||
python-version: 3.8
|
python-version: "3.11"
|
||||||
- name: Install dependencies
|
- name: Install dependencies
|
||||||
run: |
|
run: |
|
||||||
python -m pip install --upgrade pip
|
python -m pip install --upgrade pip
|
||||||
pip install .[all]
|
pip install .[all]
|
||||||
- name: Lint with flake8
|
- uses: pre-commit/action@v3.0.0
|
||||||
|
compatibility:
|
||||||
|
needs: style
|
||||||
|
strategy:
|
||||||
|
fail-fast: false
|
||||||
|
matrix:
|
||||||
|
python-version: ["3.8", "3.9", "3.10", "3.11"]
|
||||||
|
os: [ubuntu-latest, windows-latest]
|
||||||
|
exclude:
|
||||||
|
- os: windows-latest
|
||||||
|
python-version: "3.8"
|
||||||
|
- os: windows-latest
|
||||||
|
python-version: "3.9"
|
||||||
|
- os: windows-latest
|
||||||
|
python-version: "3.10"
|
||||||
|
|
||||||
|
runs-on: ${{ matrix.os }}
|
||||||
|
steps:
|
||||||
|
- uses: actions/checkout@v3
|
||||||
|
- name: Set up Python ${{ matrix.python-version }}
|
||||||
|
uses: actions/setup-python@v4
|
||||||
|
with:
|
||||||
|
python-version: ${{ matrix.python-version }}
|
||||||
|
- name: Install dependencies
|
||||||
run: |
|
run: |
|
||||||
pip install flake8
|
python -m pip install --upgrade pip
|
||||||
# stop the build if there are Python syntax errors or undefined names
|
pip install .[all]
|
||||||
flake8 . --count --select=E9,F63,F7,F82 --show-source --statistics
|
|
||||||
# exit-zero treats all errors as warnings. The GitHub editor is 127 chars wide
|
|
||||||
flake8 . --count --exit-zero --max-complexity=10 --max-line-length=127 --statistics
|
|
||||||
- name: Test with pytest
|
- name: Test with pytest
|
||||||
run: |
|
run: |
|
||||||
pip install pytest
|
|
||||||
pytest
|
pytest
|
||||||
|
publish_pypi:
|
||||||
|
if: github.event_name == 'push' && startsWith(github.ref, 'refs/tags')
|
||||||
|
needs: compatibility
|
||||||
|
runs-on: ubuntu-latest
|
||||||
|
steps:
|
||||||
|
- uses: actions/checkout@v3
|
||||||
|
- name: Set up Python 3.10
|
||||||
|
uses: actions/setup-python@v4
|
||||||
|
with:
|
||||||
|
python-version: "3.11"
|
||||||
|
- name: Install dependencies
|
||||||
|
run: |
|
||||||
|
python -m pip install --upgrade pip
|
||||||
|
pip install .[all]
|
||||||
|
pip install wheel
|
||||||
|
- name: Build package
|
||||||
|
run: python setup.py sdist bdist_wheel
|
||||||
|
- name: Publish a Python distribution to PyPI
|
||||||
|
uses: pypa/gh-action-pypi-publish@release/v1
|
||||||
|
with:
|
||||||
|
user: __token__
|
||||||
|
password: ${{ secrets.PYPI_API_TOKEN }}
|
||||||
|
17
.gitignore
vendored
17
.gitignore
vendored
@ -129,14 +129,6 @@ dmypy.json
|
|||||||
|
|
||||||
# End of https://www.gitignore.io/api/python
|
# End of https://www.gitignore.io/api/python
|
||||||
|
|
||||||
# ProtoFlow
|
|
||||||
core
|
|
||||||
checkpoint
|
|
||||||
logs/
|
|
||||||
saved_weights/
|
|
||||||
scratch*
|
|
||||||
|
|
||||||
|
|
||||||
# Created by https://www.gitignore.io/api/visualstudiocode
|
# Created by https://www.gitignore.io/api/visualstudiocode
|
||||||
# Edit at https://www.gitignore.io/?templates=visualstudiocode
|
# Edit at https://www.gitignore.io/?templates=visualstudiocode
|
||||||
|
|
||||||
@ -154,4 +146,13 @@ scratch*
|
|||||||
# End of https://www.gitignore.io/api/visualstudiocode
|
# End of https://www.gitignore.io/api/visualstudiocode
|
||||||
.vscode/
|
.vscode/
|
||||||
|
|
||||||
|
# Vim
|
||||||
|
*~
|
||||||
|
*.swp
|
||||||
|
*.swo
|
||||||
|
|
||||||
|
# Artifacts created by ProtoTorch
|
||||||
reports
|
reports
|
||||||
|
artifacts
|
||||||
|
examples/_*.py
|
||||||
|
examples/_*.ipynb
|
||||||
|
53
.pre-commit-config.yaml
Normal file
53
.pre-commit-config.yaml
Normal file
@ -0,0 +1,53 @@
|
|||||||
|
# See https://pre-commit.com for more information
|
||||||
|
# See https://pre-commit.com/hooks.html for more hooks
|
||||||
|
|
||||||
|
repos:
|
||||||
|
- repo: https://github.com/pre-commit/pre-commit-hooks
|
||||||
|
rev: v4.4.0
|
||||||
|
hooks:
|
||||||
|
- id: trailing-whitespace
|
||||||
|
- id: end-of-file-fixer
|
||||||
|
- id: check-yaml
|
||||||
|
- id: check-added-large-files
|
||||||
|
- id: check-ast
|
||||||
|
- id: check-case-conflict
|
||||||
|
|
||||||
|
- repo: https://github.com/myint/autoflake
|
||||||
|
rev: v2.1.1
|
||||||
|
hooks:
|
||||||
|
- id: autoflake
|
||||||
|
|
||||||
|
- repo: http://github.com/PyCQA/isort
|
||||||
|
rev: 5.12.0
|
||||||
|
hooks:
|
||||||
|
- id: isort
|
||||||
|
|
||||||
|
- repo: https://github.com/pre-commit/mirrors-mypy
|
||||||
|
rev: v1.3.0
|
||||||
|
hooks:
|
||||||
|
- id: mypy
|
||||||
|
files: prototorch
|
||||||
|
additional_dependencies: [types-pkg_resources]
|
||||||
|
|
||||||
|
- repo: https://github.com/pre-commit/mirrors-yapf
|
||||||
|
rev: v0.32.0
|
||||||
|
hooks:
|
||||||
|
- id: yapf
|
||||||
|
|
||||||
|
- repo: https://github.com/pre-commit/pygrep-hooks
|
||||||
|
rev: v1.10.0
|
||||||
|
hooks:
|
||||||
|
- id: python-use-type-annotations
|
||||||
|
- id: python-no-log-warn
|
||||||
|
- id: python-check-blanket-noqa
|
||||||
|
|
||||||
|
- repo: https://github.com/asottile/pyupgrade
|
||||||
|
rev: v3.7.0
|
||||||
|
hooks:
|
||||||
|
- id: pyupgrade
|
||||||
|
|
||||||
|
- repo: https://github.com/si-cim/gitlint
|
||||||
|
rev: v0.15.2-unofficial
|
||||||
|
hooks:
|
||||||
|
- id: gitlint
|
||||||
|
args: [--contrib=CT1, --ignore=B6, --msg-filename]
|
@ -19,7 +19,7 @@ formats: all
|
|||||||
|
|
||||||
# Optionally set the version of Python and requirements required to build your docs
|
# Optionally set the version of Python and requirements required to build your docs
|
||||||
python:
|
python:
|
||||||
version: 3.8
|
version: 3.9
|
||||||
install:
|
install:
|
||||||
- method: pip
|
- method: pip
|
||||||
path: .
|
path: .
|
||||||
|
7
.remarkrc
Normal file
7
.remarkrc
Normal file
@ -0,0 +1,7 @@
|
|||||||
|
{
|
||||||
|
"plugins": [
|
||||||
|
"remark-preset-lint-recommended",
|
||||||
|
["remark-lint-list-item-indent", false],
|
||||||
|
["no-emphasis-as-header", false]
|
||||||
|
]
|
||||||
|
}
|
34
.travis.yml
34
.travis.yml
@ -1,34 +0,0 @@
|
|||||||
dist: bionic
|
|
||||||
sudo: false
|
|
||||||
language: python
|
|
||||||
python: 3.8
|
|
||||||
cache:
|
|
||||||
directories:
|
|
||||||
- "./tests/artifacts"
|
|
||||||
install:
|
|
||||||
- pip install .[all] --progress-bar off
|
|
||||||
|
|
||||||
# Generate code coverage report
|
|
||||||
script:
|
|
||||||
- coverage run -m pytest
|
|
||||||
|
|
||||||
# Push the results to codecov
|
|
||||||
after_success:
|
|
||||||
- bash <(curl -s https://codecov.io/bash)
|
|
||||||
|
|
||||||
# Publish on PyPI
|
|
||||||
deploy:
|
|
||||||
provider: pypi
|
|
||||||
username: __token__
|
|
||||||
password:
|
|
||||||
secure: rVQNCxKIuiEtMz4zLSsjdt6spG7cf3miKN5eqjxZfcELALHxAV4w/+CideQObOn3u9emmxb87R9XWKcogqK2MXqnuIcY4mWg7HUqaip1bhz/4YiVXjFILcG6itjX9IUF1DrtjKKRk6xryucSZcEB7yTcXz1hQTb768KWlLlKOVTRNwr7j07eyeafexz/L2ANQCqfOZgS4b0k2AMeDBRPykPULtyeneEFlb6MJZ2MxeqtTNVK4b/6VsQSZwQ9jGJNGWonn5Y287gHmzvEcymSJogTe2taxGBWawPnOsibws9v88DEAHdsEvYdnqEE3hFl0R5La2Lkjd8CjNUYegxioQ57i3WNS3iksq10ZLMCbH29lb9YPG7r6Y8z9H85735kV2gKLdf+o7SPS03TRgjSZKN6pn4pLG0VWkxC6l8VfLuJnRNTHX4g6oLQwOWIBbxybn9Zw/yLjAXAJNgBHt5v86H6Jfi1Va4AhEV6itkoH9IM3/uDhrE/mmorqyVled/CPNtBWNTyoDevLNxMUDnbuhH0JzLki+VOjKnTxEfq12JB8X9faFG5BjvU9oGjPPewrp5DGGzg6KDra7dikciWUxE1eTFFDhMyG1CFGcjKlDvlAGHyI6Kih35egGUeq+N/pitr2330ftM9Dm4rWpOTxPyCI89bXKssx/MgmLG7kSM=
|
|
||||||
on:
|
|
||||||
tags: true
|
|
||||||
skip_existing: true
|
|
||||||
|
|
||||||
# The password is encrypted with:
|
|
||||||
# `cd prototorch && travis encrypt your-pypi-api-token --add deploy.password`
|
|
||||||
# See https://docs.travis-ci.com/user/deployment/pypi and
|
|
||||||
# https://github.com/travis-ci/travis.rb#installation
|
|
||||||
# for more details
|
|
||||||
# Note: The encrypt command does not work well in ZSH.
|
|
3
LICENSE
3
LICENSE
@ -1,6 +1,7 @@
|
|||||||
MIT License
|
MIT License
|
||||||
|
|
||||||
Copyright (c) 2020 si-cim
|
Copyright (c) 2020 Saxon Institute for Computational Intelligence and Machine
|
||||||
|
Learning (SICIM)
|
||||||
|
|
||||||
Permission is hereby granted, free of charge, to any person obtaining a copy
|
Permission is hereby granted, free of charge, to any person obtaining a copy
|
||||||
of this software and associated documentation files (the "Software"), to deal
|
of this software and associated documentation files (the "Software"), to deal
|
||||||
|
21
README.md
21
README.md
@ -2,13 +2,9 @@
|
|||||||
|
|
||||||
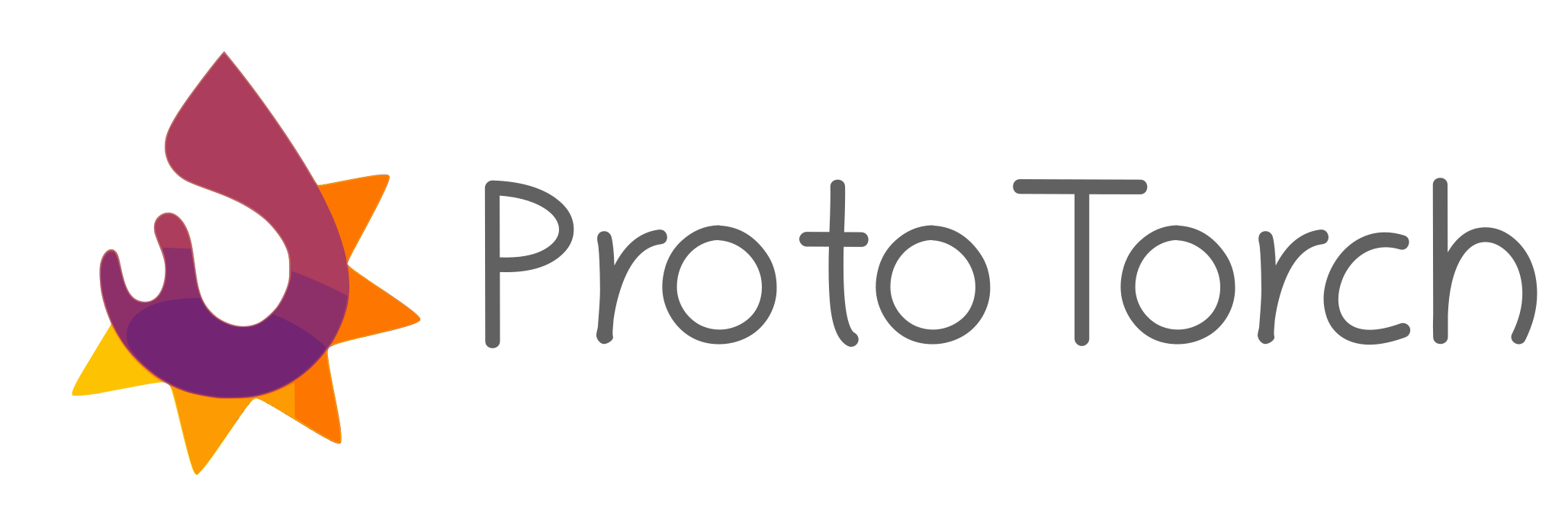
|
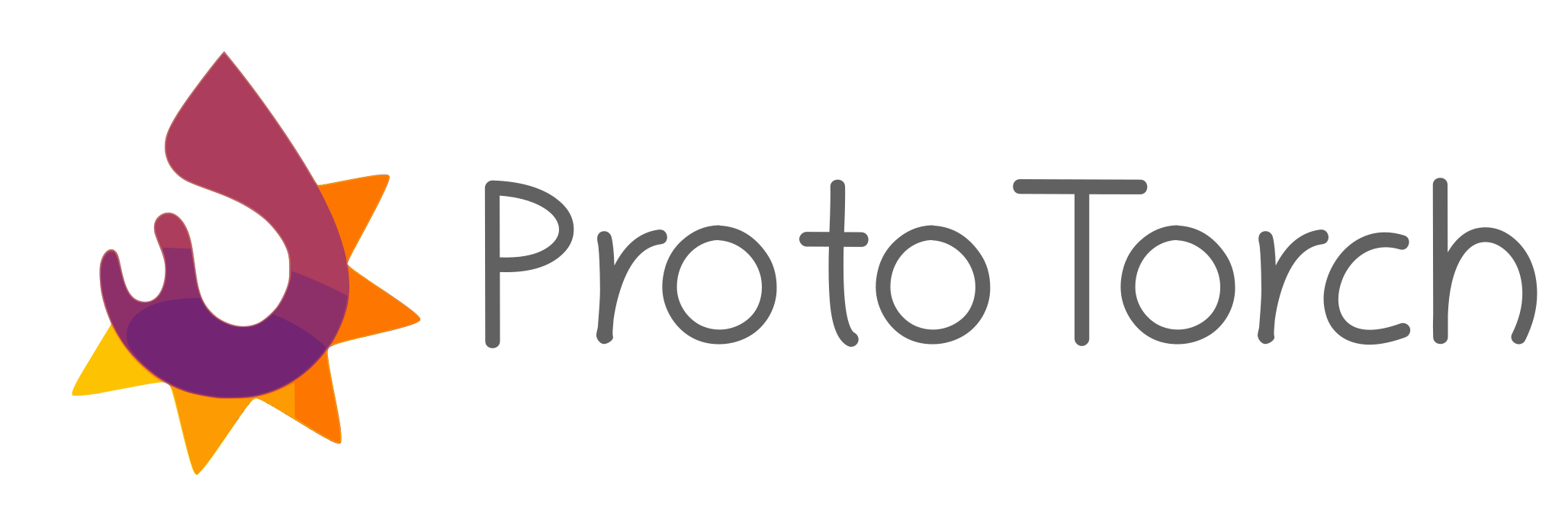
|
||||||
|
|
||||||
[](https://travis-ci.org/si-cim/prototorch)
|
|
||||||

|

|
||||||
[](https://github.com/si-cim/prototorch/releases)
|
[](https://github.com/si-cim/prototorch/releases)
|
||||||
[](https://pypi.org/project/prototorch/)
|
[](https://pypi.org/project/prototorch/)
|
||||||
[](https://codecov.io/gh/si-cim/prototorch)
|
|
||||||
[](https://www.codacy.com/gh/si-cim/prototorch?utm_source=github.com&utm_medium=referral&utm_content=si-cim/prototorch&utm_campaign=Badge_Grade)
|
|
||||||

|
|
||||||
[](https://github.com/si-cim/prototorch/blob/master/LICENSE)
|
[](https://github.com/si-cim/prototorch/blob/master/LICENSE)
|
||||||
|
|
||||||
*Tensorflow users, see:* [ProtoFlow](https://github.com/si-cim/protoflow)
|
*Tensorflow users, see:* [ProtoFlow](https://github.com/si-cim/protoflow)
|
||||||
@ -48,6 +44,23 @@ pip install -e .[all]
|
|||||||
The documentation is available at <https://www.prototorch.ml/en/latest/>. Should
|
The documentation is available at <https://www.prototorch.ml/en/latest/>. Should
|
||||||
that link not work try <https://prototorch.readthedocs.io/en/latest/>.
|
that link not work try <https://prototorch.readthedocs.io/en/latest/>.
|
||||||
|
|
||||||
|
## Contribution
|
||||||
|
|
||||||
|
This repository contains definition for [git hooks](https://githooks.com).
|
||||||
|
[Pre-commit](https://pre-commit.com) is automatically installed as development
|
||||||
|
dependency with prototorch or you can install it manually with `pip install
|
||||||
|
pre-commit`.
|
||||||
|
|
||||||
|
Please install the hooks by running:
|
||||||
|
```bash
|
||||||
|
pre-commit install
|
||||||
|
pre-commit install --hook-type commit-msg
|
||||||
|
```
|
||||||
|
before creating the first commit.
|
||||||
|
|
||||||
|
The commit will fail if the commit message does not follow the specification
|
||||||
|
provided [here](https://www.conventionalcommits.org/en/v1.0.0/#specification).
|
||||||
|
|
||||||
## Bibtex
|
## Bibtex
|
||||||
|
|
||||||
If you would like to cite the package, please use this:
|
If you would like to cite the package, please use this:
|
||||||
|
@ -1,13 +1,16 @@
|
|||||||
# ProtoTorch Releases
|
# ProtoTorch Releases
|
||||||
|
|
||||||
|
## Release 0.5.0
|
||||||
|
|
||||||
|
- Breaking: Removed deprecated `prototorch.modules.Prototypes1D`.
|
||||||
|
- Use `prototorch.components.LabeledComponents` instead.
|
||||||
|
|
||||||
## Release 0.2.0
|
## Release 0.2.0
|
||||||
|
|
||||||
### Includes
|
|
||||||
- Fixes in example scripts.
|
- Fixes in example scripts.
|
||||||
|
|
||||||
## Release 0.1.1-dev0
|
## Release 0.1.1-dev0
|
||||||
|
|
||||||
### Includes
|
|
||||||
- Minor bugfixes.
|
- Minor bugfixes.
|
||||||
- 100% line coverage.
|
- 100% line coverage.
|
||||||
|
|
||||||
|
@ -1,13 +1,24 @@
|
|||||||
.. ProtoFlow API Reference
|
.. ProtoTorch API Reference
|
||||||
|
|
||||||
ProtoFlow API Reference
|
ProtoTorch API Reference
|
||||||
======================================
|
======================================
|
||||||
|
|
||||||
Datasets
|
Datasets
|
||||||
--------------------------------------
|
--------------------------------------
|
||||||
|
|
||||||
|
Common Datasets
|
||||||
|
~~~~~~~~~~~~~~~~~~~~~~~~~~~~~~~~~~~~~~
|
||||||
.. automodule:: prototorch.datasets
|
.. automodule:: prototorch.datasets
|
||||||
:members:
|
:members:
|
||||||
:undoc-members:
|
|
||||||
|
|
||||||
|
Abstract Datasets
|
||||||
|
~~~~~~~~~~~~~~~~~~~~~~~~~~~~~~~~~~~~~~
|
||||||
|
|
||||||
|
Abstract Datasets are used to build your own datasets.
|
||||||
|
|
||||||
|
.. autoclass:: prototorch.datasets.abstract.NumpyDataset
|
||||||
|
:members:
|
||||||
|
|
||||||
Functions
|
Functions
|
||||||
--------------------------------------
|
--------------------------------------
|
||||||
|
@ -23,7 +23,7 @@ author = "Jensun Ravichandran"
|
|||||||
|
|
||||||
# The full version, including alpha/beta/rc tags
|
# The full version, including alpha/beta/rc tags
|
||||||
#
|
#
|
||||||
release = "0.4.2"
|
release = "0.7.6"
|
||||||
|
|
||||||
# -- General configuration ---------------------------------------------------
|
# -- General configuration ---------------------------------------------------
|
||||||
|
|
||||||
@ -46,6 +46,7 @@ extensions = [
|
|||||||
"sphinx.ext.viewcode",
|
"sphinx.ext.viewcode",
|
||||||
"sphinx_rtd_theme",
|
"sphinx_rtd_theme",
|
||||||
"sphinxcontrib.katex",
|
"sphinxcontrib.katex",
|
||||||
|
'sphinx_autodoc_typehints',
|
||||||
]
|
]
|
||||||
|
|
||||||
# katex_prerender = True
|
# katex_prerender = True
|
||||||
@ -119,7 +120,7 @@ html_css_files = [
|
|||||||
# -- Options for HTMLHelp output ------------------------------------------
|
# -- Options for HTMLHelp output ------------------------------------------
|
||||||
|
|
||||||
# Output file base name for HTML help builder.
|
# Output file base name for HTML help builder.
|
||||||
htmlhelp_basename = "protoflowdoc"
|
htmlhelp_basename = "prototorchdoc"
|
||||||
|
|
||||||
# -- Options for LaTeX output ---------------------------------------------
|
# -- Options for LaTeX output ---------------------------------------------
|
||||||
|
|
||||||
@ -179,6 +180,9 @@ texinfo_documents = [
|
|||||||
intersphinx_mapping = {
|
intersphinx_mapping = {
|
||||||
"python": ("https://docs.python.org/", None),
|
"python": ("https://docs.python.org/", None),
|
||||||
"numpy": ("https://docs.scipy.org/doc/numpy/", None),
|
"numpy": ("https://docs.scipy.org/doc/numpy/", None),
|
||||||
|
"torch": ('https://pytorch.org/docs/stable/', None),
|
||||||
|
"pytorch_lightning":
|
||||||
|
("https://pytorch-lightning.readthedocs.io/en/stable/", None),
|
||||||
}
|
}
|
||||||
|
|
||||||
# -- Options for Epub output ----------------------------------------------
|
# -- Options for Epub output ----------------------------------------------
|
||||||
|
100
examples/cbc_iris.py
Normal file
100
examples/cbc_iris.py
Normal file
@ -0,0 +1,100 @@
|
|||||||
|
"""ProtoTorch CBC example using 2D Iris data."""
|
||||||
|
|
||||||
|
import logging
|
||||||
|
|
||||||
|
import torch
|
||||||
|
from matplotlib import pyplot as plt
|
||||||
|
|
||||||
|
import prototorch as pt
|
||||||
|
|
||||||
|
|
||||||
|
class CBC(torch.nn.Module):
|
||||||
|
|
||||||
|
def __init__(self, data, **kwargs):
|
||||||
|
super().__init__(**kwargs)
|
||||||
|
self.components_layer = pt.components.ReasoningComponents(
|
||||||
|
distribution=[2, 1, 2],
|
||||||
|
components_initializer=pt.initializers.SSCI(data, noise=0.1),
|
||||||
|
reasonings_initializer=pt.initializers.PPRI(components_first=True),
|
||||||
|
)
|
||||||
|
|
||||||
|
def forward(self, x):
|
||||||
|
components, reasonings = self.components_layer()
|
||||||
|
sims = pt.similarities.euclidean_similarity(x, components)
|
||||||
|
probs = pt.competitions.cbcc(sims, reasonings)
|
||||||
|
return probs
|
||||||
|
|
||||||
|
|
||||||
|
class VisCBC2D():
|
||||||
|
|
||||||
|
def __init__(self, model, data):
|
||||||
|
self.model = model
|
||||||
|
self.x_train, self.y_train = pt.utils.parse_data_arg(data)
|
||||||
|
self.title = "Components Visualization"
|
||||||
|
self.fig = plt.figure(self.title)
|
||||||
|
self.border = 0.1
|
||||||
|
self.resolution = 100
|
||||||
|
self.cmap = "viridis"
|
||||||
|
|
||||||
|
def on_train_epoch_end(self):
|
||||||
|
x_train, y_train = self.x_train, self.y_train
|
||||||
|
_components = self.model.components_layer._components.detach()
|
||||||
|
ax = self.fig.gca()
|
||||||
|
ax.cla()
|
||||||
|
ax.set_title(self.title)
|
||||||
|
ax.axis("off")
|
||||||
|
ax.scatter(
|
||||||
|
x_train[:, 0],
|
||||||
|
x_train[:, 1],
|
||||||
|
c=y_train,
|
||||||
|
cmap=self.cmap,
|
||||||
|
edgecolor="k",
|
||||||
|
marker="o",
|
||||||
|
s=30,
|
||||||
|
)
|
||||||
|
ax.scatter(
|
||||||
|
_components[:, 0],
|
||||||
|
_components[:, 1],
|
||||||
|
c="w",
|
||||||
|
cmap=self.cmap,
|
||||||
|
edgecolor="k",
|
||||||
|
marker="D",
|
||||||
|
s=50,
|
||||||
|
)
|
||||||
|
x = torch.vstack((x_train, _components))
|
||||||
|
mesh_input, xx, yy = pt.utils.mesh2d(x, self.border, self.resolution)
|
||||||
|
with torch.no_grad():
|
||||||
|
y_pred = self.model(
|
||||||
|
torch.Tensor(mesh_input).type_as(_components)).argmax(1)
|
||||||
|
y_pred = y_pred.cpu().reshape(xx.shape)
|
||||||
|
ax.contourf(xx, yy, y_pred, cmap=self.cmap, alpha=0.35)
|
||||||
|
plt.pause(0.2)
|
||||||
|
|
||||||
|
|
||||||
|
if __name__ == "__main__":
|
||||||
|
train_ds = pt.datasets.Iris(dims=[0, 2])
|
||||||
|
|
||||||
|
train_loader = torch.utils.data.DataLoader(train_ds, batch_size=32)
|
||||||
|
|
||||||
|
model = CBC(train_ds)
|
||||||
|
|
||||||
|
optimizer = torch.optim.Adam(model.parameters(), lr=0.01)
|
||||||
|
criterion = pt.losses.MarginLoss(margin=0.1)
|
||||||
|
vis = VisCBC2D(model, train_ds)
|
||||||
|
|
||||||
|
for epoch in range(200):
|
||||||
|
correct = 0.0
|
||||||
|
for x, y in train_loader:
|
||||||
|
y_oh = torch.eye(3)[y]
|
||||||
|
y_pred = model(x)
|
||||||
|
loss = criterion(y_pred, y_oh).mean(0)
|
||||||
|
|
||||||
|
optimizer.zero_grad()
|
||||||
|
loss.backward()
|
||||||
|
optimizer.step()
|
||||||
|
|
||||||
|
correct += (y_pred.argmax(1) == y).float().sum(0)
|
||||||
|
|
||||||
|
acc = 100 * correct / len(train_ds)
|
||||||
|
logging.info(f"Epoch: {epoch} Accuracy: {acc:05.02f}%")
|
||||||
|
vis.on_train_epoch_end()
|
@ -1,120 +0,0 @@
|
|||||||
"""ProtoTorch GLVQ example using 2D Iris data."""
|
|
||||||
|
|
||||||
import numpy as np
|
|
||||||
import torch
|
|
||||||
from matplotlib import pyplot as plt
|
|
||||||
from sklearn.datasets import load_iris
|
|
||||||
from sklearn.preprocessing import StandardScaler
|
|
||||||
from torchinfo import summary
|
|
||||||
|
|
||||||
from prototorch.functions.competitions import wtac
|
|
||||||
from prototorch.functions.distances import euclidean_distance
|
|
||||||
from prototorch.modules.losses import GLVQLoss
|
|
||||||
from prototorch.modules.prototypes import Prototypes1D
|
|
||||||
|
|
||||||
# Prepare and preprocess the data
|
|
||||||
scaler = StandardScaler()
|
|
||||||
x_train, y_train = load_iris(return_X_y=True)
|
|
||||||
x_train = x_train[:, [0, 2]]
|
|
||||||
scaler.fit(x_train)
|
|
||||||
x_train = scaler.transform(x_train)
|
|
||||||
|
|
||||||
|
|
||||||
# Define the GLVQ model
|
|
||||||
class Model(torch.nn.Module):
|
|
||||||
def __init__(self):
|
|
||||||
"""GLVQ model for training on 2D Iris data."""
|
|
||||||
super().__init__()
|
|
||||||
self.proto_layer = Prototypes1D(
|
|
||||||
input_dim=2,
|
|
||||||
prototypes_per_class=3,
|
|
||||||
nclasses=3,
|
|
||||||
prototype_initializer="stratified_random",
|
|
||||||
data=[x_train, y_train],
|
|
||||||
)
|
|
||||||
|
|
||||||
def forward(self, x):
|
|
||||||
protos = self.proto_layer.prototypes
|
|
||||||
plabels = self.proto_layer.prototype_labels
|
|
||||||
dis = euclidean_distance(x, protos)
|
|
||||||
return dis, plabels
|
|
||||||
|
|
||||||
|
|
||||||
# Build the GLVQ model
|
|
||||||
model = Model()
|
|
||||||
|
|
||||||
# Print summary using torchinfo (might be buggy/incorrect)
|
|
||||||
print(summary(model))
|
|
||||||
|
|
||||||
# Optimize using SGD optimizer from `torch.optim`
|
|
||||||
optimizer = torch.optim.SGD(model.parameters(), lr=0.01)
|
|
||||||
criterion = GLVQLoss(squashing="sigmoid_beta", beta=10)
|
|
||||||
|
|
||||||
x_in = torch.Tensor(x_train)
|
|
||||||
y_in = torch.Tensor(y_train)
|
|
||||||
|
|
||||||
# Training loop
|
|
||||||
title = "Prototype Visualization"
|
|
||||||
fig = plt.figure(title)
|
|
||||||
for epoch in range(70):
|
|
||||||
# Compute loss
|
|
||||||
dis, plabels = model(x_in)
|
|
||||||
loss = criterion([dis, plabels], y_in)
|
|
||||||
with torch.no_grad():
|
|
||||||
pred = wtac(dis, plabels)
|
|
||||||
correct = pred.eq(y_in.view_as(pred)).sum().item()
|
|
||||||
acc = 100.0 * correct / len(x_train)
|
|
||||||
print(
|
|
||||||
f"Epoch: {epoch + 1:03d} Loss: {loss.item():05.02f} Acc: {acc:05.02f}%"
|
|
||||||
)
|
|
||||||
|
|
||||||
# Take a gradient descent step
|
|
||||||
optimizer.zero_grad()
|
|
||||||
loss.backward()
|
|
||||||
optimizer.step()
|
|
||||||
|
|
||||||
# Get the prototypes form the model
|
|
||||||
protos = model.proto_layer.prototypes.data.numpy()
|
|
||||||
if np.isnan(np.sum(protos)):
|
|
||||||
print("Stopping training because of `nan` in prototypes.")
|
|
||||||
break
|
|
||||||
|
|
||||||
# Visualize the data and the prototypes
|
|
||||||
ax = fig.gca()
|
|
||||||
ax.cla()
|
|
||||||
ax.set_title(title)
|
|
||||||
ax.set_xlabel("Data dimension 1")
|
|
||||||
ax.set_ylabel("Data dimension 2")
|
|
||||||
cmap = "viridis"
|
|
||||||
ax.scatter(x_train[:, 0], x_train[:, 1], c=y_train, edgecolor="k")
|
|
||||||
ax.scatter(
|
|
||||||
protos[:, 0],
|
|
||||||
protos[:, 1],
|
|
||||||
c=plabels,
|
|
||||||
cmap=cmap,
|
|
||||||
edgecolor="k",
|
|
||||||
marker="D",
|
|
||||||
s=50,
|
|
||||||
)
|
|
||||||
|
|
||||||
# Paint decision regions
|
|
||||||
x = np.vstack((x_train, protos))
|
|
||||||
x_min, x_max = x[:, 0].min() - 1, x[:, 0].max() + 1
|
|
||||||
y_min, y_max = x[:, 1].min() - 1, x[:, 1].max() + 1
|
|
||||||
xx, yy = np.meshgrid(np.arange(x_min, x_max, 1 / 50),
|
|
||||||
np.arange(y_min, y_max, 1 / 50))
|
|
||||||
mesh_input = np.c_[xx.ravel(), yy.ravel()]
|
|
||||||
|
|
||||||
torch_input = torch.Tensor(mesh_input)
|
|
||||||
d = model(torch_input)[0]
|
|
||||||
w_indices = torch.argmin(d, dim=1)
|
|
||||||
y_pred = torch.index_select(plabels, 0, w_indices)
|
|
||||||
y_pred = y_pred.reshape(xx.shape)
|
|
||||||
|
|
||||||
# Plot voronoi regions
|
|
||||||
ax.contourf(xx, yy, y_pred, cmap=cmap, alpha=0.35)
|
|
||||||
|
|
||||||
ax.set_xlim(left=x_min + 0, right=x_max - 0)
|
|
||||||
ax.set_ylim(bottom=y_min + 0, top=y_max - 0)
|
|
||||||
|
|
||||||
plt.pause(0.1)
|
|
76
examples/gmlvq.py
Normal file
76
examples/gmlvq.py
Normal file
@ -0,0 +1,76 @@
|
|||||||
|
"""ProtoTorch GMLVQ example using Iris data."""
|
||||||
|
|
||||||
|
import torch
|
||||||
|
|
||||||
|
import prototorch as pt
|
||||||
|
|
||||||
|
|
||||||
|
class GMLVQ(torch.nn.Module):
|
||||||
|
"""
|
||||||
|
Implementation of Generalized Matrix Learning Vector Quantization.
|
||||||
|
"""
|
||||||
|
|
||||||
|
def __init__(self, data, **kwargs):
|
||||||
|
super().__init__(**kwargs)
|
||||||
|
|
||||||
|
self.components_layer = pt.components.LabeledComponents(
|
||||||
|
distribution=[1, 1, 1],
|
||||||
|
components_initializer=pt.initializers.SMCI(data, noise=0.1),
|
||||||
|
)
|
||||||
|
|
||||||
|
self.backbone = pt.transforms.Omega(
|
||||||
|
len(data[0][0]),
|
||||||
|
len(data[0][0]),
|
||||||
|
pt.initializers.RandomLinearTransformInitializer(),
|
||||||
|
)
|
||||||
|
|
||||||
|
def forward(self, data):
|
||||||
|
"""
|
||||||
|
Forward function that returns a tuple of dissimilarities and label information.
|
||||||
|
Feed into GLVQLoss to get a complete GMLVQ model.
|
||||||
|
"""
|
||||||
|
components, label = self.components_layer()
|
||||||
|
|
||||||
|
latent_x = self.backbone(data)
|
||||||
|
latent_components = self.backbone(components)
|
||||||
|
|
||||||
|
distance = pt.distances.squared_euclidean_distance(
|
||||||
|
latent_x, latent_components)
|
||||||
|
|
||||||
|
return distance, label
|
||||||
|
|
||||||
|
def predict(self, data):
|
||||||
|
"""
|
||||||
|
The GMLVQ has a modified prediction step, where a competition layer is applied.
|
||||||
|
"""
|
||||||
|
components, label = self.components_layer()
|
||||||
|
distance = pt.distances.squared_euclidean_distance(data, components)
|
||||||
|
winning_label = pt.competitions.wtac(distance, label)
|
||||||
|
return winning_label
|
||||||
|
|
||||||
|
|
||||||
|
if __name__ == "__main__":
|
||||||
|
train_ds = pt.datasets.Iris()
|
||||||
|
|
||||||
|
train_loader = torch.utils.data.DataLoader(train_ds, batch_size=32)
|
||||||
|
|
||||||
|
model = GMLVQ(train_ds)
|
||||||
|
optimizer = torch.optim.Adam(model.parameters(), lr=0.05)
|
||||||
|
criterion = pt.losses.GLVQLoss()
|
||||||
|
|
||||||
|
for epoch in range(200):
|
||||||
|
correct = 0.0
|
||||||
|
for x, y in train_loader:
|
||||||
|
d, labels = model(x)
|
||||||
|
loss = criterion(d, y, labels).mean(0)
|
||||||
|
|
||||||
|
optimizer.zero_grad()
|
||||||
|
loss.backward()
|
||||||
|
optimizer.step()
|
||||||
|
|
||||||
|
with torch.no_grad():
|
||||||
|
y_pred = model.predict(x)
|
||||||
|
correct += (y_pred == y).float().sum(0)
|
||||||
|
|
||||||
|
acc = 100 * correct / len(train_ds)
|
||||||
|
print(f"Epoch: {epoch} Accuracy: {acc:05.02f}%")
|
@ -1,104 +0,0 @@
|
|||||||
"""ProtoTorch "siamese" GMLVQ example using Tecator."""
|
|
||||||
|
|
||||||
import matplotlib.pyplot as plt
|
|
||||||
import torch
|
|
||||||
from torch.utils.data import DataLoader
|
|
||||||
|
|
||||||
from prototorch.datasets.tecator import Tecator
|
|
||||||
from prototorch.functions.distances import sed
|
|
||||||
from prototorch.modules import Prototypes1D
|
|
||||||
from prototorch.modules.losses import GLVQLoss
|
|
||||||
from prototorch.utils.colors import get_legend_handles
|
|
||||||
|
|
||||||
# Prepare the dataset and dataloader
|
|
||||||
train_data = Tecator(root="./artifacts", train=True)
|
|
||||||
train_loader = DataLoader(train_data, batch_size=128, shuffle=True)
|
|
||||||
|
|
||||||
|
|
||||||
class Model(torch.nn.Module):
|
|
||||||
def __init__(self, **kwargs):
|
|
||||||
"""GMLVQ model as a siamese network."""
|
|
||||||
super().__init__()
|
|
||||||
x, y = train_data.data, train_data.targets
|
|
||||||
self.p1 = Prototypes1D(
|
|
||||||
input_dim=100,
|
|
||||||
prototypes_per_class=2,
|
|
||||||
nclasses=2,
|
|
||||||
prototype_initializer="stratified_random",
|
|
||||||
data=[x, y],
|
|
||||||
)
|
|
||||||
self.omega = torch.nn.Linear(in_features=100,
|
|
||||||
out_features=100,
|
|
||||||
bias=False)
|
|
||||||
torch.nn.init.eye_(self.omega.weight)
|
|
||||||
|
|
||||||
def forward(self, x):
|
|
||||||
protos = self.p1.prototypes
|
|
||||||
plabels = self.p1.prototype_labels
|
|
||||||
|
|
||||||
# Process `x` and `protos` through `omega`
|
|
||||||
x_map = self.omega(x)
|
|
||||||
protos_map = self.omega(protos)
|
|
||||||
|
|
||||||
# Compute distances and output
|
|
||||||
dis = sed(x_map, protos_map)
|
|
||||||
return dis, plabels
|
|
||||||
|
|
||||||
|
|
||||||
# Build the GLVQ model
|
|
||||||
model = Model()
|
|
||||||
|
|
||||||
# Print a summary of the model
|
|
||||||
print(model)
|
|
||||||
|
|
||||||
# Optimize using Adam optimizer from `torch.optim`
|
|
||||||
optimizer = torch.optim.Adam(model.parameters(), lr=0.001_0)
|
|
||||||
scheduler = torch.optim.lr_scheduler.StepLR(optimizer, step_size=75, gamma=0.1)
|
|
||||||
criterion = GLVQLoss(squashing="identity", beta=10)
|
|
||||||
|
|
||||||
# Training loop
|
|
||||||
for epoch in range(150):
|
|
||||||
epoch_loss = 0.0 # zero-out epoch loss
|
|
||||||
optimizer.zero_grad() # zero-out gradients
|
|
||||||
for xb, yb in train_loader:
|
|
||||||
# Compute loss
|
|
||||||
distances, plabels = model(xb)
|
|
||||||
loss = criterion([distances, plabels], yb)
|
|
||||||
epoch_loss += loss.item()
|
|
||||||
# Backprop
|
|
||||||
loss.backward()
|
|
||||||
# Take a gradient descent step
|
|
||||||
optimizer.step()
|
|
||||||
scheduler.step()
|
|
||||||
|
|
||||||
lr = optimizer.param_groups[0]["lr"]
|
|
||||||
print(f"Epoch: {epoch + 1:03d} Loss: {epoch_loss:06.02f} lr: {lr:07.06f}")
|
|
||||||
|
|
||||||
# Get the omega matrix form the model
|
|
||||||
omega = model.omega.weight.data.numpy().T
|
|
||||||
|
|
||||||
# Visualize the lambda matrix
|
|
||||||
title = "Lambda Matrix Visualization"
|
|
||||||
fig = plt.figure(title)
|
|
||||||
ax = fig.gca()
|
|
||||||
ax.set_title(title)
|
|
||||||
im = ax.imshow(omega.dot(omega.T), cmap="viridis")
|
|
||||||
plt.show()
|
|
||||||
|
|
||||||
# Get the prototypes form the model
|
|
||||||
protos = model.p1.prototypes.data.numpy()
|
|
||||||
plabels = model.p1.prototype_labels
|
|
||||||
|
|
||||||
# Visualize the prototypes
|
|
||||||
title = "Tecator Prototypes"
|
|
||||||
fig = plt.figure(title)
|
|
||||||
ax = fig.gca()
|
|
||||||
ax.set_title(title)
|
|
||||||
ax.set_xlabel("Spectral frequencies")
|
|
||||||
ax.set_ylabel("Absorption")
|
|
||||||
clabels = ["Class 0 - Low fat", "Class 1 - High fat"]
|
|
||||||
handles, colors = get_legend_handles(clabels, marker="line", zero_indexed=True)
|
|
||||||
for x, y in zip(protos, plabels):
|
|
||||||
ax.plot(x, c=colors[int(y)])
|
|
||||||
ax.legend(handles, clabels)
|
|
||||||
plt.show()
|
|
@ -1,184 +0,0 @@
|
|||||||
"""
|
|
||||||
ProtoTorch GTLVQ example using MNIST data.
|
|
||||||
The GTLVQ is placed as an classification model on
|
|
||||||
top of a CNN, considered as featurer extractor.
|
|
||||||
Initialization of subpsace and prototypes in
|
|
||||||
Siamnese fashion
|
|
||||||
For more info about GTLVQ see:
|
|
||||||
DOI:10.1109/IJCNN.2016.7727534
|
|
||||||
"""
|
|
||||||
|
|
||||||
import numpy as np
|
|
||||||
import torch
|
|
||||||
import torch.nn as nn
|
|
||||||
import torchvision
|
|
||||||
from torchvision import transforms
|
|
||||||
|
|
||||||
from prototorch.functions.helper import calculate_prototype_accuracy
|
|
||||||
from prototorch.modules.losses import GLVQLoss
|
|
||||||
from prototorch.modules.models import GTLVQ
|
|
||||||
|
|
||||||
# Parameters and options
|
|
||||||
n_epochs = 50
|
|
||||||
batch_size_train = 64
|
|
||||||
batch_size_test = 1000
|
|
||||||
learning_rate = 0.1
|
|
||||||
momentum = 0.5
|
|
||||||
log_interval = 10
|
|
||||||
cuda = "cuda:1"
|
|
||||||
random_seed = 1
|
|
||||||
device = torch.device(cuda if torch.cuda.is_available() else "cpu")
|
|
||||||
|
|
||||||
# Configures reproducability
|
|
||||||
torch.manual_seed(random_seed)
|
|
||||||
np.random.seed(random_seed)
|
|
||||||
|
|
||||||
# Prepare and preprocess the data
|
|
||||||
train_loader = torch.utils.data.DataLoader(
|
|
||||||
torchvision.datasets.MNIST(
|
|
||||||
"./files/",
|
|
||||||
train=True,
|
|
||||||
download=True,
|
|
||||||
transform=torchvision.transforms.Compose([
|
|
||||||
transforms.ToTensor(),
|
|
||||||
transforms.Normalize((0.1307, ), (0.3081, ))
|
|
||||||
]),
|
|
||||||
),
|
|
||||||
batch_size=batch_size_train,
|
|
||||||
shuffle=True,
|
|
||||||
)
|
|
||||||
|
|
||||||
test_loader = torch.utils.data.DataLoader(
|
|
||||||
torchvision.datasets.MNIST(
|
|
||||||
"./files/",
|
|
||||||
train=False,
|
|
||||||
download=True,
|
|
||||||
transform=torchvision.transforms.Compose([
|
|
||||||
transforms.ToTensor(),
|
|
||||||
transforms.Normalize((0.1307, ), (0.3081, ))
|
|
||||||
]),
|
|
||||||
),
|
|
||||||
batch_size=batch_size_test,
|
|
||||||
shuffle=True,
|
|
||||||
)
|
|
||||||
|
|
||||||
|
|
||||||
# Define the GLVQ model plus appropriate feature extractor
|
|
||||||
class CNNGTLVQ(torch.nn.Module):
|
|
||||||
def __init__(
|
|
||||||
self,
|
|
||||||
num_classes,
|
|
||||||
subspace_data,
|
|
||||||
prototype_data,
|
|
||||||
tangent_projection_type="local",
|
|
||||||
prototypes_per_class=2,
|
|
||||||
bottleneck_dim=128,
|
|
||||||
):
|
|
||||||
super(CNNGTLVQ, self).__init__()
|
|
||||||
|
|
||||||
# Feature Extractor - Simple CNN
|
|
||||||
self.fe = nn.Sequential(
|
|
||||||
nn.Conv2d(1, 32, 3, 1),
|
|
||||||
nn.ReLU(),
|
|
||||||
nn.Conv2d(32, 64, 3, 1),
|
|
||||||
nn.ReLU(),
|
|
||||||
nn.MaxPool2d(2),
|
|
||||||
nn.Dropout(0.25),
|
|
||||||
nn.Flatten(),
|
|
||||||
nn.Linear(9216, bottleneck_dim),
|
|
||||||
nn.Dropout(0.5),
|
|
||||||
nn.LeakyReLU(),
|
|
||||||
nn.LayerNorm(bottleneck_dim),
|
|
||||||
)
|
|
||||||
|
|
||||||
# Forward pass of subspace and prototype initialization data through feature extractor
|
|
||||||
subspace_data = self.fe(subspace_data)
|
|
||||||
prototype_data[0] = self.fe(prototype_data[0])
|
|
||||||
|
|
||||||
# Initialization of GTLVQ
|
|
||||||
self.gtlvq = GTLVQ(
|
|
||||||
num_classes,
|
|
||||||
subspace_data,
|
|
||||||
prototype_data,
|
|
||||||
tangent_projection_type=tangent_projection_type,
|
|
||||||
feature_dim=bottleneck_dim,
|
|
||||||
prototypes_per_class=prototypes_per_class,
|
|
||||||
)
|
|
||||||
|
|
||||||
def forward(self, x):
|
|
||||||
# Feature Extraction
|
|
||||||
x = self.fe(x)
|
|
||||||
|
|
||||||
# GTLVQ Forward pass
|
|
||||||
dis = self.gtlvq(x)
|
|
||||||
return dis
|
|
||||||
|
|
||||||
|
|
||||||
# Get init data
|
|
||||||
subspace_data = torch.cat(
|
|
||||||
[next(iter(train_loader))[0],
|
|
||||||
next(iter(test_loader))[0]])
|
|
||||||
prototype_data = next(iter(train_loader))
|
|
||||||
|
|
||||||
# Build the CNN GTLVQ model
|
|
||||||
model = CNNGTLVQ(
|
|
||||||
10,
|
|
||||||
subspace_data,
|
|
||||||
prototype_data,
|
|
||||||
tangent_projection_type="local",
|
|
||||||
bottleneck_dim=128,
|
|
||||||
).to(device)
|
|
||||||
|
|
||||||
# Optimize using SGD optimizer from `torch.optim`
|
|
||||||
optimizer = torch.optim.Adam(
|
|
||||||
[{
|
|
||||||
"params": model.fe.parameters()
|
|
||||||
}, {
|
|
||||||
"params": model.gtlvq.parameters()
|
|
||||||
}],
|
|
||||||
lr=learning_rate,
|
|
||||||
)
|
|
||||||
criterion = GLVQLoss(squashing="sigmoid_beta", beta=10)
|
|
||||||
|
|
||||||
# Training loop
|
|
||||||
for epoch in range(n_epochs):
|
|
||||||
for batch_idx, (x_train, y_train) in enumerate(train_loader):
|
|
||||||
model.train()
|
|
||||||
x_train, y_train = x_train.to(device), y_train.to(device)
|
|
||||||
optimizer.zero_grad()
|
|
||||||
|
|
||||||
distances = model(x_train)
|
|
||||||
plabels = model.gtlvq.cls.prototype_labels.to(device)
|
|
||||||
|
|
||||||
# Compute loss.
|
|
||||||
loss = criterion([distances, plabels], y_train)
|
|
||||||
loss.backward()
|
|
||||||
optimizer.step()
|
|
||||||
|
|
||||||
# GTLVQ uses projected SGD, which means to orthogonalize the subspaces after every gradient update.
|
|
||||||
model.gtlvq.orthogonalize_subspace()
|
|
||||||
|
|
||||||
if batch_idx % log_interval == 0:
|
|
||||||
acc = calculate_prototype_accuracy(distances, y_train, plabels)
|
|
||||||
print(
|
|
||||||
f"Epoch: {epoch + 1:02d}/{n_epochs:02d} Epoch Progress: {100. * batch_idx / len(train_loader):02.02f} % Loss: {loss.item():02.02f} \
|
|
||||||
Train Acc: {acc.item():02.02f}")
|
|
||||||
|
|
||||||
# Test
|
|
||||||
with torch.no_grad():
|
|
||||||
model.eval()
|
|
||||||
correct = 0
|
|
||||||
total = 0
|
|
||||||
for x_test, y_test in test_loader:
|
|
||||||
x_test, y_test = x_test.to(device), y_test.to(device)
|
|
||||||
test_distances = model(torch.tensor(x_test))
|
|
||||||
test_plabels = model.gtlvq.cls.prototype_labels.to(device)
|
|
||||||
i = torch.argmin(test_distances, 1)
|
|
||||||
correct += torch.sum(y_test == test_plabels[i])
|
|
||||||
total += y_test.size(0)
|
|
||||||
print("Accuracy of the network on the test images: %d %%" %
|
|
||||||
(torch.true_divide(correct, total) * 100))
|
|
||||||
|
|
||||||
# Save the model
|
|
||||||
PATH = "./glvq_mnist_model.pth"
|
|
||||||
torch.save(model.state_dict(), PATH)
|
|
@ -1,110 +0,0 @@
|
|||||||
"""ProtoTorch LGMLVQ example using 2D Iris data."""
|
|
||||||
|
|
||||||
import numpy as np
|
|
||||||
import torch
|
|
||||||
from matplotlib import pyplot as plt
|
|
||||||
from sklearn.datasets import load_iris
|
|
||||||
from sklearn.metrics import accuracy_score
|
|
||||||
|
|
||||||
from prototorch.functions.competitions import stratified_min
|
|
||||||
from prototorch.functions.distances import lomega_distance
|
|
||||||
from prototorch.functions.init import eye_
|
|
||||||
from prototorch.modules.losses import GLVQLoss
|
|
||||||
from prototorch.modules.prototypes import Prototypes1D
|
|
||||||
|
|
||||||
# Prepare training data
|
|
||||||
x_train, y_train = load_iris(True)
|
|
||||||
x_train = x_train[:, [0, 2]]
|
|
||||||
|
|
||||||
|
|
||||||
# Define the model
|
|
||||||
class Model(torch.nn.Module):
|
|
||||||
def __init__(self):
|
|
||||||
"""Local-GMLVQ model."""
|
|
||||||
super().__init__()
|
|
||||||
self.p1 = Prototypes1D(
|
|
||||||
input_dim=2,
|
|
||||||
prototype_distribution=[1, 2, 2],
|
|
||||||
prototype_initializer="stratified_random",
|
|
||||||
data=[x_train, y_train],
|
|
||||||
)
|
|
||||||
omegas = torch.zeros(5, 2, 2)
|
|
||||||
self.omegas = torch.nn.Parameter(omegas)
|
|
||||||
eye_(self.omegas)
|
|
||||||
|
|
||||||
def forward(self, x):
|
|
||||||
protos = self.p1.prototypes
|
|
||||||
plabels = self.p1.prototype_labels
|
|
||||||
omegas = self.omegas
|
|
||||||
dis = lomega_distance(x, protos, omegas)
|
|
||||||
return dis, plabels
|
|
||||||
|
|
||||||
|
|
||||||
# Build the model
|
|
||||||
model = Model()
|
|
||||||
|
|
||||||
# Optimize using Adam optimizer from `torch.optim`
|
|
||||||
optimizer = torch.optim.Adam(model.parameters(), lr=0.01)
|
|
||||||
criterion = GLVQLoss(squashing="sigmoid_beta", beta=10)
|
|
||||||
|
|
||||||
x_in = torch.Tensor(x_train)
|
|
||||||
y_in = torch.Tensor(y_train)
|
|
||||||
|
|
||||||
# Training loop
|
|
||||||
title = "Prototype Visualization"
|
|
||||||
fig = plt.figure(title)
|
|
||||||
for epoch in range(100):
|
|
||||||
# Compute loss
|
|
||||||
dis, plabels = model(x_in)
|
|
||||||
loss = criterion([dis, plabels], y_in)
|
|
||||||
y_pred = np.argmin(stratified_min(dis, plabels).detach().numpy(), axis=1)
|
|
||||||
acc = accuracy_score(y_train, y_pred)
|
|
||||||
log_string = f"Epoch: {epoch + 1:03d} Loss: {loss.item():05.02f} "
|
|
||||||
log_string += f"Acc: {acc * 100:05.02f}%"
|
|
||||||
print(log_string)
|
|
||||||
|
|
||||||
# Take a gradient descent step
|
|
||||||
optimizer.zero_grad()
|
|
||||||
loss.backward()
|
|
||||||
optimizer.step()
|
|
||||||
|
|
||||||
# Get the prototypes form the model
|
|
||||||
protos = model.p1.prototypes.data.numpy()
|
|
||||||
|
|
||||||
# Visualize the data and the prototypes
|
|
||||||
ax = fig.gca()
|
|
||||||
ax.cla()
|
|
||||||
ax.set_title(title)
|
|
||||||
ax.set_xlabel("Data dimension 1")
|
|
||||||
ax.set_ylabel("Data dimension 2")
|
|
||||||
cmap = "viridis"
|
|
||||||
ax.scatter(x_train[:, 0], x_train[:, 1], c=y_train, edgecolor="k")
|
|
||||||
ax.scatter(
|
|
||||||
protos[:, 0],
|
|
||||||
protos[:, 1],
|
|
||||||
c=plabels,
|
|
||||||
cmap=cmap,
|
|
||||||
edgecolor="k",
|
|
||||||
marker="D",
|
|
||||||
s=50,
|
|
||||||
)
|
|
||||||
|
|
||||||
# Paint decision regions
|
|
||||||
x = np.vstack((x_train, protos))
|
|
||||||
x_min, x_max = x[:, 0].min() - 1, x[:, 0].max() + 1
|
|
||||||
y_min, y_max = x[:, 1].min() - 1, x[:, 1].max() + 1
|
|
||||||
xx, yy = np.meshgrid(np.arange(x_min, x_max, 1 / 50),
|
|
||||||
np.arange(y_min, y_max, 1 / 50))
|
|
||||||
mesh_input = np.c_[xx.ravel(), yy.ravel()]
|
|
||||||
|
|
||||||
d, plabels = model(torch.Tensor(mesh_input))
|
|
||||||
y_pred = np.argmin(stratified_min(d, plabels).detach().numpy(), axis=1)
|
|
||||||
y_pred = y_pred.reshape(xx.shape)
|
|
||||||
|
|
||||||
# Plot voronoi regions
|
|
||||||
ax.contourf(xx, yy, y_pred, cmap=cmap, alpha=0.35)
|
|
||||||
|
|
||||||
ax.set_xlim(left=x_min + 0, right=x_max - 0)
|
|
||||||
ax.set_ylim(bottom=y_min + 0, top=y_max - 0)
|
|
||||||
|
|
||||||
plt.pause(0.1)
|
|
@ -1,39 +1,35 @@
|
|||||||
"""This example script shows the usage of the new components architecture.
|
"""This example script shows the usage of the new components architecture.
|
||||||
|
|
||||||
Serialization/deserialization also works as expected.
|
Serialization/deserialization also works as expected.
|
||||||
|
|
||||||
"""
|
"""
|
||||||
|
|
||||||
# DATASET
|
|
||||||
import torch
|
import torch
|
||||||
from sklearn.datasets import load_iris
|
|
||||||
from sklearn.preprocessing import StandardScaler
|
|
||||||
|
|
||||||
scaler = StandardScaler()
|
import prototorch as pt
|
||||||
x_train, y_train = load_iris(return_X_y=True)
|
|
||||||
x_train = x_train[:, [0, 2]]
|
|
||||||
scaler.fit(x_train)
|
|
||||||
x_train = scaler.transform(x_train)
|
|
||||||
|
|
||||||
x_train = torch.Tensor(x_train)
|
ds = pt.datasets.Iris()
|
||||||
y_train = torch.Tensor(y_train)
|
|
||||||
num_classes = len(torch.unique(y_train))
|
|
||||||
|
|
||||||
# CREATE NEW COMPONENTS
|
unsupervised = pt.components.Components(
|
||||||
from prototorch.components import *
|
6,
|
||||||
from prototorch.components.initializers import *
|
initializer=pt.initializers.ZCI(2),
|
||||||
|
)
|
||||||
unsupervised = Components(6, SelectionInitializer(x_train))
|
|
||||||
print(unsupervised())
|
print(unsupervised())
|
||||||
|
|
||||||
prototypes = LabeledComponents(
|
prototypes = pt.components.LabeledComponents(
|
||||||
(3, 2), StratifiedSelectionInitializer(x_train, y_train))
|
(3, 2),
|
||||||
|
components_initializer=pt.initializers.SSCI(ds),
|
||||||
|
)
|
||||||
print(prototypes())
|
print(prototypes())
|
||||||
|
|
||||||
components = ReasoningComponents(
|
components = pt.components.ReasoningComponents(
|
||||||
(3, 6), StratifiedSelectionInitializer(x_train, y_train))
|
(3, 2),
|
||||||
print(components())
|
components_initializer=pt.initializers.SSCI(ds),
|
||||||
|
reasonings_initializer=pt.initializers.PPRI(),
|
||||||
|
)
|
||||||
|
print(prototypes())
|
||||||
|
|
||||||
# TEST SERIALIZATION
|
# Test Serialization
|
||||||
import io
|
import io
|
||||||
|
|
||||||
save = io.BytesIO()
|
save = io.BytesIO()
|
||||||
@ -41,25 +37,20 @@ torch.save(unsupervised, save)
|
|||||||
save.seek(0)
|
save.seek(0)
|
||||||
serialized_unsupervised = torch.load(save)
|
serialized_unsupervised = torch.load(save)
|
||||||
|
|
||||||
assert torch.all(unsupervised.components == serialized_unsupervised.components
|
assert torch.all(unsupervised.components == serialized_unsupervised.components)
|
||||||
), "Serialization of Components failed."
|
|
||||||
|
|
||||||
save = io.BytesIO()
|
save = io.BytesIO()
|
||||||
torch.save(prototypes, save)
|
torch.save(prototypes, save)
|
||||||
save.seek(0)
|
save.seek(0)
|
||||||
serialized_prototypes = torch.load(save)
|
serialized_prototypes = torch.load(save)
|
||||||
|
|
||||||
assert torch.all(prototypes.components == serialized_prototypes.components
|
assert torch.all(prototypes.components == serialized_prototypes.components)
|
||||||
), "Serialization of Components failed."
|
assert torch.all(prototypes.labels == serialized_prototypes.labels)
|
||||||
assert torch.all(prototypes.component_labels == serialized_prototypes.
|
|
||||||
component_labels), "Serialization of Components failed."
|
|
||||||
|
|
||||||
save = io.BytesIO()
|
save = io.BytesIO()
|
||||||
torch.save(components, save)
|
torch.save(components, save)
|
||||||
save.seek(0)
|
save.seek(0)
|
||||||
serialized_components = torch.load(save)
|
serialized_components = torch.load(save)
|
||||||
|
|
||||||
assert torch.all(components.components == serialized_components.components
|
assert torch.all(components.components == serialized_components.components)
|
||||||
), "Serialization of Components failed."
|
assert torch.all(components.reasonings == serialized_components.reasonings)
|
||||||
assert torch.all(components.reasonings == serialized_components.reasonings
|
|
||||||
), "Serialization of Components failed."
|
|
||||||
|
@ -1,21 +1,40 @@
|
|||||||
"""ProtoTorch package."""
|
"""ProtoTorch package"""
|
||||||
|
|
||||||
# Core Setup
|
|
||||||
__version__ = "0.4.2"
|
|
||||||
|
|
||||||
__all_core__ = [
|
|
||||||
"datasets",
|
|
||||||
"functions",
|
|
||||||
"modules",
|
|
||||||
]
|
|
||||||
|
|
||||||
from .datasets import *
|
|
||||||
|
|
||||||
# Plugin Loader
|
|
||||||
import pkgutil
|
import pkgutil
|
||||||
|
|
||||||
import pkg_resources
|
import pkg_resources
|
||||||
|
|
||||||
|
from . import datasets # noqa: F401
|
||||||
|
from . import nn # noqa: F401
|
||||||
|
from . import utils # noqa: F401
|
||||||
|
from .core import competitions # noqa: F401
|
||||||
|
from .core import components # noqa: F401
|
||||||
|
from .core import distances # noqa: F401
|
||||||
|
from .core import initializers # noqa: F401
|
||||||
|
from .core import losses # noqa: F401
|
||||||
|
from .core import pooling # noqa: F401
|
||||||
|
from .core import similarities # noqa: F401
|
||||||
|
from .core import transforms # noqa: F401
|
||||||
|
|
||||||
|
# Core Setup
|
||||||
|
__version__ = "0.7.6"
|
||||||
|
|
||||||
|
__all_core__ = [
|
||||||
|
"competitions",
|
||||||
|
"components",
|
||||||
|
"core",
|
||||||
|
"datasets",
|
||||||
|
"distances",
|
||||||
|
"initializers",
|
||||||
|
"losses",
|
||||||
|
"nn",
|
||||||
|
"pooling",
|
||||||
|
"similarities",
|
||||||
|
"transforms",
|
||||||
|
"utils",
|
||||||
|
]
|
||||||
|
|
||||||
|
# Plugin Loader
|
||||||
__path__ = pkgutil.extend_path(__path__, __name__)
|
__path__ = pkgutil.extend_path(__path__, __name__)
|
||||||
|
|
||||||
|
|
||||||
|
@ -1,2 +0,0 @@
|
|||||||
from prototorch.components.components import *
|
|
||||||
from prototorch.components.initializers import *
|
|
@ -1,151 +0,0 @@
|
|||||||
"""ProtoTorch components modules."""
|
|
||||||
|
|
||||||
import warnings
|
|
||||||
from typing import Tuple
|
|
||||||
|
|
||||||
import torch
|
|
||||||
from prototorch.components.initializers import (ClassAwareInitializer,
|
|
||||||
ComponentsInitializer,
|
|
||||||
EqualLabelsInitializer,
|
|
||||||
UnequalLabelsInitializer,
|
|
||||||
ZeroReasoningsInitializer)
|
|
||||||
from prototorch.functions.initializers import get_initializer
|
|
||||||
from torch.nn.parameter import Parameter
|
|
||||||
|
|
||||||
|
|
||||||
class Components(torch.nn.Module):
|
|
||||||
"""Components is a set of learnable Tensors."""
|
|
||||||
def __init__(self,
|
|
||||||
number_of_components=None,
|
|
||||||
initializer=None,
|
|
||||||
*,
|
|
||||||
initialized_components=None,
|
|
||||||
dtype=torch.float32):
|
|
||||||
super().__init__()
|
|
||||||
|
|
||||||
# Ignore all initialization settings if initialized_components is given.
|
|
||||||
if initialized_components is not None:
|
|
||||||
self._components = Parameter(initialized_components)
|
|
||||||
if number_of_components is not None or initializer is not None:
|
|
||||||
wmsg = "Arguments ignored while initializing Components"
|
|
||||||
warnings.warn(wmsg)
|
|
||||||
else:
|
|
||||||
self._initialize_components(number_of_components, initializer)
|
|
||||||
|
|
||||||
def _precheck_initializer(self, initializer):
|
|
||||||
if not isinstance(initializer, ComponentsInitializer):
|
|
||||||
emsg = f"`initializer` has to be some subtype of " \
|
|
||||||
f"{ComponentsInitializer}. " \
|
|
||||||
f"You have provided: {initializer=} instead."
|
|
||||||
raise TypeError(emsg)
|
|
||||||
|
|
||||||
def _initialize_components(self, number_of_components, initializer):
|
|
||||||
self._precheck_initializer(initializer)
|
|
||||||
self._components = Parameter(
|
|
||||||
initializer.generate(number_of_components))
|
|
||||||
|
|
||||||
@property
|
|
||||||
def components(self):
|
|
||||||
"""Tensor containing the component tensors."""
|
|
||||||
return self._components.detach().cpu()
|
|
||||||
|
|
||||||
def forward(self):
|
|
||||||
return self._components
|
|
||||||
|
|
||||||
def extra_repr(self):
|
|
||||||
return f"components.shape: {tuple(self._components.shape)}"
|
|
||||||
|
|
||||||
|
|
||||||
class LabeledComponents(Components):
|
|
||||||
"""LabeledComponents generate a set of components and a set of labels.
|
|
||||||
|
|
||||||
Every Component has a label assigned.
|
|
||||||
"""
|
|
||||||
def __init__(self,
|
|
||||||
distribution=None,
|
|
||||||
initializer=None,
|
|
||||||
*,
|
|
||||||
initialized_components=None):
|
|
||||||
if initialized_components is not None:
|
|
||||||
super().__init__(initialized_components=initialized_components[0])
|
|
||||||
self._labels = initialized_components[1]
|
|
||||||
else:
|
|
||||||
self._initialize_labels(distribution)
|
|
||||||
super().__init__(number_of_components=len(self._labels),
|
|
||||||
initializer=initializer)
|
|
||||||
|
|
||||||
def _initialize_components(self, number_of_components, initializer):
|
|
||||||
if isinstance(initializer, ClassAwareInitializer):
|
|
||||||
self._precheck_initializer(initializer)
|
|
||||||
self._components = Parameter(
|
|
||||||
initializer.generate(number_of_components, self.distribution))
|
|
||||||
else:
|
|
||||||
super()._initialize_components(self, number_of_components,
|
|
||||||
initializer)
|
|
||||||
|
|
||||||
def _initialize_labels(self, distribution):
|
|
||||||
if type(distribution) == tuple:
|
|
||||||
num_classes, prototypes_per_class = distribution
|
|
||||||
labels = EqualLabelsInitializer(num_classes, prototypes_per_class)
|
|
||||||
elif type(distribution) == list:
|
|
||||||
labels = UnequalLabelsInitializer(distribution)
|
|
||||||
|
|
||||||
self.distribution = labels.distribution
|
|
||||||
self._labels = labels.generate()
|
|
||||||
|
|
||||||
@property
|
|
||||||
def component_labels(self):
|
|
||||||
"""Tensor containing the component tensors."""
|
|
||||||
return self._labels.detach().cpu()
|
|
||||||
|
|
||||||
def forward(self):
|
|
||||||
return super().forward(), self._labels
|
|
||||||
|
|
||||||
|
|
||||||
class ReasoningComponents(Components):
|
|
||||||
"""ReasoningComponents generate a set of components and a set of reasoning matrices.
|
|
||||||
|
|
||||||
Every Component has a reasoning matrix assigned.
|
|
||||||
|
|
||||||
A reasoning matrix is a Nx2 matrix, where N is the number of Classes. The
|
|
||||||
first element is called positive reasoning :math:`p`, the second negative
|
|
||||||
reasoning :math:`n`. A components can reason in favour (positive) of a
|
|
||||||
class, against (negative) a class or not at all (neutral).
|
|
||||||
|
|
||||||
It holds that :math:`0 \leq n \leq 1`, :math:`0 \leq p \leq 1` and :math:`0
|
|
||||||
\leq n+p \leq 1`. Therefore :math:`n` and :math:`p` are two elements of a
|
|
||||||
three element probability distribution.
|
|
||||||
|
|
||||||
"""
|
|
||||||
def __init__(self,
|
|
||||||
reasonings=None,
|
|
||||||
initializer=None,
|
|
||||||
*,
|
|
||||||
initialized_components=None):
|
|
||||||
if initialized_components is not None:
|
|
||||||
super().__init__(initialized_components=initialized_components[0])
|
|
||||||
self._reasonings = initialized_components[1]
|
|
||||||
else:
|
|
||||||
self._initialize_reasonings(reasonings)
|
|
||||||
super().__init__(number_of_components=len(self._reasonings),
|
|
||||||
initializer=initializer)
|
|
||||||
|
|
||||||
def _initialize_reasonings(self, reasonings):
|
|
||||||
if type(reasonings) == tuple:
|
|
||||||
num_classes, number_of_components = reasonings
|
|
||||||
reasonings = ZeroReasoningsInitializer(num_classes,
|
|
||||||
number_of_components)
|
|
||||||
|
|
||||||
self._reasonings = reasonings.generate()
|
|
||||||
|
|
||||||
@property
|
|
||||||
def reasonings(self):
|
|
||||||
"""Returns Reasoning Matrix.
|
|
||||||
|
|
||||||
Dimension NxCx2
|
|
||||||
|
|
||||||
"""
|
|
||||||
return self._reasonings.detach().cpu()
|
|
||||||
|
|
||||||
def forward(self):
|
|
||||||
return super().forward(), self._reasonings
|
|
@ -1,197 +0,0 @@
|
|||||||
"""ProtoTroch Initializers."""
|
|
||||||
import warnings
|
|
||||||
from collections.abc import Iterable
|
|
||||||
from itertools import chain
|
|
||||||
|
|
||||||
import torch
|
|
||||||
from torch.utils.data import DataLoader, Dataset
|
|
||||||
|
|
||||||
|
|
||||||
def parse_init_arg(arg):
|
|
||||||
if isinstance(arg, Dataset):
|
|
||||||
data, labels = next(iter(DataLoader(arg, batch_size=len(arg))))
|
|
||||||
# data = data.view(len(arg), -1) # flatten
|
|
||||||
else:
|
|
||||||
data, labels = arg
|
|
||||||
if not isinstance(data, torch.Tensor):
|
|
||||||
wmsg = f"Converting data to {torch.Tensor}."
|
|
||||||
warnings.warn(wmsg)
|
|
||||||
data = torch.Tensor(data)
|
|
||||||
if not isinstance(labels, torch.Tensor):
|
|
||||||
wmsg = f"Converting labels to {torch.Tensor}."
|
|
||||||
warnings.warn(wmsg)
|
|
||||||
labels = torch.Tensor(labels)
|
|
||||||
return data, labels
|
|
||||||
|
|
||||||
|
|
||||||
# Components
|
|
||||||
class ComponentsInitializer(object):
|
|
||||||
def generate(self, number_of_components):
|
|
||||||
raise NotImplementedError("Subclasses should implement this!")
|
|
||||||
|
|
||||||
|
|
||||||
class DimensionAwareInitializer(ComponentsInitializer):
|
|
||||||
def __init__(self, c_dims):
|
|
||||||
super().__init__()
|
|
||||||
if isinstance(c_dims, Iterable):
|
|
||||||
self.components_dims = tuple(c_dims)
|
|
||||||
else:
|
|
||||||
self.components_dims = (c_dims, )
|
|
||||||
|
|
||||||
|
|
||||||
class OnesInitializer(DimensionAwareInitializer):
|
|
||||||
def generate(self, length):
|
|
||||||
gen_dims = (length, ) + self.components_dims
|
|
||||||
return torch.ones(gen_dims)
|
|
||||||
|
|
||||||
|
|
||||||
class ZerosInitializer(DimensionAwareInitializer):
|
|
||||||
def generate(self, length):
|
|
||||||
gen_dims = (length, ) + self.components_dims
|
|
||||||
return torch.zeros(gen_dims)
|
|
||||||
|
|
||||||
|
|
||||||
class UniformInitializer(DimensionAwareInitializer):
|
|
||||||
def __init__(self, c_dims, min=0.0, max=1.0):
|
|
||||||
super().__init__(c_dims)
|
|
||||||
|
|
||||||
self.min = min
|
|
||||||
self.max = max
|
|
||||||
|
|
||||||
def generate(self, length):
|
|
||||||
gen_dims = (length, ) + self.components_dims
|
|
||||||
return torch.ones(gen_dims).uniform_(self.min, self.max)
|
|
||||||
|
|
||||||
|
|
||||||
class PositionAwareInitializer(ComponentsInitializer):
|
|
||||||
def __init__(self, positions):
|
|
||||||
super().__init__()
|
|
||||||
self.data = positions
|
|
||||||
|
|
||||||
|
|
||||||
class SelectionInitializer(PositionAwareInitializer):
|
|
||||||
def generate(self, length):
|
|
||||||
indices = torch.LongTensor(length).random_(0, len(self.data))
|
|
||||||
return self.data[indices]
|
|
||||||
|
|
||||||
|
|
||||||
class MeanInitializer(PositionAwareInitializer):
|
|
||||||
def generate(self, length):
|
|
||||||
mean = torch.mean(self.data, dim=0)
|
|
||||||
repeat_dim = [length] + [1] * len(mean.shape)
|
|
||||||
return mean.repeat(repeat_dim)
|
|
||||||
|
|
||||||
|
|
||||||
class ClassAwareInitializer(ComponentsInitializer):
|
|
||||||
def __init__(self, arg):
|
|
||||||
super().__init__()
|
|
||||||
data, labels = parse_init_arg(arg)
|
|
||||||
self.data = data
|
|
||||||
self.labels = labels
|
|
||||||
|
|
||||||
self.clabels = torch.unique(self.labels)
|
|
||||||
self.num_classes = len(self.clabels)
|
|
||||||
|
|
||||||
def _get_samples_from_initializer(self, length, dist):
|
|
||||||
if not dist:
|
|
||||||
per_class = length // self.num_classes
|
|
||||||
dist = self.num_classes * [per_class]
|
|
||||||
samples_list = [
|
|
||||||
init.generate(n) for init, n in zip(self.initializers, dist)
|
|
||||||
]
|
|
||||||
return torch.vstack(samples_list)
|
|
||||||
|
|
||||||
|
|
||||||
class StratifiedMeanInitializer(ClassAwareInitializer):
|
|
||||||
def __init__(self, arg):
|
|
||||||
super().__init__(arg)
|
|
||||||
|
|
||||||
self.initializers = []
|
|
||||||
for clabel in self.clabels:
|
|
||||||
class_data = self.data[self.labels == clabel]
|
|
||||||
class_initializer = MeanInitializer(class_data)
|
|
||||||
self.initializers.append(class_initializer)
|
|
||||||
|
|
||||||
def generate(self, length, dist=[]):
|
|
||||||
samples = self._get_samples_from_initializer(length, dist)
|
|
||||||
return samples
|
|
||||||
|
|
||||||
|
|
||||||
class StratifiedSelectionInitializer(ClassAwareInitializer):
|
|
||||||
def __init__(self, arg, *, noise=None):
|
|
||||||
super().__init__(arg)
|
|
||||||
self.noise = noise
|
|
||||||
|
|
||||||
self.initializers = []
|
|
||||||
for clabel in self.clabels:
|
|
||||||
class_data = self.data[self.labels == clabel]
|
|
||||||
class_initializer = SelectionInitializer(class_data)
|
|
||||||
self.initializers.append(class_initializer)
|
|
||||||
|
|
||||||
def add_noise(self, x):
|
|
||||||
"""Shifts some dimensions of the data randomly."""
|
|
||||||
n1 = torch.rand_like(x)
|
|
||||||
n2 = torch.rand_like(x)
|
|
||||||
mask = torch.bernoulli(n1) - torch.bernoulli(n2)
|
|
||||||
return x + (self.noise * mask)
|
|
||||||
|
|
||||||
def generate(self, length, dist=[]):
|
|
||||||
samples = self._get_samples_from_initializer(length, dist)
|
|
||||||
if self.noise is not None:
|
|
||||||
# samples = self.add_noise(samples)
|
|
||||||
samples = samples + self.noise
|
|
||||||
return samples
|
|
||||||
|
|
||||||
|
|
||||||
# Labels
|
|
||||||
class LabelsInitializer:
|
|
||||||
def generate(self):
|
|
||||||
raise NotImplementedError("Subclasses should implement this!")
|
|
||||||
|
|
||||||
|
|
||||||
class UnequalLabelsInitializer(LabelsInitializer):
|
|
||||||
def __init__(self, dist):
|
|
||||||
self.dist = dist
|
|
||||||
|
|
||||||
@property
|
|
||||||
def distribution(self):
|
|
||||||
return self.dist
|
|
||||||
|
|
||||||
def generate(self):
|
|
||||||
clabels = range(len(self.dist))
|
|
||||||
labels = list(chain(*[[i] * n for i, n in zip(clabels, self.dist)]))
|
|
||||||
return torch.tensor(labels)
|
|
||||||
|
|
||||||
|
|
||||||
class EqualLabelsInitializer(LabelsInitializer):
|
|
||||||
def __init__(self, classes, per_class):
|
|
||||||
self.classes = classes
|
|
||||||
self.per_class = per_class
|
|
||||||
|
|
||||||
@property
|
|
||||||
def distribution(self):
|
|
||||||
return self.classes * [self.per_class]
|
|
||||||
|
|
||||||
def generate(self):
|
|
||||||
return torch.arange(self.classes).repeat(self.per_class, 1).T.flatten()
|
|
||||||
|
|
||||||
|
|
||||||
# Reasonings
|
|
||||||
class ReasoningsInitializer:
|
|
||||||
def generate(self, length):
|
|
||||||
raise NotImplementedError("Subclasses should implement this!")
|
|
||||||
|
|
||||||
|
|
||||||
class ZeroReasoningsInitializer(ReasoningsInitializer):
|
|
||||||
def __init__(self, classes, length):
|
|
||||||
self.classes = classes
|
|
||||||
self.length = length
|
|
||||||
|
|
||||||
def generate(self):
|
|
||||||
return torch.zeros((self.length, self.classes, 2))
|
|
||||||
|
|
||||||
|
|
||||||
# Aliases
|
|
||||||
SSI = StratifiedSampleInitializer = StratifiedSelectionInitializer
|
|
||||||
SMI = StratifiedMeanInitializer
|
|
||||||
Random = RandomInitializer = UniformInitializer
|
|
10
prototorch/core/__init__.py
Normal file
10
prototorch/core/__init__.py
Normal file
@ -0,0 +1,10 @@
|
|||||||
|
"""ProtoTorch core"""
|
||||||
|
|
||||||
|
from .competitions import *
|
||||||
|
from .components import *
|
||||||
|
from .distances import *
|
||||||
|
from .initializers import *
|
||||||
|
from .losses import *
|
||||||
|
from .pooling import *
|
||||||
|
from .similarities import *
|
||||||
|
from .transforms import *
|
93
prototorch/core/competitions.py
Normal file
93
prototorch/core/competitions.py
Normal file
@ -0,0 +1,93 @@
|
|||||||
|
"""ProtoTorch competitions"""
|
||||||
|
|
||||||
|
import torch
|
||||||
|
|
||||||
|
|
||||||
|
def wtac(distances: torch.Tensor, labels: torch.LongTensor):
|
||||||
|
"""Winner-Takes-All-Competition.
|
||||||
|
|
||||||
|
Returns the labels corresponding to the winners.
|
||||||
|
|
||||||
|
"""
|
||||||
|
winning_indices = torch.min(distances, dim=1).indices
|
||||||
|
winning_labels = labels[winning_indices].squeeze()
|
||||||
|
return winning_labels
|
||||||
|
|
||||||
|
|
||||||
|
def knnc(distances: torch.Tensor, labels: torch.LongTensor, k: int = 1):
|
||||||
|
"""K-Nearest-Neighbors-Competition.
|
||||||
|
|
||||||
|
Returns the labels corresponding to the winners.
|
||||||
|
|
||||||
|
"""
|
||||||
|
winning_indices = torch.topk(-distances, k=k, dim=1).indices
|
||||||
|
winning_labels = torch.mode(labels[winning_indices], dim=1).values
|
||||||
|
return winning_labels
|
||||||
|
|
||||||
|
|
||||||
|
def cbcc(detections: torch.Tensor, reasonings: torch.Tensor):
|
||||||
|
"""Classification-By-Components Competition.
|
||||||
|
|
||||||
|
Returns probability distributions over the classes.
|
||||||
|
|
||||||
|
`detections` must be of shape [batch_size, num_components].
|
||||||
|
`reasonings` must be of shape [num_components, num_classes, 2].
|
||||||
|
|
||||||
|
"""
|
||||||
|
A, B = reasonings.permute(2, 1, 0).clamp(0, 1)
|
||||||
|
pk = A
|
||||||
|
nk = (1 - A) * B
|
||||||
|
numerator = (detections @ (pk - nk).T) + nk.sum(1)
|
||||||
|
probs = numerator / ((pk + nk).sum(1) + 1e-8)
|
||||||
|
return probs
|
||||||
|
|
||||||
|
|
||||||
|
class WTAC(torch.nn.Module):
|
||||||
|
"""Winner-Takes-All-Competition Layer.
|
||||||
|
|
||||||
|
Thin wrapper over the `wtac` function.
|
||||||
|
|
||||||
|
"""
|
||||||
|
|
||||||
|
def forward(self, distances, labels): # pylint: disable=no-self-use
|
||||||
|
return wtac(distances, labels)
|
||||||
|
|
||||||
|
|
||||||
|
class LTAC(torch.nn.Module):
|
||||||
|
"""Loser-Takes-All-Competition Layer.
|
||||||
|
|
||||||
|
Thin wrapper over the `wtac` function.
|
||||||
|
|
||||||
|
"""
|
||||||
|
|
||||||
|
def forward(self, probs, labels): # pylint: disable=no-self-use
|
||||||
|
return wtac(-1.0 * probs, labels)
|
||||||
|
|
||||||
|
|
||||||
|
class KNNC(torch.nn.Module):
|
||||||
|
"""K-Nearest-Neighbors-Competition.
|
||||||
|
|
||||||
|
Thin wrapper over the `knnc` function.
|
||||||
|
|
||||||
|
"""
|
||||||
|
|
||||||
|
def __init__(self, k=1, **kwargs):
|
||||||
|
super().__init__(**kwargs)
|
||||||
|
self.k = k
|
||||||
|
|
||||||
|
def forward(self, distances, labels):
|
||||||
|
return knnc(distances, labels, k=self.k)
|
||||||
|
|
||||||
|
def extra_repr(self):
|
||||||
|
return f"k: {self.k}"
|
||||||
|
|
||||||
|
|
||||||
|
class CBCC(torch.nn.Module):
|
||||||
|
"""Classification-By-Components Competition.
|
||||||
|
|
||||||
|
Thin wrapper over the `cbcc` function.
|
||||||
|
|
||||||
|
"""
|
||||||
|
|
||||||
|
def forward(self, detections, reasonings): # pylint: disable=no-self-use
|
||||||
|
return cbcc(detections, reasonings)
|
380
prototorch/core/components.py
Normal file
380
prototorch/core/components.py
Normal file
@ -0,0 +1,380 @@
|
|||||||
|
"""ProtoTorch components"""
|
||||||
|
|
||||||
|
import inspect
|
||||||
|
from typing import Union
|
||||||
|
|
||||||
|
import torch
|
||||||
|
from torch.nn.parameter import Parameter
|
||||||
|
|
||||||
|
from prototorch.utils import parse_distribution
|
||||||
|
|
||||||
|
from .initializers import (
|
||||||
|
AbstractClassAwareCompInitializer,
|
||||||
|
AbstractComponentsInitializer,
|
||||||
|
AbstractLabelsInitializer,
|
||||||
|
AbstractReasoningsInitializer,
|
||||||
|
LabelsInitializer,
|
||||||
|
PurePositiveReasoningsInitializer,
|
||||||
|
RandomReasoningsInitializer,
|
||||||
|
)
|
||||||
|
|
||||||
|
|
||||||
|
def validate_initializer(initializer, instanceof):
|
||||||
|
"""Check if the initializer is valid."""
|
||||||
|
if not isinstance(initializer, instanceof):
|
||||||
|
emsg = f"`initializer` has to be an instance " \
|
||||||
|
f"of some subtype of {instanceof}. " \
|
||||||
|
f"You have provided: {initializer} instead. "
|
||||||
|
helpmsg = ""
|
||||||
|
if inspect.isclass(initializer):
|
||||||
|
helpmsg = f"Perhaps you meant to say, {initializer.__name__}() " \
|
||||||
|
f"with the brackets instead of just {initializer.__name__}?"
|
||||||
|
raise TypeError(emsg + helpmsg)
|
||||||
|
return True
|
||||||
|
|
||||||
|
|
||||||
|
def gencat(ins, attr, init, *iargs, **ikwargs):
|
||||||
|
"""Generate new items and concatenate with existing items."""
|
||||||
|
new_items = init.generate(*iargs, **ikwargs)
|
||||||
|
if hasattr(ins, attr):
|
||||||
|
items = torch.cat([getattr(ins, attr), new_items])
|
||||||
|
else:
|
||||||
|
items = new_items
|
||||||
|
return items, new_items
|
||||||
|
|
||||||
|
|
||||||
|
def removeind(ins, attr, indices):
|
||||||
|
"""Remove items at specified indices."""
|
||||||
|
mask = torch.ones(len(ins), dtype=torch.bool)
|
||||||
|
mask[indices] = False
|
||||||
|
items = getattr(ins, attr)[mask]
|
||||||
|
return items, mask
|
||||||
|
|
||||||
|
|
||||||
|
def get_cikwargs(init, distribution):
|
||||||
|
"""Return appropriate key-word arguments for a component initializer."""
|
||||||
|
if isinstance(init, AbstractClassAwareCompInitializer):
|
||||||
|
cikwargs = dict(distribution=distribution)
|
||||||
|
else:
|
||||||
|
distribution = parse_distribution(distribution)
|
||||||
|
num_components = sum(distribution.values())
|
||||||
|
cikwargs = dict(num_components=num_components)
|
||||||
|
return cikwargs
|
||||||
|
|
||||||
|
|
||||||
|
class AbstractComponents(torch.nn.Module):
|
||||||
|
"""Abstract class for all components modules."""
|
||||||
|
|
||||||
|
@property
|
||||||
|
def num_components(self):
|
||||||
|
"""Current number of components."""
|
||||||
|
return len(self._components)
|
||||||
|
|
||||||
|
@property
|
||||||
|
def components(self):
|
||||||
|
"""Detached Tensor containing the components."""
|
||||||
|
return self._components.detach().cpu()
|
||||||
|
|
||||||
|
def _register_components(self, components):
|
||||||
|
self.register_parameter("_components", Parameter(components))
|
||||||
|
|
||||||
|
def extra_repr(self):
|
||||||
|
return f"components: (shape: {tuple(self._components.shape)})"
|
||||||
|
|
||||||
|
def __len__(self):
|
||||||
|
return self.num_components
|
||||||
|
|
||||||
|
|
||||||
|
class Components(AbstractComponents):
|
||||||
|
"""A set of adaptable Tensors."""
|
||||||
|
|
||||||
|
def __init__(self, num_components: int,
|
||||||
|
initializer: AbstractComponentsInitializer):
|
||||||
|
super().__init__()
|
||||||
|
self.add_components(num_components, initializer)
|
||||||
|
|
||||||
|
def add_components(self, num_components: int,
|
||||||
|
initializer: AbstractComponentsInitializer):
|
||||||
|
"""Generate and add new components."""
|
||||||
|
assert validate_initializer(initializer, AbstractComponentsInitializer)
|
||||||
|
_components, new_components = gencat(self, "_components", initializer,
|
||||||
|
num_components)
|
||||||
|
self._register_components(_components)
|
||||||
|
return new_components
|
||||||
|
|
||||||
|
def remove_components(self, indices):
|
||||||
|
"""Remove components at specified indices."""
|
||||||
|
_components, mask = removeind(self, "_components", indices)
|
||||||
|
self._register_components(_components)
|
||||||
|
return mask
|
||||||
|
|
||||||
|
def forward(self):
|
||||||
|
"""Simply return the components parameter Tensor."""
|
||||||
|
return self._components
|
||||||
|
|
||||||
|
|
||||||
|
class AbstractLabels(torch.nn.Module):
|
||||||
|
"""Abstract class for all labels modules."""
|
||||||
|
|
||||||
|
@property
|
||||||
|
def labels(self):
|
||||||
|
return self._labels.cpu()
|
||||||
|
|
||||||
|
@property
|
||||||
|
def num_labels(self):
|
||||||
|
return len(self._labels)
|
||||||
|
|
||||||
|
@property
|
||||||
|
def unique_labels(self):
|
||||||
|
return torch.unique(self._labels)
|
||||||
|
|
||||||
|
@property
|
||||||
|
def num_unique(self):
|
||||||
|
return len(self.unique_labels)
|
||||||
|
|
||||||
|
@property
|
||||||
|
def distribution(self):
|
||||||
|
unique, counts = torch.unique(self._labels,
|
||||||
|
sorted=True,
|
||||||
|
return_counts=True)
|
||||||
|
return dict(zip(unique.tolist(), counts.tolist()))
|
||||||
|
|
||||||
|
def _register_labels(self, labels):
|
||||||
|
self.register_buffer("_labels", labels)
|
||||||
|
|
||||||
|
def extra_repr(self):
|
||||||
|
r = f"num_labels: {self.num_labels}, num_unique: {self.num_unique}"
|
||||||
|
if len(self.distribution) < 11: # avoid lengthy representations
|
||||||
|
d = self.distribution
|
||||||
|
unique, counts = list(d.keys()), list(d.values())
|
||||||
|
r += f", unique: {unique}, counts: {counts}"
|
||||||
|
return r
|
||||||
|
|
||||||
|
def __len__(self):
|
||||||
|
return self.num_labels
|
||||||
|
|
||||||
|
|
||||||
|
class Labels(AbstractLabels):
|
||||||
|
"""A set of standalone labels."""
|
||||||
|
|
||||||
|
def __init__(self,
|
||||||
|
distribution: Union[dict, list, tuple],
|
||||||
|
initializer: AbstractLabelsInitializer = LabelsInitializer()):
|
||||||
|
super().__init__()
|
||||||
|
self.add_labels(distribution, initializer)
|
||||||
|
|
||||||
|
def add_labels(
|
||||||
|
self,
|
||||||
|
distribution: Union[dict, tuple, list],
|
||||||
|
initializer: AbstractLabelsInitializer = LabelsInitializer()):
|
||||||
|
"""Generate and add new labels."""
|
||||||
|
assert validate_initializer(initializer, AbstractLabelsInitializer)
|
||||||
|
_labels, new_labels = gencat(self, "_labels", initializer,
|
||||||
|
distribution)
|
||||||
|
self._register_labels(_labels)
|
||||||
|
return new_labels
|
||||||
|
|
||||||
|
def remove_labels(self, indices):
|
||||||
|
"""Remove labels at specified indices."""
|
||||||
|
_labels, mask = removeind(self, "_labels", indices)
|
||||||
|
self._register_labels(_labels)
|
||||||
|
return mask
|
||||||
|
|
||||||
|
def forward(self):
|
||||||
|
"""Simply return the labels."""
|
||||||
|
return self._labels
|
||||||
|
|
||||||
|
|
||||||
|
class LabeledComponents(AbstractComponents):
|
||||||
|
"""A set of adaptable components and corresponding unadaptable labels."""
|
||||||
|
|
||||||
|
def __init__(
|
||||||
|
self,
|
||||||
|
distribution: Union[dict, list, tuple],
|
||||||
|
components_initializer: AbstractComponentsInitializer,
|
||||||
|
labels_initializer: AbstractLabelsInitializer = LabelsInitializer()):
|
||||||
|
super().__init__()
|
||||||
|
self.add_components(distribution, components_initializer,
|
||||||
|
labels_initializer)
|
||||||
|
|
||||||
|
@property
|
||||||
|
def distribution(self):
|
||||||
|
unique, counts = torch.unique(self._labels,
|
||||||
|
sorted=True,
|
||||||
|
return_counts=True)
|
||||||
|
return dict(zip(unique.tolist(), counts.tolist()))
|
||||||
|
|
||||||
|
@property
|
||||||
|
def num_classes(self):
|
||||||
|
return len(self.distribution.keys())
|
||||||
|
|
||||||
|
@property
|
||||||
|
def labels(self):
|
||||||
|
"""Tensor containing the component labels."""
|
||||||
|
return self._labels.cpu()
|
||||||
|
|
||||||
|
def _register_labels(self, labels):
|
||||||
|
self.register_buffer("_labels", labels)
|
||||||
|
|
||||||
|
def add_components(
|
||||||
|
self,
|
||||||
|
distribution,
|
||||||
|
components_initializer,
|
||||||
|
labels_initializer: AbstractLabelsInitializer = LabelsInitializer()):
|
||||||
|
"""Generate and add new components and labels."""
|
||||||
|
assert validate_initializer(components_initializer,
|
||||||
|
AbstractComponentsInitializer)
|
||||||
|
assert validate_initializer(labels_initializer,
|
||||||
|
AbstractLabelsInitializer)
|
||||||
|
cikwargs = get_cikwargs(components_initializer, distribution)
|
||||||
|
_components, new_components = gencat(self, "_components",
|
||||||
|
components_initializer,
|
||||||
|
**cikwargs)
|
||||||
|
_labels, new_labels = gencat(self, "_labels", labels_initializer,
|
||||||
|
distribution)
|
||||||
|
self._register_components(_components)
|
||||||
|
self._register_labels(_labels)
|
||||||
|
return new_components, new_labels
|
||||||
|
|
||||||
|
def remove_components(self, indices):
|
||||||
|
"""Remove components and labels at specified indices."""
|
||||||
|
_components, mask = removeind(self, "_components", indices)
|
||||||
|
_labels, mask = removeind(self, "_labels", indices)
|
||||||
|
self._register_components(_components)
|
||||||
|
self._register_labels(_labels)
|
||||||
|
return mask
|
||||||
|
|
||||||
|
def forward(self):
|
||||||
|
"""Simply return the components parameter Tensor and labels."""
|
||||||
|
return self._components, self._labels
|
||||||
|
|
||||||
|
|
||||||
|
class Reasonings(torch.nn.Module):
|
||||||
|
"""A set of standalone reasoning matrices.
|
||||||
|
|
||||||
|
The `reasonings` tensor is of shape [num_components, num_classes, 2].
|
||||||
|
|
||||||
|
"""
|
||||||
|
|
||||||
|
def __init__(
|
||||||
|
self,
|
||||||
|
distribution: Union[dict, list, tuple],
|
||||||
|
initializer:
|
||||||
|
AbstractReasoningsInitializer = RandomReasoningsInitializer(),
|
||||||
|
):
|
||||||
|
super().__init__()
|
||||||
|
self.add_reasonings(distribution, initializer)
|
||||||
|
|
||||||
|
@property
|
||||||
|
def num_classes(self):
|
||||||
|
return self._reasonings.shape[1]
|
||||||
|
|
||||||
|
@property
|
||||||
|
def reasonings(self):
|
||||||
|
"""Tensor containing the reasoning matrices."""
|
||||||
|
return self._reasonings.detach().cpu()
|
||||||
|
|
||||||
|
def _register_reasonings(self, reasonings):
|
||||||
|
self.register_buffer("_reasonings", reasonings)
|
||||||
|
|
||||||
|
def add_reasonings(
|
||||||
|
self,
|
||||||
|
distribution: Union[dict, list, tuple],
|
||||||
|
initializer:
|
||||||
|
AbstractReasoningsInitializer = RandomReasoningsInitializer()):
|
||||||
|
"""Generate and add new reasonings."""
|
||||||
|
assert validate_initializer(initializer, AbstractReasoningsInitializer)
|
||||||
|
_reasonings, new_reasonings = gencat(self, "_reasonings", initializer,
|
||||||
|
distribution)
|
||||||
|
self._register_reasonings(_reasonings)
|
||||||
|
return new_reasonings
|
||||||
|
|
||||||
|
def remove_reasonings(self, indices):
|
||||||
|
"""Remove reasonings at specified indices."""
|
||||||
|
_reasonings, mask = removeind(self, "_reasonings", indices)
|
||||||
|
self._register_reasonings(_reasonings)
|
||||||
|
return mask
|
||||||
|
|
||||||
|
def forward(self):
|
||||||
|
"""Simply return the reasonings."""
|
||||||
|
return self._reasonings
|
||||||
|
|
||||||
|
|
||||||
|
class ReasoningComponents(AbstractComponents):
|
||||||
|
r"""A set of components and a corresponding adapatable reasoning matrices.
|
||||||
|
|
||||||
|
Every component has its own reasoning matrix.
|
||||||
|
|
||||||
|
A reasoning matrix is an Nx2 matrix, where N is the number of classes. The
|
||||||
|
first element is called positive reasoning :math:`p`, the second negative
|
||||||
|
reasoning :math:`n`. A components can reason in favour (positive) of a
|
||||||
|
class, against (negative) a class or not at all (neutral).
|
||||||
|
|
||||||
|
It holds that :math:`0 \leq n \leq 1`, :math:`0 \leq p \leq 1` and :math:`0
|
||||||
|
\leq n+p \leq 1`. Therefore :math:`n` and :math:`p` are two elements of a
|
||||||
|
three element probability distribution.
|
||||||
|
|
||||||
|
"""
|
||||||
|
|
||||||
|
def __init__(
|
||||||
|
self,
|
||||||
|
distribution: Union[dict, list, tuple],
|
||||||
|
components_initializer: AbstractComponentsInitializer,
|
||||||
|
reasonings_initializer:
|
||||||
|
AbstractReasoningsInitializer = PurePositiveReasoningsInitializer()):
|
||||||
|
super().__init__()
|
||||||
|
self.add_components(distribution, components_initializer,
|
||||||
|
reasonings_initializer)
|
||||||
|
|
||||||
|
@property
|
||||||
|
def num_classes(self):
|
||||||
|
return self._reasonings.shape[1]
|
||||||
|
|
||||||
|
@property
|
||||||
|
def reasonings(self):
|
||||||
|
"""Tensor containing the reasoning matrices."""
|
||||||
|
return self._reasonings.detach().cpu()
|
||||||
|
|
||||||
|
@property
|
||||||
|
def reasoning_matrices(self):
|
||||||
|
"""Reasoning matrices for each class."""
|
||||||
|
with torch.no_grad():
|
||||||
|
A, B = self._reasonings.permute(2, 1, 0).clamp(0, 1)
|
||||||
|
pk = A
|
||||||
|
nk = (1 - pk) * B
|
||||||
|
ik = 1 - pk - nk
|
||||||
|
matrices = torch.stack([pk, nk, ik], dim=-1).permute(1, 2, 0)
|
||||||
|
return matrices.cpu()
|
||||||
|
|
||||||
|
def _register_reasonings(self, reasonings):
|
||||||
|
self.register_parameter("_reasonings", Parameter(reasonings))
|
||||||
|
|
||||||
|
def add_components(self, distribution, components_initializer,
|
||||||
|
reasonings_initializer: AbstractReasoningsInitializer):
|
||||||
|
"""Generate and add new components and reasonings."""
|
||||||
|
assert validate_initializer(components_initializer,
|
||||||
|
AbstractComponentsInitializer)
|
||||||
|
assert validate_initializer(reasonings_initializer,
|
||||||
|
AbstractReasoningsInitializer)
|
||||||
|
cikwargs = get_cikwargs(components_initializer, distribution)
|
||||||
|
_components, new_components = gencat(self, "_components",
|
||||||
|
components_initializer,
|
||||||
|
**cikwargs)
|
||||||
|
_reasonings, new_reasonings = gencat(self, "_reasonings",
|
||||||
|
reasonings_initializer,
|
||||||
|
distribution)
|
||||||
|
self._register_components(_components)
|
||||||
|
self._register_reasonings(_reasonings)
|
||||||
|
return new_components, new_reasonings
|
||||||
|
|
||||||
|
def remove_components(self, indices):
|
||||||
|
"""Remove components and reasonings at specified indices."""
|
||||||
|
_components, mask = removeind(self, "_components", indices)
|
||||||
|
_reasonings, mask = removeind(self, "_reasonings", indices)
|
||||||
|
self._register_components(_components)
|
||||||
|
self._register_reasonings(_reasonings)
|
||||||
|
return mask
|
||||||
|
|
||||||
|
def forward(self):
|
||||||
|
"""Simply return the components and reasonings."""
|
||||||
|
return self._components, self._reasonings
|
95
prototorch/core/distances.py
Normal file
95
prototorch/core/distances.py
Normal file
@ -0,0 +1,95 @@
|
|||||||
|
"""ProtoTorch distances"""
|
||||||
|
|
||||||
|
import torch
|
||||||
|
|
||||||
|
|
||||||
|
def squared_euclidean_distance(x, y):
|
||||||
|
r"""Compute the squared Euclidean distance between :math:`\bm x` and :math:`\bm y`.
|
||||||
|
|
||||||
|
Compute :math:`{\langle \bm x - \bm y \rangle}_2`
|
||||||
|
|
||||||
|
**Alias:**
|
||||||
|
``prototorch.functions.distances.sed``
|
||||||
|
"""
|
||||||
|
x, y = (arr.view(arr.size(0), -1) for arr in (x, y))
|
||||||
|
expanded_x = x.unsqueeze(dim=1)
|
||||||
|
batchwise_difference = y - expanded_x
|
||||||
|
differences_raised = torch.pow(batchwise_difference, 2)
|
||||||
|
distances = torch.sum(differences_raised, axis=2)
|
||||||
|
return distances
|
||||||
|
|
||||||
|
|
||||||
|
def euclidean_distance(x, y):
|
||||||
|
r"""Compute the Euclidean distance between :math:`x` and :math:`y`.
|
||||||
|
|
||||||
|
Compute :math:`\sqrt{{\langle \bm x - \bm y \rangle}_2}`
|
||||||
|
|
||||||
|
:returns: Distance Tensor of shape :math:`X \times Y`
|
||||||
|
:rtype: `torch.tensor`
|
||||||
|
"""
|
||||||
|
x, y = (arr.view(arr.size(0), -1) for arr in (x, y))
|
||||||
|
distances_raised = squared_euclidean_distance(x, y)
|
||||||
|
distances = torch.sqrt(distances_raised)
|
||||||
|
return distances
|
||||||
|
|
||||||
|
|
||||||
|
def euclidean_distance_v2(x, y):
|
||||||
|
x, y = (arr.view(arr.size(0), -1) for arr in (x, y))
|
||||||
|
diff = y - x.unsqueeze(1)
|
||||||
|
pairwise_distances = (diff @ diff.permute((0, 2, 1))).sqrt()
|
||||||
|
# Passing `dim1=-2` and `dim2=-1` to `diagonal()` takes the
|
||||||
|
# batch diagonal. See:
|
||||||
|
# https://pytorch.org/docs/stable/generated/torch.diagonal.html
|
||||||
|
distances = torch.diagonal(pairwise_distances, dim1=-2, dim2=-1)
|
||||||
|
return distances
|
||||||
|
|
||||||
|
|
||||||
|
def lpnorm_distance(x, y, p):
|
||||||
|
r"""Calculate the lp-norm between :math:`\bm x` and :math:`\bm y`.
|
||||||
|
Also known as Minkowski distance.
|
||||||
|
|
||||||
|
Compute :math:`{\| \bm x - \bm y \|}_p`.
|
||||||
|
|
||||||
|
Calls ``torch.cdist``
|
||||||
|
|
||||||
|
:param p: p parameter of the lp norm
|
||||||
|
"""
|
||||||
|
x, y = (arr.view(arr.size(0), -1) for arr in (x, y))
|
||||||
|
distances = torch.cdist(x, y, p=p)
|
||||||
|
return distances
|
||||||
|
|
||||||
|
|
||||||
|
def omega_distance(x, y, omega):
|
||||||
|
r"""Omega distance.
|
||||||
|
|
||||||
|
Compute :math:`{\| \Omega \bm x - \Omega \bm y \|}_p`
|
||||||
|
|
||||||
|
:param `torch.tensor` omega: Two dimensional matrix
|
||||||
|
"""
|
||||||
|
x, y = (arr.view(arr.size(0), -1) for arr in (x, y))
|
||||||
|
projected_x = x @ omega
|
||||||
|
projected_y = y @ omega
|
||||||
|
distances = squared_euclidean_distance(projected_x, projected_y)
|
||||||
|
return distances
|
||||||
|
|
||||||
|
|
||||||
|
def lomega_distance(x, y, omegas):
|
||||||
|
r"""Localized Omega distance.
|
||||||
|
|
||||||
|
Compute :math:`{\| \Omega_k \bm x - \Omega_k \bm y_k \|}_p`
|
||||||
|
|
||||||
|
:param `torch.tensor` omegas: Three dimensional matrix
|
||||||
|
"""
|
||||||
|
x, y = (arr.view(arr.size(0), -1) for arr in (x, y))
|
||||||
|
projected_x = x @ omegas
|
||||||
|
projected_y = torch.diagonal(y @ omegas).T
|
||||||
|
expanded_y = torch.unsqueeze(projected_y, dim=1)
|
||||||
|
batchwise_difference = expanded_y - projected_x
|
||||||
|
differences_squared = batchwise_difference**2
|
||||||
|
distances = torch.sum(differences_squared, dim=2)
|
||||||
|
distances = distances.permute(1, 0)
|
||||||
|
return distances
|
||||||
|
|
||||||
|
|
||||||
|
# Aliases
|
||||||
|
sed = squared_euclidean_distance
|
555
prototorch/core/initializers.py
Normal file
555
prototorch/core/initializers.py
Normal file
@ -0,0 +1,555 @@
|
|||||||
|
"""ProtoTorch code initializers"""
|
||||||
|
|
||||||
|
import warnings
|
||||||
|
from abc import ABC, abstractmethod
|
||||||
|
from collections.abc import Iterable
|
||||||
|
from typing import (
|
||||||
|
Callable,
|
||||||
|
Type,
|
||||||
|
Union,
|
||||||
|
)
|
||||||
|
|
||||||
|
import torch
|
||||||
|
|
||||||
|
from prototorch.utils import parse_data_arg, parse_distribution
|
||||||
|
|
||||||
|
|
||||||
|
# Components
|
||||||
|
class AbstractComponentsInitializer(ABC):
|
||||||
|
"""Abstract class for all components initializers."""
|
||||||
|
...
|
||||||
|
|
||||||
|
|
||||||
|
class LiteralCompInitializer(AbstractComponentsInitializer):
|
||||||
|
"""'Generate' the provided components.
|
||||||
|
|
||||||
|
Use this to 'generate' pre-initialized components elsewhere.
|
||||||
|
|
||||||
|
"""
|
||||||
|
|
||||||
|
def __init__(self, components):
|
||||||
|
self.components = components
|
||||||
|
|
||||||
|
def generate(self, num_components: int = 0):
|
||||||
|
"""Ignore `num_components` and simply return `self.components`."""
|
||||||
|
provided_num_components = len(self.components)
|
||||||
|
if provided_num_components != num_components:
|
||||||
|
wmsg = f"The number of components ({provided_num_components}) " \
|
||||||
|
f"provided to {self.__class__.__name__} " \
|
||||||
|
f"does not match the expected number ({num_components})."
|
||||||
|
warnings.warn(wmsg)
|
||||||
|
if not isinstance(self.components, torch.Tensor):
|
||||||
|
wmsg = f"Converting components to {torch.Tensor}..."
|
||||||
|
warnings.warn(wmsg)
|
||||||
|
self.components = torch.Tensor(self.components)
|
||||||
|
return self.components
|
||||||
|
|
||||||
|
|
||||||
|
class ShapeAwareCompInitializer(AbstractComponentsInitializer):
|
||||||
|
"""Abstract class for all dimension-aware components initializers."""
|
||||||
|
|
||||||
|
def __init__(self, shape: Union[Iterable, int]):
|
||||||
|
if isinstance(shape, Iterable):
|
||||||
|
self.component_shape = tuple(shape)
|
||||||
|
else:
|
||||||
|
self.component_shape = (shape, )
|
||||||
|
|
||||||
|
@abstractmethod
|
||||||
|
def generate(self, num_components: int):
|
||||||
|
...
|
||||||
|
|
||||||
|
|
||||||
|
class ZerosCompInitializer(ShapeAwareCompInitializer):
|
||||||
|
"""Generate zeros corresponding to the components shape."""
|
||||||
|
|
||||||
|
def generate(self, num_components: int):
|
||||||
|
components = torch.zeros((num_components, ) + self.component_shape)
|
||||||
|
return components
|
||||||
|
|
||||||
|
|
||||||
|
class OnesCompInitializer(ShapeAwareCompInitializer):
|
||||||
|
"""Generate ones corresponding to the components shape."""
|
||||||
|
|
||||||
|
def generate(self, num_components: int):
|
||||||
|
components = torch.ones((num_components, ) + self.component_shape)
|
||||||
|
return components
|
||||||
|
|
||||||
|
|
||||||
|
class FillValueCompInitializer(OnesCompInitializer):
|
||||||
|
"""Generate components with the provided `fill_value`."""
|
||||||
|
|
||||||
|
def __init__(self, shape, fill_value: float = 1.0):
|
||||||
|
super().__init__(shape)
|
||||||
|
self.fill_value = fill_value
|
||||||
|
|
||||||
|
def generate(self, num_components: int):
|
||||||
|
ones = super().generate(num_components)
|
||||||
|
components = ones.fill_(self.fill_value)
|
||||||
|
return components
|
||||||
|
|
||||||
|
|
||||||
|
class UniformCompInitializer(OnesCompInitializer):
|
||||||
|
"""Generate components by sampling from a continuous uniform distribution."""
|
||||||
|
|
||||||
|
def __init__(self, shape, minimum=0.0, maximum=1.0, scale=1.0):
|
||||||
|
super().__init__(shape)
|
||||||
|
self.minimum = minimum
|
||||||
|
self.maximum = maximum
|
||||||
|
self.scale = scale
|
||||||
|
|
||||||
|
def generate(self, num_components: int):
|
||||||
|
ones = super().generate(num_components)
|
||||||
|
components = self.scale * ones.uniform_(self.minimum, self.maximum)
|
||||||
|
return components
|
||||||
|
|
||||||
|
|
||||||
|
class RandomNormalCompInitializer(OnesCompInitializer):
|
||||||
|
"""Generate components by sampling from a standard normal distribution."""
|
||||||
|
|
||||||
|
def __init__(self, shape, shift=0.0, scale=1.0):
|
||||||
|
super().__init__(shape)
|
||||||
|
self.shift = shift
|
||||||
|
self.scale = scale
|
||||||
|
|
||||||
|
def generate(self, num_components: int):
|
||||||
|
ones = super().generate(num_components)
|
||||||
|
components = self.scale * (torch.randn_like(ones) + self.shift)
|
||||||
|
return components
|
||||||
|
|
||||||
|
|
||||||
|
class AbstractDataAwareCompInitializer(AbstractComponentsInitializer):
|
||||||
|
"""Abstract class for all data-aware components initializers.
|
||||||
|
|
||||||
|
Components generated by data-aware components initializers inherit the shape
|
||||||
|
of the provided data.
|
||||||
|
|
||||||
|
`data` has to be a torch tensor.
|
||||||
|
|
||||||
|
"""
|
||||||
|
|
||||||
|
def __init__(self,
|
||||||
|
data: torch.Tensor,
|
||||||
|
noise: float = 0.0,
|
||||||
|
transform: Callable = torch.nn.Identity()):
|
||||||
|
self.data = data
|
||||||
|
self.noise = noise
|
||||||
|
self.transform = transform
|
||||||
|
|
||||||
|
def generate_end_hook(self, samples):
|
||||||
|
drift = torch.rand_like(samples) * self.noise
|
||||||
|
components = self.transform(samples + drift)
|
||||||
|
return components
|
||||||
|
|
||||||
|
@abstractmethod
|
||||||
|
def generate(self, num_components: int):
|
||||||
|
...
|
||||||
|
return self.generate_end_hook(...)
|
||||||
|
|
||||||
|
def __del__(self):
|
||||||
|
del self.data
|
||||||
|
|
||||||
|
|
||||||
|
class DataAwareCompInitializer(AbstractDataAwareCompInitializer):
|
||||||
|
"""'Generate' the components from the provided data."""
|
||||||
|
|
||||||
|
def generate(self, num_components: int = 0):
|
||||||
|
"""Ignore `num_components` and simply return transformed `self.data`."""
|
||||||
|
components = self.generate_end_hook(self.data)
|
||||||
|
return components
|
||||||
|
|
||||||
|
|
||||||
|
class SelectionCompInitializer(AbstractDataAwareCompInitializer):
|
||||||
|
"""Generate components by uniformly sampling from the provided data."""
|
||||||
|
|
||||||
|
def generate(self, num_components: int):
|
||||||
|
indices = torch.LongTensor(num_components).random_(0, len(self.data))
|
||||||
|
samples = self.data[indices]
|
||||||
|
components = self.generate_end_hook(samples)
|
||||||
|
return components
|
||||||
|
|
||||||
|
|
||||||
|
class MeanCompInitializer(AbstractDataAwareCompInitializer):
|
||||||
|
"""Generate components by computing the mean of the provided data."""
|
||||||
|
|
||||||
|
def generate(self, num_components: int):
|
||||||
|
mean = self.data.mean(dim=0)
|
||||||
|
repeat_dim = [num_components] + [1] * len(mean.shape)
|
||||||
|
samples = mean.repeat(repeat_dim)
|
||||||
|
components = self.generate_end_hook(samples)
|
||||||
|
return components
|
||||||
|
|
||||||
|
|
||||||
|
class AbstractClassAwareCompInitializer(AbstractComponentsInitializer):
|
||||||
|
"""Abstract class for all class-aware components initializers.
|
||||||
|
|
||||||
|
Components generated by class-aware components initializers inherit the shape
|
||||||
|
of the provided data.
|
||||||
|
|
||||||
|
`data` could be a torch Dataset or DataLoader or a list/tuple of data and
|
||||||
|
target tensors.
|
||||||
|
|
||||||
|
"""
|
||||||
|
|
||||||
|
def __init__(self,
|
||||||
|
data,
|
||||||
|
noise: float = 0.0,
|
||||||
|
transform: Callable = torch.nn.Identity()):
|
||||||
|
self.data, self.targets = parse_data_arg(data)
|
||||||
|
self.noise = noise
|
||||||
|
self.transform = transform
|
||||||
|
self.clabels = torch.unique(self.targets).int().tolist()
|
||||||
|
self.num_classes = len(self.clabels)
|
||||||
|
|
||||||
|
def generate_end_hook(self, samples):
|
||||||
|
drift = torch.rand_like(samples) * self.noise
|
||||||
|
components = self.transform(samples + drift)
|
||||||
|
return components
|
||||||
|
|
||||||
|
@abstractmethod
|
||||||
|
def generate(self, distribution: Union[dict, list, tuple]):
|
||||||
|
...
|
||||||
|
return self.generate_end_hook(...)
|
||||||
|
|
||||||
|
def __del__(self):
|
||||||
|
del self.data
|
||||||
|
del self.targets
|
||||||
|
|
||||||
|
|
||||||
|
class ClassAwareCompInitializer(AbstractClassAwareCompInitializer):
|
||||||
|
"""'Generate' components from provided data and requested distribution."""
|
||||||
|
|
||||||
|
def generate(self, distribution: Union[dict, list, tuple]):
|
||||||
|
"""Ignore `distribution` and simply return transformed `self.data`."""
|
||||||
|
components = self.generate_end_hook(self.data)
|
||||||
|
return components
|
||||||
|
|
||||||
|
|
||||||
|
class AbstractStratifiedCompInitializer(AbstractClassAwareCompInitializer):
|
||||||
|
"""Abstract class for all stratified components initializers."""
|
||||||
|
|
||||||
|
@property
|
||||||
|
@abstractmethod
|
||||||
|
def subinit_type(self) -> Type[AbstractDataAwareCompInitializer]:
|
||||||
|
...
|
||||||
|
|
||||||
|
def generate(self, distribution: Union[dict, list, tuple]):
|
||||||
|
distribution = parse_distribution(distribution)
|
||||||
|
components = torch.tensor([])
|
||||||
|
for k, v in distribution.items():
|
||||||
|
stratified_data = self.data[self.targets == k]
|
||||||
|
if len(stratified_data) == 0:
|
||||||
|
raise ValueError(f"No data available for class {k}.")
|
||||||
|
initializer = self.subinit_type(
|
||||||
|
stratified_data,
|
||||||
|
noise=self.noise,
|
||||||
|
transform=self.transform,
|
||||||
|
)
|
||||||
|
samples = initializer.generate(num_components=v)
|
||||||
|
components = torch.cat([components, samples])
|
||||||
|
return components
|
||||||
|
|
||||||
|
|
||||||
|
class StratifiedSelectionCompInitializer(AbstractStratifiedCompInitializer):
|
||||||
|
"""Generate components using stratified sampling from the provided data."""
|
||||||
|
|
||||||
|
@property
|
||||||
|
def subinit_type(self):
|
||||||
|
return SelectionCompInitializer
|
||||||
|
|
||||||
|
|
||||||
|
class StratifiedMeanCompInitializer(AbstractStratifiedCompInitializer):
|
||||||
|
"""Generate components at stratified means of the provided data."""
|
||||||
|
|
||||||
|
@property
|
||||||
|
def subinit_type(self):
|
||||||
|
return MeanCompInitializer
|
||||||
|
|
||||||
|
|
||||||
|
# Labels
|
||||||
|
class AbstractLabelsInitializer(ABC):
|
||||||
|
"""Abstract class for all labels initializers."""
|
||||||
|
|
||||||
|
@abstractmethod
|
||||||
|
def generate(self, distribution: Union[dict, list, tuple]):
|
||||||
|
...
|
||||||
|
|
||||||
|
|
||||||
|
class LiteralLabelsInitializer(AbstractLabelsInitializer):
|
||||||
|
"""'Generate' the provided labels.
|
||||||
|
|
||||||
|
Use this to 'generate' pre-initialized labels elsewhere.
|
||||||
|
|
||||||
|
"""
|
||||||
|
|
||||||
|
def __init__(self, labels):
|
||||||
|
self.labels = labels
|
||||||
|
|
||||||
|
def generate(self, distribution: Union[dict, list, tuple]):
|
||||||
|
"""Ignore `distribution` and simply return `self.labels`.
|
||||||
|
|
||||||
|
Convert to long tensor, if necessary.
|
||||||
|
"""
|
||||||
|
labels = self.labels
|
||||||
|
if not isinstance(labels, torch.LongTensor):
|
||||||
|
wmsg = f"Converting labels to {torch.LongTensor}..."
|
||||||
|
warnings.warn(wmsg)
|
||||||
|
labels = torch.LongTensor(labels)
|
||||||
|
return labels
|
||||||
|
|
||||||
|
|
||||||
|
class DataAwareLabelsInitializer(AbstractLabelsInitializer):
|
||||||
|
"""'Generate' the labels from a torch Dataset."""
|
||||||
|
|
||||||
|
def __init__(self, data):
|
||||||
|
self.data, self.targets = parse_data_arg(data)
|
||||||
|
|
||||||
|
def generate(self, distribution: Union[dict, list, tuple]):
|
||||||
|
"""Ignore `num_components` and simply return `self.targets`."""
|
||||||
|
return self.targets
|
||||||
|
|
||||||
|
|
||||||
|
class LabelsInitializer(AbstractLabelsInitializer):
|
||||||
|
"""Generate labels from `distribution`."""
|
||||||
|
|
||||||
|
def generate(self, distribution: Union[dict, list, tuple]):
|
||||||
|
distribution = parse_distribution(distribution)
|
||||||
|
labels_list = []
|
||||||
|
for k, v in distribution.items():
|
||||||
|
labels_list.extend([k] * v)
|
||||||
|
labels = torch.LongTensor(labels_list)
|
||||||
|
return labels
|
||||||
|
|
||||||
|
|
||||||
|
class OneHotLabelsInitializer(LabelsInitializer):
|
||||||
|
"""Generate one-hot-encoded labels from `distribution`."""
|
||||||
|
|
||||||
|
def generate(self, distribution: Union[dict, list, tuple]):
|
||||||
|
distribution = parse_distribution(distribution)
|
||||||
|
num_classes = len(distribution.keys())
|
||||||
|
# this breaks if class labels are not [0,...,nclasses]
|
||||||
|
labels = torch.eye(num_classes)[super().generate(distribution)]
|
||||||
|
return labels
|
||||||
|
|
||||||
|
|
||||||
|
# Reasonings
|
||||||
|
def compute_distribution_shape(distribution):
|
||||||
|
distribution = parse_distribution(distribution)
|
||||||
|
num_components = sum(distribution.values())
|
||||||
|
num_classes = len(distribution.keys())
|
||||||
|
return (num_components, num_classes, 2)
|
||||||
|
|
||||||
|
|
||||||
|
class AbstractReasoningsInitializer(ABC):
|
||||||
|
"""Abstract class for all reasonings initializers."""
|
||||||
|
|
||||||
|
def __init__(self, components_first: bool = True):
|
||||||
|
self.components_first = components_first
|
||||||
|
|
||||||
|
def generate_end_hook(self, reasonings):
|
||||||
|
if not self.components_first:
|
||||||
|
reasonings = reasonings.permute(2, 1, 0)
|
||||||
|
return reasonings
|
||||||
|
|
||||||
|
@abstractmethod
|
||||||
|
def generate(self, distribution: Union[dict, list, tuple]):
|
||||||
|
...
|
||||||
|
return self.generate_end_hook(...)
|
||||||
|
|
||||||
|
|
||||||
|
class LiteralReasoningsInitializer(AbstractReasoningsInitializer):
|
||||||
|
"""'Generate' the provided reasonings.
|
||||||
|
|
||||||
|
Use this to 'generate' pre-initialized reasonings elsewhere.
|
||||||
|
|
||||||
|
"""
|
||||||
|
|
||||||
|
def __init__(self, reasonings, **kwargs):
|
||||||
|
super().__init__(**kwargs)
|
||||||
|
self.reasonings = reasonings
|
||||||
|
|
||||||
|
def generate(self, distribution: Union[dict, list, tuple]):
|
||||||
|
"""Ignore `distributuion` and simply return self.reasonings."""
|
||||||
|
reasonings = self.reasonings
|
||||||
|
if not isinstance(reasonings, torch.Tensor):
|
||||||
|
wmsg = f"Converting reasonings to {torch.Tensor}..."
|
||||||
|
warnings.warn(wmsg)
|
||||||
|
reasonings = torch.Tensor(reasonings)
|
||||||
|
reasonings = self.generate_end_hook(reasonings)
|
||||||
|
return reasonings
|
||||||
|
|
||||||
|
|
||||||
|
class ZerosReasoningsInitializer(AbstractReasoningsInitializer):
|
||||||
|
"""Reasonings are all initialized with zeros."""
|
||||||
|
|
||||||
|
def generate(self, distribution: Union[dict, list, tuple]):
|
||||||
|
shape = compute_distribution_shape(distribution)
|
||||||
|
reasonings = torch.zeros(*shape)
|
||||||
|
reasonings = self.generate_end_hook(reasonings)
|
||||||
|
return reasonings
|
||||||
|
|
||||||
|
|
||||||
|
class OnesReasoningsInitializer(AbstractReasoningsInitializer):
|
||||||
|
"""Reasonings are all initialized with ones."""
|
||||||
|
|
||||||
|
def generate(self, distribution: Union[dict, list, tuple]):
|
||||||
|
shape = compute_distribution_shape(distribution)
|
||||||
|
reasonings = torch.ones(*shape)
|
||||||
|
reasonings = self.generate_end_hook(reasonings)
|
||||||
|
return reasonings
|
||||||
|
|
||||||
|
|
||||||
|
class RandomReasoningsInitializer(AbstractReasoningsInitializer):
|
||||||
|
"""Reasonings are randomly initialized."""
|
||||||
|
|
||||||
|
def __init__(self, minimum=0.4, maximum=0.6, **kwargs):
|
||||||
|
super().__init__(**kwargs)
|
||||||
|
self.minimum = minimum
|
||||||
|
self.maximum = maximum
|
||||||
|
|
||||||
|
def generate(self, distribution: Union[dict, list, tuple]):
|
||||||
|
shape = compute_distribution_shape(distribution)
|
||||||
|
reasonings = torch.ones(*shape).uniform_(self.minimum, self.maximum)
|
||||||
|
reasonings = self.generate_end_hook(reasonings)
|
||||||
|
return reasonings
|
||||||
|
|
||||||
|
|
||||||
|
class PurePositiveReasoningsInitializer(AbstractReasoningsInitializer):
|
||||||
|
"""Each component reasons positively for exactly one class."""
|
||||||
|
|
||||||
|
def generate(self, distribution: Union[dict, list, tuple]):
|
||||||
|
num_components, num_classes, _ = compute_distribution_shape(
|
||||||
|
distribution)
|
||||||
|
A = OneHotLabelsInitializer().generate(distribution)
|
||||||
|
B = torch.zeros(num_components, num_classes)
|
||||||
|
reasonings = torch.stack([A, B], dim=-1)
|
||||||
|
reasonings = self.generate_end_hook(reasonings)
|
||||||
|
return reasonings
|
||||||
|
|
||||||
|
|
||||||
|
# Transforms
|
||||||
|
class AbstractTransformInitializer(ABC):
|
||||||
|
"""Abstract class for all transform initializers."""
|
||||||
|
...
|
||||||
|
|
||||||
|
|
||||||
|
class AbstractLinearTransformInitializer(AbstractTransformInitializer):
|
||||||
|
"""Abstract class for all linear transform initializers."""
|
||||||
|
|
||||||
|
def __init__(self, out_dim_first: bool = False):
|
||||||
|
self.out_dim_first = out_dim_first
|
||||||
|
|
||||||
|
def generate_end_hook(self, weights):
|
||||||
|
if self.out_dim_first:
|
||||||
|
weights = weights.permute(1, 0)
|
||||||
|
return weights
|
||||||
|
|
||||||
|
@abstractmethod
|
||||||
|
def generate(self, in_dim: int, out_dim: int):
|
||||||
|
...
|
||||||
|
return self.generate_end_hook(...)
|
||||||
|
|
||||||
|
|
||||||
|
class ZerosLinearTransformInitializer(AbstractLinearTransformInitializer):
|
||||||
|
"""Initialize a matrix with zeros."""
|
||||||
|
|
||||||
|
def generate(self, in_dim: int, out_dim: int):
|
||||||
|
weights = torch.zeros(in_dim, out_dim)
|
||||||
|
return self.generate_end_hook(weights)
|
||||||
|
|
||||||
|
|
||||||
|
class OnesLinearTransformInitializer(AbstractLinearTransformInitializer):
|
||||||
|
"""Initialize a matrix with ones."""
|
||||||
|
|
||||||
|
def generate(self, in_dim: int, out_dim: int):
|
||||||
|
weights = torch.ones(in_dim, out_dim)
|
||||||
|
return self.generate_end_hook(weights)
|
||||||
|
|
||||||
|
|
||||||
|
class RandomLinearTransformInitializer(AbstractLinearTransformInitializer):
|
||||||
|
"""Initialize a matrix with random values."""
|
||||||
|
|
||||||
|
def generate(self, in_dim: int, out_dim: int):
|
||||||
|
weights = torch.rand(in_dim, out_dim)
|
||||||
|
return self.generate_end_hook(weights)
|
||||||
|
|
||||||
|
|
||||||
|
class EyeLinearTransformInitializer(AbstractLinearTransformInitializer):
|
||||||
|
"""Initialize a matrix with the largest possible identity matrix."""
|
||||||
|
|
||||||
|
def generate(self, in_dim: int, out_dim: int):
|
||||||
|
weights = torch.zeros(in_dim, out_dim)
|
||||||
|
I = torch.eye(min(in_dim, out_dim))
|
||||||
|
weights[:I.shape[0], :I.shape[1]] = I
|
||||||
|
return self.generate_end_hook(weights)
|
||||||
|
|
||||||
|
|
||||||
|
class AbstractDataAwareLTInitializer(AbstractLinearTransformInitializer):
|
||||||
|
"""Abstract class for all data-aware linear transform initializers."""
|
||||||
|
|
||||||
|
def __init__(self,
|
||||||
|
data: torch.Tensor,
|
||||||
|
noise: float = 0.0,
|
||||||
|
transform: Callable = torch.nn.Identity(),
|
||||||
|
out_dim_first: bool = False):
|
||||||
|
super().__init__(out_dim_first)
|
||||||
|
self.data = data
|
||||||
|
self.noise = noise
|
||||||
|
self.transform = transform
|
||||||
|
|
||||||
|
def generate_end_hook(self, weights: torch.Tensor):
|
||||||
|
drift = torch.rand_like(weights) * self.noise
|
||||||
|
weights = self.transform(weights + drift)
|
||||||
|
if self.out_dim_first:
|
||||||
|
weights = weights.permute(1, 0)
|
||||||
|
return weights
|
||||||
|
|
||||||
|
|
||||||
|
class PCALinearTransformInitializer(AbstractDataAwareLTInitializer):
|
||||||
|
"""Initialize a matrix with Eigenvectors from the data."""
|
||||||
|
|
||||||
|
def generate(self, in_dim: int, out_dim: int):
|
||||||
|
_, _, weights = torch.pca_lowrank(self.data, q=out_dim)
|
||||||
|
return self.generate_end_hook(weights)
|
||||||
|
|
||||||
|
|
||||||
|
class LiteralLinearTransformInitializer(AbstractDataAwareLTInitializer):
|
||||||
|
"""'Generate' the provided weights."""
|
||||||
|
|
||||||
|
def generate(self, in_dim: int, out_dim: int):
|
||||||
|
return self.generate_end_hook(self.data)
|
||||||
|
|
||||||
|
|
||||||
|
# Aliases - Components
|
||||||
|
CACI = ClassAwareCompInitializer
|
||||||
|
DACI = DataAwareCompInitializer
|
||||||
|
FVCI = FillValueCompInitializer
|
||||||
|
LCI = LiteralCompInitializer
|
||||||
|
MCI = MeanCompInitializer
|
||||||
|
OCI = OnesCompInitializer
|
||||||
|
RNCI = RandomNormalCompInitializer
|
||||||
|
SCI = SelectionCompInitializer
|
||||||
|
SMCI = StratifiedMeanCompInitializer
|
||||||
|
SSCI = StratifiedSelectionCompInitializer
|
||||||
|
UCI = UniformCompInitializer
|
||||||
|
ZCI = ZerosCompInitializer
|
||||||
|
|
||||||
|
# Aliases - Labels
|
||||||
|
DLI = DataAwareLabelsInitializer
|
||||||
|
LI = LabelsInitializer
|
||||||
|
LLI = LiteralLabelsInitializer
|
||||||
|
OHLI = OneHotLabelsInitializer
|
||||||
|
|
||||||
|
# Aliases - Reasonings
|
||||||
|
LRI = LiteralReasoningsInitializer
|
||||||
|
ORI = OnesReasoningsInitializer
|
||||||
|
PPRI = PurePositiveReasoningsInitializer
|
||||||
|
RRI = RandomReasoningsInitializer
|
||||||
|
ZRI = ZerosReasoningsInitializer
|
||||||
|
|
||||||
|
# Aliases - Transforms
|
||||||
|
ELTI = Eye = EyeLinearTransformInitializer
|
||||||
|
OLTI = OnesLinearTransformInitializer
|
||||||
|
RLTI = RandomLinearTransformInitializer
|
||||||
|
ZLTI = ZerosLinearTransformInitializer
|
||||||
|
PCALTI = PCALinearTransformInitializer
|
||||||
|
LLTI = LiteralLinearTransformInitializer
|
184
prototorch/core/losses.py
Normal file
184
prototorch/core/losses.py
Normal file
@ -0,0 +1,184 @@
|
|||||||
|
"""ProtoTorch losses"""
|
||||||
|
|
||||||
|
import torch
|
||||||
|
|
||||||
|
from prototorch.nn.activations import get_activation
|
||||||
|
|
||||||
|
|
||||||
|
# Helpers
|
||||||
|
def _get_matcher(targets, labels):
|
||||||
|
"""Returns a boolean tensor."""
|
||||||
|
matcher = torch.eq(targets.unsqueeze(dim=1), labels)
|
||||||
|
if labels.ndim == 2:
|
||||||
|
# if the labels are one-hot vectors
|
||||||
|
num_classes = targets.size()[1]
|
||||||
|
matcher = torch.eq(torch.sum(matcher, dim=-1), num_classes)
|
||||||
|
return matcher
|
||||||
|
|
||||||
|
|
||||||
|
def _get_dp_dm(distances, targets, plabels, with_indices=False):
|
||||||
|
"""Returns the d+ and d- values for a batch of distances."""
|
||||||
|
matcher = _get_matcher(targets, plabels)
|
||||||
|
not_matcher = torch.bitwise_not(matcher)
|
||||||
|
|
||||||
|
inf = torch.full_like(distances, fill_value=float("inf"))
|
||||||
|
d_matching = torch.where(matcher, distances, inf)
|
||||||
|
d_unmatching = torch.where(not_matcher, distances, inf)
|
||||||
|
dp = torch.min(d_matching, dim=-1, keepdim=True)
|
||||||
|
dm = torch.min(d_unmatching, dim=-1, keepdim=True)
|
||||||
|
if with_indices:
|
||||||
|
return dp, dm
|
||||||
|
return dp.values, dm.values
|
||||||
|
|
||||||
|
|
||||||
|
# GLVQ
|
||||||
|
def glvq_loss(distances, target_labels, prototype_labels):
|
||||||
|
"""GLVQ loss function with support for one-hot labels."""
|
||||||
|
dp, dm = _get_dp_dm(distances, target_labels, prototype_labels)
|
||||||
|
mu = (dp - dm) / (dp + dm)
|
||||||
|
return mu
|
||||||
|
|
||||||
|
|
||||||
|
def lvq1_loss(distances, target_labels, prototype_labels):
|
||||||
|
"""LVQ1 loss function with support for one-hot labels.
|
||||||
|
|
||||||
|
See Section 4 [Sado&Yamada]
|
||||||
|
https://papers.nips.cc/paper/1995/file/9c3b1830513cc3b8fc4b76635d32e692-Paper.pdf
|
||||||
|
"""
|
||||||
|
dp, dm = _get_dp_dm(distances, target_labels, prototype_labels)
|
||||||
|
mu = dp
|
||||||
|
mu[dp > dm] = -dm[dp > dm]
|
||||||
|
return mu
|
||||||
|
|
||||||
|
|
||||||
|
def lvq21_loss(distances, target_labels, prototype_labels):
|
||||||
|
"""LVQ2.1 loss function with support for one-hot labels.
|
||||||
|
|
||||||
|
See Section 4 [Sado&Yamada]
|
||||||
|
https://papers.nips.cc/paper/1995/file/9c3b1830513cc3b8fc4b76635d32e692-Paper.pdf
|
||||||
|
"""
|
||||||
|
dp, dm = _get_dp_dm(distances, target_labels, prototype_labels)
|
||||||
|
mu = dp - dm
|
||||||
|
|
||||||
|
return mu
|
||||||
|
|
||||||
|
|
||||||
|
# Probabilistic
|
||||||
|
def _get_class_probabilities(probabilities, targets, prototype_labels):
|
||||||
|
# Create Label Mapping
|
||||||
|
uniques = prototype_labels.unique(sorted=True).tolist()
|
||||||
|
key_val = {key: val for key, val in zip(uniques, range(len(uniques)))}
|
||||||
|
|
||||||
|
target_indices = torch.LongTensor(list(map(key_val.get, targets.tolist())))
|
||||||
|
|
||||||
|
whole = probabilities.sum(dim=1)
|
||||||
|
correct = probabilities[torch.arange(len(probabilities)), target_indices]
|
||||||
|
wrong = whole - correct
|
||||||
|
|
||||||
|
return whole, correct, wrong
|
||||||
|
|
||||||
|
|
||||||
|
def nllr_loss(probabilities, targets, prototype_labels):
|
||||||
|
"""Compute the Negative Log-Likelihood Ratio loss."""
|
||||||
|
_, correct, wrong = _get_class_probabilities(probabilities, targets,
|
||||||
|
prototype_labels)
|
||||||
|
|
||||||
|
likelihood = correct / wrong
|
||||||
|
log_likelihood = torch.log(likelihood)
|
||||||
|
return -1.0 * log_likelihood
|
||||||
|
|
||||||
|
|
||||||
|
def rslvq_loss(probabilities, targets, prototype_labels):
|
||||||
|
"""Compute the Robust Soft Learning Vector Quantization (RSLVQ) loss."""
|
||||||
|
whole, correct, _ = _get_class_probabilities(probabilities, targets,
|
||||||
|
prototype_labels)
|
||||||
|
|
||||||
|
likelihood = correct / whole
|
||||||
|
log_likelihood = torch.log(likelihood)
|
||||||
|
return -1.0 * log_likelihood
|
||||||
|
|
||||||
|
|
||||||
|
def margin_loss(y_pred, y_true, margin=0.3):
|
||||||
|
"""Compute the margin loss."""
|
||||||
|
dp = torch.sum(y_true * y_pred, dim=-1)
|
||||||
|
dm = torch.max(y_pred - y_true, dim=-1).values
|
||||||
|
return torch.nn.functional.relu(dm - dp + margin)
|
||||||
|
|
||||||
|
|
||||||
|
class GLVQLoss(torch.nn.Module):
|
||||||
|
|
||||||
|
def __init__(self,
|
||||||
|
margin=0.0,
|
||||||
|
transfer_fn="identity",
|
||||||
|
beta=10,
|
||||||
|
add_dp=False,
|
||||||
|
**kwargs):
|
||||||
|
super().__init__(**kwargs)
|
||||||
|
self.margin = margin
|
||||||
|
self.transfer_fn = get_activation(transfer_fn)
|
||||||
|
self.beta = torch.tensor(beta)
|
||||||
|
self.add_dp = add_dp
|
||||||
|
|
||||||
|
def forward(self, outputs, targets, plabels):
|
||||||
|
# mu = glvq_loss(outputs, targets, plabels)
|
||||||
|
dp, dm = _get_dp_dm(outputs, targets, plabels)
|
||||||
|
mu = (dp - dm) / (dp + dm)
|
||||||
|
if self.add_dp:
|
||||||
|
mu = mu + dp
|
||||||
|
batch_loss = self.transfer_fn(mu + self.margin, beta=self.beta)
|
||||||
|
return batch_loss.sum()
|
||||||
|
|
||||||
|
|
||||||
|
class MarginLoss(torch.nn.modules.loss._Loss):
|
||||||
|
|
||||||
|
def __init__(self,
|
||||||
|
margin=0.3,
|
||||||
|
size_average=None,
|
||||||
|
reduce=None,
|
||||||
|
reduction="mean"):
|
||||||
|
super().__init__(size_average, reduce, reduction)
|
||||||
|
self.margin = margin
|
||||||
|
|
||||||
|
def forward(self, y_pred, y_true):
|
||||||
|
return margin_loss(y_pred, y_true, self.margin)
|
||||||
|
|
||||||
|
|
||||||
|
class NeuralGasEnergy(torch.nn.Module):
|
||||||
|
|
||||||
|
def __init__(self, lm, **kwargs):
|
||||||
|
super().__init__(**kwargs)
|
||||||
|
self.lm = lm
|
||||||
|
|
||||||
|
def forward(self, d):
|
||||||
|
order = torch.argsort(d, dim=1)
|
||||||
|
ranks = torch.argsort(order, dim=1)
|
||||||
|
cost = torch.sum(self._nghood_fn(ranks, self.lm) * d)
|
||||||
|
|
||||||
|
return cost, order
|
||||||
|
|
||||||
|
def extra_repr(self):
|
||||||
|
return f"lambda: {self.lm}"
|
||||||
|
|
||||||
|
@staticmethod
|
||||||
|
def _nghood_fn(rankings, lm):
|
||||||
|
return torch.exp(-rankings / lm)
|
||||||
|
|
||||||
|
|
||||||
|
class GrowingNeuralGasEnergy(NeuralGasEnergy):
|
||||||
|
|
||||||
|
def __init__(self, topology_layer, **kwargs):
|
||||||
|
super().__init__(**kwargs)
|
||||||
|
self.topology_layer = topology_layer
|
||||||
|
|
||||||
|
@staticmethod
|
||||||
|
def _nghood_fn(rankings, topology):
|
||||||
|
winner = rankings[:, 0]
|
||||||
|
|
||||||
|
weights = torch.zeros_like(rankings, dtype=torch.float)
|
||||||
|
weights[torch.arange(rankings.shape[0]), winner] = 1.0
|
||||||
|
|
||||||
|
neighbours = topology.get_neighbours(winner)
|
||||||
|
|
||||||
|
weights[neighbours] = 0.1
|
||||||
|
|
||||||
|
return weights
|
108
prototorch/core/pooling.py
Normal file
108
prototorch/core/pooling.py
Normal file
@ -0,0 +1,108 @@
|
|||||||
|
"""ProtoTorch pooling"""
|
||||||
|
|
||||||
|
from typing import Callable
|
||||||
|
|
||||||
|
import torch
|
||||||
|
|
||||||
|
|
||||||
|
def stratify_with(values: torch.Tensor,
|
||||||
|
labels: torch.LongTensor,
|
||||||
|
fn: Callable,
|
||||||
|
fill_value: float = 0.0) -> (torch.Tensor):
|
||||||
|
"""Apply an arbitrary stratification strategy on the columns on `values`.
|
||||||
|
|
||||||
|
The outputs correspond to sorted labels.
|
||||||
|
"""
|
||||||
|
clabels = torch.unique(labels, dim=0, sorted=True)
|
||||||
|
num_classes = clabels.size()[0]
|
||||||
|
if values.size()[1] == num_classes:
|
||||||
|
# skip if stratification is trivial
|
||||||
|
return values
|
||||||
|
batch_size = values.size()[0]
|
||||||
|
winning_values = torch.zeros(num_classes, batch_size, device=labels.device)
|
||||||
|
filler = torch.full_like(values.T, fill_value=fill_value)
|
||||||
|
for i, cl in enumerate(clabels):
|
||||||
|
matcher = torch.eq(labels.unsqueeze(dim=1), cl)
|
||||||
|
if labels.ndim == 2:
|
||||||
|
# if the labels are one-hot vectors
|
||||||
|
matcher = torch.eq(torch.sum(matcher, dim=-1), num_classes)
|
||||||
|
cdists = torch.where(matcher, values.T, filler).T
|
||||||
|
winning_values[i] = fn(cdists)
|
||||||
|
if labels.ndim == 2:
|
||||||
|
# Transpose to return with `batch_size` first and
|
||||||
|
# reverse the columns to fix the ordering of the classes
|
||||||
|
return torch.flip(winning_values.T, dims=(1, ))
|
||||||
|
|
||||||
|
return winning_values.T # return with `batch_size` first
|
||||||
|
|
||||||
|
|
||||||
|
def stratified_sum_pooling(values: torch.Tensor,
|
||||||
|
labels: torch.LongTensor) -> (torch.Tensor):
|
||||||
|
"""Group-wise sum."""
|
||||||
|
winning_values = stratify_with(
|
||||||
|
values,
|
||||||
|
labels,
|
||||||
|
fn=lambda x: torch.sum(x, dim=1, keepdim=True).squeeze(),
|
||||||
|
fill_value=0.0)
|
||||||
|
return winning_values
|
||||||
|
|
||||||
|
|
||||||
|
def stratified_min_pooling(values: torch.Tensor,
|
||||||
|
labels: torch.LongTensor) -> (torch.Tensor):
|
||||||
|
"""Group-wise minimum."""
|
||||||
|
winning_values = stratify_with(
|
||||||
|
values,
|
||||||
|
labels,
|
||||||
|
fn=lambda x: torch.min(x, dim=1, keepdim=True).values.squeeze(),
|
||||||
|
fill_value=float("inf"))
|
||||||
|
return winning_values
|
||||||
|
|
||||||
|
|
||||||
|
def stratified_max_pooling(values: torch.Tensor,
|
||||||
|
labels: torch.LongTensor) -> (torch.Tensor):
|
||||||
|
"""Group-wise maximum."""
|
||||||
|
winning_values = stratify_with(
|
||||||
|
values,
|
||||||
|
labels,
|
||||||
|
fn=lambda x: torch.max(x, dim=1, keepdim=True).values.squeeze(),
|
||||||
|
fill_value=-1.0 * float("inf"))
|
||||||
|
return winning_values
|
||||||
|
|
||||||
|
|
||||||
|
def stratified_prod_pooling(values: torch.Tensor,
|
||||||
|
labels: torch.LongTensor) -> (torch.Tensor):
|
||||||
|
"""Group-wise maximum."""
|
||||||
|
winning_values = stratify_with(
|
||||||
|
values,
|
||||||
|
labels,
|
||||||
|
fn=lambda x: torch.prod(x, dim=1, keepdim=True).squeeze(),
|
||||||
|
fill_value=1.0)
|
||||||
|
return winning_values
|
||||||
|
|
||||||
|
|
||||||
|
class StratifiedSumPooling(torch.nn.Module):
|
||||||
|
"""Thin wrapper over the `stratified_sum_pooling` function."""
|
||||||
|
|
||||||
|
def forward(self, values, labels): # pylint: disable=no-self-use
|
||||||
|
return stratified_sum_pooling(values, labels)
|
||||||
|
|
||||||
|
|
||||||
|
class StratifiedProdPooling(torch.nn.Module):
|
||||||
|
"""Thin wrapper over the `stratified_prod_pooling` function."""
|
||||||
|
|
||||||
|
def forward(self, values, labels): # pylint: disable=no-self-use
|
||||||
|
return stratified_prod_pooling(values, labels)
|
||||||
|
|
||||||
|
|
||||||
|
class StratifiedMinPooling(torch.nn.Module):
|
||||||
|
"""Thin wrapper over the `stratified_min_pooling` function."""
|
||||||
|
|
||||||
|
def forward(self, values, labels): # pylint: disable=no-self-use
|
||||||
|
return stratified_min_pooling(values, labels)
|
||||||
|
|
||||||
|
|
||||||
|
class StratifiedMaxPooling(torch.nn.Module):
|
||||||
|
"""Thin wrapper over the `stratified_max_pooling` function."""
|
||||||
|
|
||||||
|
def forward(self, values, labels): # pylint: disable=no-self-use
|
||||||
|
return stratified_max_pooling(values, labels)
|
@ -1,7 +1,19 @@
|
|||||||
"""ProtoTorch similarity functions."""
|
"""ProtoTorch similarities."""
|
||||||
|
|
||||||
import torch
|
import torch
|
||||||
|
|
||||||
|
from .distances import euclidean_distance
|
||||||
|
|
||||||
|
|
||||||
|
def gaussian(x, variance=1.0):
|
||||||
|
return torch.exp(-(x * x) / (2 * variance))
|
||||||
|
|
||||||
|
|
||||||
|
def euclidean_similarity(x, y, variance=1.0):
|
||||||
|
distances = euclidean_distance(x, y)
|
||||||
|
similarities = gaussian(distances, variance)
|
||||||
|
return similarities
|
||||||
|
|
||||||
|
|
||||||
def cosine_similarity(x, y):
|
def cosine_similarity(x, y):
|
||||||
"""Compute the cosine similarity between :math:`x` and :math:`y`.
|
"""Compute the cosine similarity between :math:`x` and :math:`y`.
|
||||||
@ -9,6 +21,7 @@ def cosine_similarity(x, y):
|
|||||||
Expected dimension of x is 2.
|
Expected dimension of x is 2.
|
||||||
Expected dimension of y is 2.
|
Expected dimension of y is 2.
|
||||||
"""
|
"""
|
||||||
|
x, y = (arr.view(arr.size(0), -1) for arr in (x, y))
|
||||||
norm_x = x.pow(2).sum(1).sqrt()
|
norm_x = x.pow(2).sum(1).sqrt()
|
||||||
norm_y = y.pow(2).sum(1).sqrt()
|
norm_y = y.pow(2).sum(1).sqrt()
|
||||||
norm_mat = norm_x.unsqueeze(-1) @ norm_y.unsqueeze(-1).T
|
norm_mat = norm_x.unsqueeze(-1) @ norm_y.unsqueeze(-1).T
|
47
prototorch/core/transforms.py
Normal file
47
prototorch/core/transforms.py
Normal file
@ -0,0 +1,47 @@
|
|||||||
|
"""ProtoTorch transforms"""
|
||||||
|
|
||||||
|
import torch
|
||||||
|
from torch.nn.parameter import Parameter
|
||||||
|
|
||||||
|
from .initializers import (
|
||||||
|
AbstractLinearTransformInitializer,
|
||||||
|
EyeLinearTransformInitializer,
|
||||||
|
)
|
||||||
|
|
||||||
|
|
||||||
|
class LinearTransform(torch.nn.Module):
|
||||||
|
|
||||||
|
def __init__(
|
||||||
|
self,
|
||||||
|
in_dim: int,
|
||||||
|
out_dim: int,
|
||||||
|
initializer:
|
||||||
|
AbstractLinearTransformInitializer = EyeLinearTransformInitializer()):
|
||||||
|
super().__init__()
|
||||||
|
self.set_weights(in_dim, out_dim, initializer)
|
||||||
|
|
||||||
|
@property
|
||||||
|
def weights(self):
|
||||||
|
return self._weights.detach().cpu()
|
||||||
|
|
||||||
|
def _register_weights(self, weights):
|
||||||
|
self.register_parameter("_weights", Parameter(weights))
|
||||||
|
|
||||||
|
def set_weights(
|
||||||
|
self,
|
||||||
|
in_dim: int,
|
||||||
|
out_dim: int,
|
||||||
|
initializer:
|
||||||
|
AbstractLinearTransformInitializer = EyeLinearTransformInitializer()):
|
||||||
|
weights = initializer.generate(in_dim, out_dim)
|
||||||
|
self._register_weights(weights)
|
||||||
|
|
||||||
|
def forward(self, x):
|
||||||
|
return x @ self._weights
|
||||||
|
|
||||||
|
def extra_repr(self):
|
||||||
|
return f"weights: (shape: {tuple(self._weights.shape)})"
|
||||||
|
|
||||||
|
|
||||||
|
# Aliases
|
||||||
|
Omega = LinearTransform
|
@ -1,11 +1,13 @@
|
|||||||
"""ProtoTorch datasets."""
|
"""ProtoTorch datasets"""
|
||||||
|
|
||||||
from .abstract import NumpyDataset
|
from .abstract import CSVDataset, NumpyDataset
|
||||||
|
from .sklearn import (
|
||||||
|
Blobs,
|
||||||
|
Circles,
|
||||||
|
Iris,
|
||||||
|
Moons,
|
||||||
|
Random,
|
||||||
|
)
|
||||||
from .spiral import Spiral
|
from .spiral import Spiral
|
||||||
from .tecator import Tecator
|
from .tecator import Tecator
|
||||||
|
from .xor import XOR
|
||||||
__all__ = [
|
|
||||||
"NumpyDataset",
|
|
||||||
"Spiral",
|
|
||||||
"Tecator",
|
|
||||||
]
|
|
||||||
|
@ -1,31 +1,26 @@
|
|||||||
"""ProtoTorch abstract dataset classes.
|
"""ProtoTorch abstract dataset classes
|
||||||
|
|
||||||
Based on `torchvision.VisionDataset` and `torchvision.MNIST`
|
Based on `torchvision.VisionDataset` and `torchvision.MNIST`.
|
||||||
|
|
||||||
For the original code, see:
|
For the original code, see:
|
||||||
https://github.com/pytorch/vision/blob/master/torchvision/datasets/vision.py
|
https://github.com/pytorch/vision/blob/master/torchvision/datasets/vision.py
|
||||||
https://github.com/pytorch/vision/blob/master/torchvision/datasets/mnist.py
|
https://github.com/pytorch/vision/blob/master/torchvision/datasets/mnist.py
|
||||||
|
|
||||||
"""
|
"""
|
||||||
|
|
||||||
import os
|
import os
|
||||||
|
|
||||||
|
import numpy as np
|
||||||
import torch
|
import torch
|
||||||
|
|
||||||
|
|
||||||
class NumpyDataset(torch.utils.data.TensorDataset):
|
|
||||||
"""Create a PyTorch TensorDataset from NumPy arrays."""
|
|
||||||
def __init__(self, *arrays):
|
|
||||||
tensors = [torch.Tensor(arr) for arr in arrays]
|
|
||||||
super().__init__(*tensors)
|
|
||||||
|
|
||||||
|
|
||||||
class Dataset(torch.utils.data.Dataset):
|
class Dataset(torch.utils.data.Dataset):
|
||||||
"""Abstract dataset class to be inherited."""
|
"""Abstract dataset class to be inherited."""
|
||||||
|
|
||||||
_repr_indent = 2
|
_repr_indent = 2
|
||||||
|
|
||||||
def __init__(self, root):
|
def __init__(self, root):
|
||||||
if isinstance(root, torch._six.string_classes):
|
if isinstance(root, str):
|
||||||
root = os.path.expanduser(root)
|
root = os.path.expanduser(root)
|
||||||
self.root = root
|
self.root = root
|
||||||
|
|
||||||
@ -42,7 +37,7 @@ class ProtoDataset(Dataset):
|
|||||||
training_file = "training.pt"
|
training_file = "training.pt"
|
||||||
test_file = "test.pt"
|
test_file = "test.pt"
|
||||||
|
|
||||||
def __init__(self, root, train=True, download=True, verbose=True):
|
def __init__(self, root="", train=True, download=True, verbose=True):
|
||||||
super().__init__(root)
|
super().__init__(root)
|
||||||
self.train = train # training set or test set
|
self.train = train # training set or test set
|
||||||
self.verbose = verbose
|
self.verbose = verbose
|
||||||
@ -94,3 +89,27 @@ class ProtoDataset(Dataset):
|
|||||||
|
|
||||||
def _download(self):
|
def _download(self):
|
||||||
raise NotImplementedError
|
raise NotImplementedError
|
||||||
|
|
||||||
|
|
||||||
|
class NumpyDataset(torch.utils.data.TensorDataset):
|
||||||
|
"""Create a PyTorch TensorDataset from NumPy arrays."""
|
||||||
|
|
||||||
|
def __init__(self, data, targets):
|
||||||
|
self.data = torch.Tensor(data)
|
||||||
|
self.targets = torch.LongTensor(targets)
|
||||||
|
tensors = [self.data, self.targets]
|
||||||
|
super().__init__(*tensors)
|
||||||
|
|
||||||
|
|
||||||
|
class CSVDataset(NumpyDataset):
|
||||||
|
"""Create a Dataset from a CSV file."""
|
||||||
|
|
||||||
|
def __init__(self, filepath, target_col=-1, delimiter=',', skip_header=0):
|
||||||
|
raw = np.genfromtxt(
|
||||||
|
filepath,
|
||||||
|
delimiter=delimiter,
|
||||||
|
skip_header=skip_header,
|
||||||
|
)
|
||||||
|
data = np.delete(raw, 1, target_col)
|
||||||
|
targets = raw[:, target_col]
|
||||||
|
super().__init__(data, targets)
|
||||||
|
165
prototorch/datasets/sklearn.py
Normal file
165
prototorch/datasets/sklearn.py
Normal file
@ -0,0 +1,165 @@
|
|||||||
|
"""Thin wrappers for a few scikit-learn datasets.
|
||||||
|
|
||||||
|
URL:
|
||||||
|
https://scikit-learn.org/stable/modules/classes.html#module-sklearn.datasets
|
||||||
|
|
||||||
|
"""
|
||||||
|
|
||||||
|
from __future__ import annotations
|
||||||
|
|
||||||
|
import warnings
|
||||||
|
from typing import Sequence
|
||||||
|
|
||||||
|
from sklearn.datasets import (
|
||||||
|
load_iris,
|
||||||
|
make_blobs,
|
||||||
|
make_circles,
|
||||||
|
make_classification,
|
||||||
|
make_moons,
|
||||||
|
)
|
||||||
|
|
||||||
|
from prototorch.datasets.abstract import NumpyDataset
|
||||||
|
|
||||||
|
|
||||||
|
class Iris(NumpyDataset):
|
||||||
|
"""Iris Dataset by Ronald Fisher introduced in 1936.
|
||||||
|
|
||||||
|
The dataset contains four measurements from flowers of three species of iris.
|
||||||
|
|
||||||
|
.. list-table:: Iris
|
||||||
|
:header-rows: 1
|
||||||
|
|
||||||
|
* - dimensions
|
||||||
|
- classes
|
||||||
|
- training size
|
||||||
|
- validation size
|
||||||
|
- test size
|
||||||
|
* - 4
|
||||||
|
- 3
|
||||||
|
- 150
|
||||||
|
- 0
|
||||||
|
- 0
|
||||||
|
|
||||||
|
:param dims: select a subset of dimensions
|
||||||
|
"""
|
||||||
|
|
||||||
|
def __init__(self, dims: Sequence[int] | None = None):
|
||||||
|
x, y = load_iris(return_X_y=True)
|
||||||
|
if dims is not None:
|
||||||
|
x = x[:, dims]
|
||||||
|
super().__init__(x, y)
|
||||||
|
|
||||||
|
|
||||||
|
class Blobs(NumpyDataset):
|
||||||
|
"""Generate isotropic Gaussian blobs for clustering.
|
||||||
|
|
||||||
|
Read more at
|
||||||
|
https://scikit-learn.org/stable/datasets/sample_generators.html#sample-generators.
|
||||||
|
|
||||||
|
"""
|
||||||
|
|
||||||
|
def __init__(
|
||||||
|
self,
|
||||||
|
num_samples: int = 300,
|
||||||
|
num_features: int = 2,
|
||||||
|
seed: None | int = 0,
|
||||||
|
):
|
||||||
|
x, y = make_blobs(
|
||||||
|
num_samples,
|
||||||
|
num_features,
|
||||||
|
centers=None,
|
||||||
|
random_state=seed,
|
||||||
|
shuffle=False,
|
||||||
|
)
|
||||||
|
super().__init__(x, y)
|
||||||
|
|
||||||
|
|
||||||
|
class Random(NumpyDataset):
|
||||||
|
"""Generate a random n-class classification problem.
|
||||||
|
|
||||||
|
Read more at
|
||||||
|
https://scikit-learn.org/stable/modules/generated/sklearn.datasets.make_classification.html.
|
||||||
|
|
||||||
|
Note: n_classes * n_clusters_per_class <= 2**n_informative must satisfy.
|
||||||
|
"""
|
||||||
|
|
||||||
|
def __init__(
|
||||||
|
self,
|
||||||
|
num_samples: int = 300,
|
||||||
|
num_features: int = 2,
|
||||||
|
num_classes: int = 2,
|
||||||
|
num_clusters: int = 2,
|
||||||
|
num_informative: None | int = None,
|
||||||
|
separation: float = 1.0,
|
||||||
|
seed: None | int = 0,
|
||||||
|
):
|
||||||
|
if not num_informative:
|
||||||
|
import math
|
||||||
|
num_informative = math.ceil(math.log2(num_classes * num_clusters))
|
||||||
|
if num_features < num_informative:
|
||||||
|
warnings.warn("Generating more features than requested.")
|
||||||
|
num_features = num_informative
|
||||||
|
x, y = make_classification(
|
||||||
|
num_samples,
|
||||||
|
num_features,
|
||||||
|
n_informative=num_informative,
|
||||||
|
n_redundant=0,
|
||||||
|
n_classes=num_classes,
|
||||||
|
n_clusters_per_class=num_clusters,
|
||||||
|
class_sep=separation,
|
||||||
|
random_state=seed,
|
||||||
|
shuffle=False,
|
||||||
|
)
|
||||||
|
super().__init__(x, y)
|
||||||
|
|
||||||
|
|
||||||
|
class Circles(NumpyDataset):
|
||||||
|
"""Make a large circle containing a smaller circle in 2D.
|
||||||
|
|
||||||
|
A simple toy dataset to visualize clustering and classification algorithms.
|
||||||
|
|
||||||
|
Read more at
|
||||||
|
https://scikit-learn.org/stable/modules/generated/sklearn.datasets.make_circles.html
|
||||||
|
|
||||||
|
"""
|
||||||
|
|
||||||
|
def __init__(
|
||||||
|
self,
|
||||||
|
num_samples: int = 300,
|
||||||
|
noise: float = 0.3,
|
||||||
|
factor: float = 0.8,
|
||||||
|
seed: None | int = 0,
|
||||||
|
):
|
||||||
|
x, y = make_circles(
|
||||||
|
num_samples,
|
||||||
|
noise=noise,
|
||||||
|
factor=factor,
|
||||||
|
random_state=seed,
|
||||||
|
shuffle=False,
|
||||||
|
)
|
||||||
|
super().__init__(x, y)
|
||||||
|
|
||||||
|
|
||||||
|
class Moons(NumpyDataset):
|
||||||
|
"""Make two interleaving half circles.
|
||||||
|
|
||||||
|
A simple toy dataset to visualize clustering and classification algorithms.
|
||||||
|
|
||||||
|
Read more at
|
||||||
|
https://scikit-learn.org/stable/modules/generated/sklearn.datasets.make_moons.html
|
||||||
|
|
||||||
|
"""
|
||||||
|
|
||||||
|
def __init__(
|
||||||
|
self,
|
||||||
|
num_samples: int = 300,
|
||||||
|
noise: float = 0.3,
|
||||||
|
seed: None | int = 0,
|
||||||
|
):
|
||||||
|
x, y = make_moons(
|
||||||
|
num_samples,
|
||||||
|
noise=noise,
|
||||||
|
random_state=seed,
|
||||||
|
shuffle=False,
|
||||||
|
)
|
||||||
|
super().__init__(x, y)
|
@ -4,18 +4,23 @@ import numpy as np
|
|||||||
import torch
|
import torch
|
||||||
|
|
||||||
|
|
||||||
def make_spiral(n_samples=500, noise=0.3):
|
def make_spiral(num_samples=500, noise=0.3):
|
||||||
|
"""Generates the Spiral Dataset.
|
||||||
|
|
||||||
|
For use in Prototorch use `prototorch.datasets.Spiral` instead.
|
||||||
|
"""
|
||||||
|
|
||||||
def get_samples(n, delta_t):
|
def get_samples(n, delta_t):
|
||||||
points = []
|
points = []
|
||||||
for i in range(n):
|
for i in range(n):
|
||||||
r = i / n_samples * 5
|
r = i / num_samples * 5
|
||||||
t = 1.75 * i / n * 2 * np.pi + delta_t
|
t = 1.75 * i / n * 2 * np.pi + delta_t
|
||||||
x = r * np.sin(t) + np.random.rand(1) * noise
|
x = r * np.sin(t) + np.random.rand(1) * noise
|
||||||
y = r * np.cos(t) + np.random.rand(1) * noise
|
y = r * np.cos(t) + np.random.rand(1) * noise
|
||||||
points.append([x, y])
|
points.append([x, y])
|
||||||
return points
|
return points
|
||||||
|
|
||||||
n = n_samples // 2
|
n = num_samples // 2
|
||||||
positive = get_samples(n=n, delta_t=0)
|
positive = get_samples(n=n, delta_t=0)
|
||||||
negative = get_samples(n=n, delta_t=np.pi)
|
negative = get_samples(n=n, delta_t=np.pi)
|
||||||
x = np.concatenate(
|
x = np.concatenate(
|
||||||
@ -27,7 +32,28 @@ def make_spiral(n_samples=500, noise=0.3):
|
|||||||
|
|
||||||
|
|
||||||
class Spiral(torch.utils.data.TensorDataset):
|
class Spiral(torch.utils.data.TensorDataset):
|
||||||
"""Spiral dataset for binary classification."""
|
"""Spiral dataset for binary classification.
|
||||||
def __init__(self, n_samples=500, noise=0.3):
|
|
||||||
x, y = make_spiral(n_samples, noise)
|
This datasets consists of two spirals of two different classes.
|
||||||
|
|
||||||
|
.. list-table:: Spiral
|
||||||
|
:header-rows: 1
|
||||||
|
|
||||||
|
* - dimensions
|
||||||
|
- classes
|
||||||
|
- training size
|
||||||
|
- validation size
|
||||||
|
- test size
|
||||||
|
* - 2
|
||||||
|
- 2
|
||||||
|
- num_samples
|
||||||
|
- 0
|
||||||
|
- 0
|
||||||
|
|
||||||
|
:param num_samples: number of random samples
|
||||||
|
:param noise: noise added to the spirals
|
||||||
|
"""
|
||||||
|
|
||||||
|
def __init__(self, num_samples: int = 500, noise: float = 0.3):
|
||||||
|
x, y = make_spiral(num_samples, noise)
|
||||||
super().__init__(torch.Tensor(x), torch.LongTensor(y))
|
super().__init__(torch.Tensor(x), torch.LongTensor(y))
|
||||||
|
@ -36,6 +36,7 @@ Description:
|
|||||||
are determined by analytic chemistry.
|
are determined by analytic chemistry.
|
||||||
"""
|
"""
|
||||||
|
|
||||||
|
import logging
|
||||||
import os
|
import os
|
||||||
|
|
||||||
import numpy as np
|
import numpy as np
|
||||||
@ -47,8 +48,23 @@ from prototorch.datasets.abstract import ProtoDataset
|
|||||||
|
|
||||||
class Tecator(ProtoDataset):
|
class Tecator(ProtoDataset):
|
||||||
"""
|
"""
|
||||||
`Tecator Dataset <http://lib.stat.cmu.edu/datasets/tecator>`__
|
`Tecator Dataset <http://lib.stat.cmu.edu/datasets/tecator>`__ for classification.
|
||||||
for classification.
|
|
||||||
|
The dataset contains wavelength measurements of meat.
|
||||||
|
|
||||||
|
.. list-table:: Tecator
|
||||||
|
:header-rows: 1
|
||||||
|
|
||||||
|
* - dimensions
|
||||||
|
- classes
|
||||||
|
- training size
|
||||||
|
- validation size
|
||||||
|
- test size
|
||||||
|
* - 100
|
||||||
|
- 2
|
||||||
|
- 129
|
||||||
|
- 43
|
||||||
|
- 43
|
||||||
"""
|
"""
|
||||||
|
|
||||||
_resources = [
|
_resources = [
|
||||||
@ -66,13 +82,11 @@ class Tecator(ProtoDataset):
|
|||||||
if self._check_exists():
|
if self._check_exists():
|
||||||
return
|
return
|
||||||
|
|
||||||
if self.verbose:
|
logging.debug("Making directories...")
|
||||||
print("Making directories...")
|
|
||||||
os.makedirs(self.raw_folder, exist_ok=True)
|
os.makedirs(self.raw_folder, exist_ok=True)
|
||||||
os.makedirs(self.processed_folder, exist_ok=True)
|
os.makedirs(self.processed_folder, exist_ok=True)
|
||||||
|
|
||||||
if self.verbose:
|
logging.debug("Downloading...")
|
||||||
print("Downloading...")
|
|
||||||
for fileid, md5 in self._resources:
|
for fileid, md5 in self._resources:
|
||||||
filename = "tecator.npz"
|
filename = "tecator.npz"
|
||||||
download_file_from_google_drive(fileid,
|
download_file_from_google_drive(fileid,
|
||||||
@ -80,19 +94,18 @@ class Tecator(ProtoDataset):
|
|||||||
filename=filename,
|
filename=filename,
|
||||||
md5=md5)
|
md5=md5)
|
||||||
|
|
||||||
if self.verbose:
|
logging.debug("Processing...")
|
||||||
print("Processing...")
|
|
||||||
with np.load(os.path.join(self.raw_folder, "tecator.npz"),
|
with np.load(os.path.join(self.raw_folder, "tecator.npz"),
|
||||||
allow_pickle=False) as f:
|
allow_pickle=False) as f:
|
||||||
x_train, y_train = f["x_train"], f["y_train"]
|
x_train, y_train = f["x_train"], f["y_train"]
|
||||||
x_test, y_test = f["x_test"], f["y_test"]
|
x_test, y_test = f["x_test"], f["y_test"]
|
||||||
training_set = [
|
training_set = [
|
||||||
torch.tensor(x_train, dtype=torch.float32),
|
torch.Tensor(x_train),
|
||||||
torch.tensor(y_train),
|
torch.LongTensor(y_train),
|
||||||
]
|
]
|
||||||
test_set = [
|
test_set = [
|
||||||
torch.tensor(x_test, dtype=torch.float32),
|
torch.Tensor(x_test),
|
||||||
torch.tensor(y_test),
|
torch.LongTensor(y_test),
|
||||||
]
|
]
|
||||||
|
|
||||||
with open(os.path.join(self.processed_folder, self.training_file),
|
with open(os.path.join(self.processed_folder, self.training_file),
|
||||||
@ -102,5 +115,4 @@ class Tecator(ProtoDataset):
|
|||||||
"wb") as f:
|
"wb") as f:
|
||||||
torch.save(test_set, f)
|
torch.save(test_set, f)
|
||||||
|
|
||||||
if self.verbose:
|
logging.debug("Done!")
|
||||||
print("Done!")
|
|
||||||
|
19
prototorch/datasets/xor.py
Normal file
19
prototorch/datasets/xor.py
Normal file
@ -0,0 +1,19 @@
|
|||||||
|
"""Exclusive-or (XOR) dataset for binary classification."""
|
||||||
|
|
||||||
|
import torch
|
||||||
|
|
||||||
|
|
||||||
|
def make_xor(num_samples=500):
|
||||||
|
x = torch.rand(num_samples, 2)
|
||||||
|
y = torch.zeros(num_samples)
|
||||||
|
y[torch.logical_and(x[:, 0] > 0.5, x[:, 1] < 0.5)] = 1
|
||||||
|
y[torch.logical_and(x[:, 1] > 0.5, x[:, 0] < 0.5)] = 1
|
||||||
|
return x, y
|
||||||
|
|
||||||
|
|
||||||
|
class XOR(torch.utils.data.TensorDataset):
|
||||||
|
"""Exclusive-or (XOR) dataset for binary classification."""
|
||||||
|
|
||||||
|
def __init__(self, num_samples: int = 500):
|
||||||
|
x, y = make_xor(num_samples)
|
||||||
|
super().__init__(x, y)
|
@ -1,12 +0,0 @@
|
|||||||
"""ProtoTorch functions."""
|
|
||||||
|
|
||||||
from .activations import identity, sigmoid_beta, swish_beta
|
|
||||||
from .competitions import knnc, wtac
|
|
||||||
|
|
||||||
__all__ = [
|
|
||||||
"identity",
|
|
||||||
"sigmoid_beta",
|
|
||||||
"swish_beta",
|
|
||||||
"knnc",
|
|
||||||
"wtac",
|
|
||||||
]
|
|
@ -1,45 +0,0 @@
|
|||||||
"""ProtoTorch competition functions."""
|
|
||||||
|
|
||||||
import torch
|
|
||||||
|
|
||||||
|
|
||||||
# @torch.jit.script
|
|
||||||
def stratified_min(distances, labels):
|
|
||||||
clabels = torch.unique(labels, dim=0)
|
|
||||||
nclasses = clabels.size()[0]
|
|
||||||
if distances.size()[1] == nclasses:
|
|
||||||
# skip if only one prototype per class
|
|
||||||
return distances
|
|
||||||
batch_size = distances.size()[0]
|
|
||||||
winning_distances = torch.zeros(nclasses, batch_size)
|
|
||||||
inf = torch.full_like(distances.T, fill_value=float("inf"))
|
|
||||||
# distances_to_wpluses = torch.where(matcher, distances, inf)
|
|
||||||
for i, cl in enumerate(clabels):
|
|
||||||
# cdists = distances.T[labels == cl]
|
|
||||||
matcher = torch.eq(labels.unsqueeze(dim=1), cl)
|
|
||||||
if labels.ndim == 2:
|
|
||||||
# if the labels are one-hot vectors
|
|
||||||
matcher = torch.eq(torch.sum(matcher, dim=-1), nclasses)
|
|
||||||
cdists = torch.where(matcher, distances.T, inf).T
|
|
||||||
winning_distances[i] = torch.min(cdists, dim=1,
|
|
||||||
keepdim=True).values.squeeze()
|
|
||||||
if labels.ndim == 2:
|
|
||||||
# Transpose to return with `batch_size` first and
|
|
||||||
# reverse the columns to fix the ordering of the classes
|
|
||||||
return torch.flip(winning_distances.T, dims=(1, ))
|
|
||||||
|
|
||||||
return winning_distances.T # return with `batch_size` first
|
|
||||||
|
|
||||||
|
|
||||||
# @torch.jit.script
|
|
||||||
def wtac(distances, labels):
|
|
||||||
winning_indices = torch.min(distances, dim=1).indices
|
|
||||||
winning_labels = labels[winning_indices].squeeze()
|
|
||||||
return winning_labels
|
|
||||||
|
|
||||||
|
|
||||||
# @torch.jit.script
|
|
||||||
def knnc(distances, labels, k):
|
|
||||||
winning_indices = torch.topk(-distances, k=k.item(), dim=1).indices
|
|
||||||
winning_labels = labels[winning_indices].squeeze()
|
|
||||||
return winning_labels
|
|
@ -1,265 +0,0 @@
|
|||||||
"""ProtoTorch distance functions."""
|
|
||||||
|
|
||||||
import numpy as np
|
|
||||||
import torch
|
|
||||||
|
|
||||||
from prototorch.functions.helper import (_check_shapes, _int_and_mixed_shape,
|
|
||||||
equal_int_shape)
|
|
||||||
|
|
||||||
|
|
||||||
def squared_euclidean_distance(x, y):
|
|
||||||
r"""Compute the squared Euclidean distance between :math:`\bm x` and :math:`\bm y`.
|
|
||||||
|
|
||||||
Compute :math:`{\langle \bm x - \bm y \rangle}_2`
|
|
||||||
|
|
||||||
:param `torch.tensor` x: Two dimensional vector
|
|
||||||
:param `torch.tensor` y: Two dimensional vector
|
|
||||||
|
|
||||||
**Alias:**
|
|
||||||
``prototorch.functions.distances.sed``
|
|
||||||
"""
|
|
||||||
expanded_x = x.unsqueeze(dim=1)
|
|
||||||
batchwise_difference = y - expanded_x
|
|
||||||
differences_raised = torch.pow(batchwise_difference, 2)
|
|
||||||
distances = torch.sum(differences_raised, axis=2)
|
|
||||||
return distances
|
|
||||||
|
|
||||||
|
|
||||||
def euclidean_distance(x, y):
|
|
||||||
r"""Compute the Euclidean distance between :math:`x` and :math:`y`.
|
|
||||||
|
|
||||||
Compute :math:`\sqrt{{\langle \bm x - \bm y \rangle}_2}`
|
|
||||||
|
|
||||||
:param `torch.tensor` x: Input Tensor of shape :math:`X \times N`
|
|
||||||
:param `torch.tensor` y: Input Tensor of shape :math:`Y \times N`
|
|
||||||
|
|
||||||
:returns: Distance Tensor of shape :math:`X \times Y`
|
|
||||||
:rtype: `torch.tensor`
|
|
||||||
"""
|
|
||||||
distances_raised = squared_euclidean_distance(x, y)
|
|
||||||
distances = torch.sqrt(distances_raised)
|
|
||||||
return distances
|
|
||||||
|
|
||||||
|
|
||||||
def euclidean_distance_v2(x, y):
|
|
||||||
diff = y - x.unsqueeze(1)
|
|
||||||
pairwise_distances = (diff @ diff.permute((0, 2, 1))).sqrt()
|
|
||||||
# Passing `dim1=-2` and `dim2=-1` to `diagonal()` takes the
|
|
||||||
# batch diagonal. See:
|
|
||||||
# https://pytorch.org/docs/stable/generated/torch.diagonal.html
|
|
||||||
distances = torch.diagonal(pairwise_distances, dim1=-2, dim2=-1)
|
|
||||||
# print(f"{diff.shape=}") # (nx, ny, ndim)
|
|
||||||
# print(f"{pairwise_distances.shape=}") # (nx, ny, ny)
|
|
||||||
# print(f"{distances.shape=}") # (nx, ny)
|
|
||||||
return distances
|
|
||||||
|
|
||||||
|
|
||||||
def lpnorm_distance(x, y, p):
|
|
||||||
r"""Calculate the lp-norm between :math:`\bm x` and :math:`\bm y`.
|
|
||||||
Also known as Minkowski distance.
|
|
||||||
|
|
||||||
Compute :math:`{\| \bm x - \bm y \|}_p`.
|
|
||||||
|
|
||||||
Calls ``torch.cdist``
|
|
||||||
|
|
||||||
:param `torch.tensor` x: Two dimensional vector
|
|
||||||
:param `torch.tensor` y: Two dimensional vector
|
|
||||||
:param p: p parameter of the lp norm
|
|
||||||
"""
|
|
||||||
distances = torch.cdist(x, y, p=p)
|
|
||||||
return distances
|
|
||||||
|
|
||||||
|
|
||||||
def omega_distance(x, y, omega):
|
|
||||||
r"""Omega distance.
|
|
||||||
|
|
||||||
Compute :math:`{\| \Omega \bm x - \Omega \bm y \|}_p`
|
|
||||||
|
|
||||||
:param `torch.tensor` x: Two dimensional vector
|
|
||||||
:param `torch.tensor` y: Two dimensional vector
|
|
||||||
:param `torch.tensor` omega: Two dimensional matrix
|
|
||||||
"""
|
|
||||||
projected_x = x @ omega
|
|
||||||
projected_y = y @ omega
|
|
||||||
distances = squared_euclidean_distance(projected_x, projected_y)
|
|
||||||
return distances
|
|
||||||
|
|
||||||
|
|
||||||
def lomega_distance(x, y, omegas):
|
|
||||||
r"""Localized Omega distance.
|
|
||||||
|
|
||||||
Compute :math:`{\| \Omega_k \bm x - \Omega_k \bm y_k \|}_p`
|
|
||||||
|
|
||||||
:param `torch.tensor` x: Two dimensional vector
|
|
||||||
:param `torch.tensor` y: Two dimensional vector
|
|
||||||
:param `torch.tensor` omegas: Three dimensional matrix
|
|
||||||
"""
|
|
||||||
projected_x = x @ omegas
|
|
||||||
projected_y = torch.diagonal(y @ omegas).T
|
|
||||||
expanded_y = torch.unsqueeze(projected_y, dim=1)
|
|
||||||
batchwise_difference = expanded_y - projected_x
|
|
||||||
differences_squared = batchwise_difference**2
|
|
||||||
distances = torch.sum(differences_squared, dim=2)
|
|
||||||
distances = distances.permute(1, 0)
|
|
||||||
return distances
|
|
||||||
|
|
||||||
|
|
||||||
def euclidean_distance_matrix(x, y, squared=False, epsilon=1e-10):
|
|
||||||
r"""Computes an euclidean distances matrix given two distinct vectors.
|
|
||||||
last dimension must be the vector dimension!
|
|
||||||
compute the distance via the identity of the dot product. This avoids the memory overhead due to the subtraction!
|
|
||||||
|
|
||||||
- ``x.shape = (number_of_x_vectors, vector_dim)``
|
|
||||||
- ``y.shape = (number_of_y_vectors, vector_dim)``
|
|
||||||
|
|
||||||
output: matrix of distances (number_of_x_vectors, number_of_y_vectors)
|
|
||||||
"""
|
|
||||||
for tensor in [x, y]:
|
|
||||||
if tensor.ndim != 2:
|
|
||||||
raise ValueError(
|
|
||||||
"The tensor dimension must be two. You provide: tensor.ndim=" +
|
|
||||||
str(tensor.ndim) + ".")
|
|
||||||
if not equal_int_shape([tuple(x.shape)[1]], [tuple(y.shape)[1]]):
|
|
||||||
raise ValueError(
|
|
||||||
"The vector shape must be equivalent in both tensors. You provide: tuple(y.shape)[1]="
|
|
||||||
+ str(tuple(x.shape)[1]) + " and tuple(y.shape)(y)[1]=" +
|
|
||||||
str(tuple(y.shape)[1]) + ".")
|
|
||||||
|
|
||||||
y = torch.transpose(y)
|
|
||||||
|
|
||||||
diss = (torch.sum(x**2, axis=1, keepdims=True) - 2 * torch.dot(x, y) +
|
|
||||||
torch.sum(y**2, axis=0, keepdims=True))
|
|
||||||
|
|
||||||
if not squared:
|
|
||||||
if epsilon == 0:
|
|
||||||
diss = torch.sqrt(diss)
|
|
||||||
else:
|
|
||||||
diss = torch.sqrt(torch.max(diss, epsilon))
|
|
||||||
|
|
||||||
return diss
|
|
||||||
|
|
||||||
|
|
||||||
def tangent_distance(signals, protos, subspaces, squared=False, epsilon=1e-10):
|
|
||||||
r"""Tangent distances based on the tensorflow implementation of Sascha Saralajews
|
|
||||||
|
|
||||||
For more info about Tangen distances see
|
|
||||||
|
|
||||||
DOI:10.1109/IJCNN.2016.7727534.
|
|
||||||
|
|
||||||
The subspaces is always assumed as transposed and must be orthogonal!
|
|
||||||
For local non sparse signals subspaces must be provided!
|
|
||||||
|
|
||||||
- shape(signals): batch x proto_number x channels x dim1 x dim2 x ... x dimN
|
|
||||||
- shape(protos): proto_number x dim1 x dim2 x ... x dimN
|
|
||||||
- shape(subspaces): (optional [proto_number]) x prod(dim1 * dim2 * ... * dimN) x prod(projected_atom_shape)
|
|
||||||
|
|
||||||
subspace should be orthogonalized
|
|
||||||
Pytorch implementation of Sascha Saralajew's tensorflow code.
|
|
||||||
Translation by Christoph Raab
|
|
||||||
"""
|
|
||||||
signal_shape, signal_int_shape = _int_and_mixed_shape(signals)
|
|
||||||
proto_shape, proto_int_shape = _int_and_mixed_shape(protos)
|
|
||||||
subspace_int_shape = tuple(subspaces.shape)
|
|
||||||
|
|
||||||
# check if the shapes are correct
|
|
||||||
_check_shapes(signal_int_shape, proto_int_shape)
|
|
||||||
|
|
||||||
atom_axes = list(range(3, len(signal_int_shape)))
|
|
||||||
# for sparse signals, we use the memory efficient implementation
|
|
||||||
if signal_int_shape[1] == 1:
|
|
||||||
signals = torch.reshape(signals, [-1, np.prod(signal_shape[3:])])
|
|
||||||
|
|
||||||
if len(atom_axes) > 1:
|
|
||||||
protos = torch.reshape(protos, [proto_shape[0], -1])
|
|
||||||
|
|
||||||
if subspaces.ndim == 2:
|
|
||||||
# clean solution without map if the matrix_scope is global
|
|
||||||
projectors = torch.eye(subspace_int_shape[-2]) - torch.dot(
|
|
||||||
subspaces, torch.transpose(subspaces))
|
|
||||||
|
|
||||||
projected_signals = torch.dot(signals, projectors)
|
|
||||||
projected_protos = torch.dot(protos, projectors)
|
|
||||||
|
|
||||||
diss = euclidean_distance_matrix(projected_signals,
|
|
||||||
projected_protos,
|
|
||||||
squared=squared,
|
|
||||||
epsilon=epsilon)
|
|
||||||
|
|
||||||
diss = torch.reshape(
|
|
||||||
diss, [signal_shape[0], signal_shape[2], proto_shape[0]])
|
|
||||||
|
|
||||||
return torch.permute(diss, [0, 2, 1])
|
|
||||||
|
|
||||||
else:
|
|
||||||
|
|
||||||
# no solution without map possible --> memory efficient but slow!
|
|
||||||
projectors = torch.eye(subspace_int_shape[-2]) - torch.bmm(
|
|
||||||
subspaces,
|
|
||||||
subspaces) # K.batch_dot(subspaces, subspaces, [2, 2])
|
|
||||||
|
|
||||||
projected_protos = (protos @ subspaces
|
|
||||||
).T # K.batch_dot(projectors, protos, [1, 1]))
|
|
||||||
|
|
||||||
def projected_norm(projector):
|
|
||||||
return torch.sum(torch.dot(signals, projector)**2, axis=1)
|
|
||||||
|
|
||||||
diss = (torch.transpose(map(projected_norm, projectors)) -
|
|
||||||
2 * torch.dot(signals, projected_protos) +
|
|
||||||
torch.sum(projected_protos**2, axis=0, keepdims=True))
|
|
||||||
|
|
||||||
if not squared:
|
|
||||||
if epsilon == 0:
|
|
||||||
diss = torch.sqrt(diss)
|
|
||||||
else:
|
|
||||||
diss = torch.sqrt(torch.max(diss, epsilon))
|
|
||||||
|
|
||||||
diss = torch.reshape(
|
|
||||||
diss, [signal_shape[0], signal_shape[2], proto_shape[0]])
|
|
||||||
|
|
||||||
return torch.permute(diss, [0, 2, 1])
|
|
||||||
|
|
||||||
else:
|
|
||||||
signals = signals.permute([0, 2, 1] + atom_axes)
|
|
||||||
|
|
||||||
diff = signals - protos
|
|
||||||
|
|
||||||
# global tangent space
|
|
||||||
if subspaces.ndim == 2:
|
|
||||||
# Scope Projectors
|
|
||||||
projectors = subspaces #
|
|
||||||
|
|
||||||
# Scope: Tangentspace Projections
|
|
||||||
diff = torch.reshape(
|
|
||||||
diff, (signal_shape[0] * signal_shape[2], signal_shape[1], -1))
|
|
||||||
projected_diff = diff @ projectors
|
|
||||||
projected_diff = torch.reshape(
|
|
||||||
projected_diff,
|
|
||||||
(signal_shape[0], signal_shape[2], signal_shape[1]) +
|
|
||||||
signal_shape[3:],
|
|
||||||
)
|
|
||||||
|
|
||||||
diss = torch.norm(projected_diff, 2, dim=-1)
|
|
||||||
return diss.permute([0, 2, 1])
|
|
||||||
|
|
||||||
# local tangent spaces
|
|
||||||
else:
|
|
||||||
# Scope: Calculate Projectors
|
|
||||||
projectors = subspaces
|
|
||||||
|
|
||||||
# Scope: Tangentspace Projections
|
|
||||||
diff = torch.reshape(
|
|
||||||
diff, (signal_shape[0] * signal_shape[2], signal_shape[1], -1))
|
|
||||||
diff = diff.permute([1, 0, 2])
|
|
||||||
projected_diff = torch.bmm(diff, projectors)
|
|
||||||
projected_diff = torch.reshape(
|
|
||||||
projected_diff,
|
|
||||||
(signal_shape[1], signal_shape[0], signal_shape[2]) +
|
|
||||||
signal_shape[3:],
|
|
||||||
)
|
|
||||||
|
|
||||||
diss = torch.norm(projected_diff, 2, dim=-1)
|
|
||||||
return diss.permute([1, 0, 2]).squeeze(-1)
|
|
||||||
|
|
||||||
|
|
||||||
# Aliases
|
|
||||||
sed = squared_euclidean_distance
|
|
@ -1,89 +0,0 @@
|
|||||||
import torch
|
|
||||||
|
|
||||||
|
|
||||||
def calculate_prototype_accuracy(y_pred, y_true, plabels):
|
|
||||||
"""Computes the accuracy of a prototype based model.
|
|
||||||
via Winner-Takes-All rule.
|
|
||||||
Requirement:
|
|
||||||
y_pred.shape == y_true.shape
|
|
||||||
unique(y_pred) in plabels
|
|
||||||
"""
|
|
||||||
with torch.no_grad():
|
|
||||||
idx = torch.argmin(y_pred, axis=1)
|
|
||||||
return torch.true_divide(torch.sum(y_true == plabels[idx]),
|
|
||||||
len(y_pred)) * 100
|
|
||||||
|
|
||||||
|
|
||||||
def predict_label(y_pred, plabels):
|
|
||||||
r""" Predicts labels given a prediction of a prototype based model.
|
|
||||||
"""
|
|
||||||
with torch.no_grad():
|
|
||||||
return plabels[torch.argmin(y_pred, 1)]
|
|
||||||
|
|
||||||
|
|
||||||
def mixed_shape(inputs):
|
|
||||||
if not torch.is_tensor(inputs):
|
|
||||||
raise ValueError("Input must be a tensor.")
|
|
||||||
else:
|
|
||||||
int_shape = list(inputs.shape)
|
|
||||||
# sometimes int_shape returns mixed integer types
|
|
||||||
int_shape = [int(i) if i is not None else i for i in int_shape]
|
|
||||||
tensor_shape = inputs.shape
|
|
||||||
|
|
||||||
for i, s in enumerate(int_shape):
|
|
||||||
if s is None:
|
|
||||||
int_shape[i] = tensor_shape[i]
|
|
||||||
return tuple(int_shape)
|
|
||||||
|
|
||||||
|
|
||||||
def equal_int_shape(shape_1, shape_2):
|
|
||||||
if not isinstance(shape_1,
|
|
||||||
(tuple, list)) or not isinstance(shape_2, (tuple, list)):
|
|
||||||
raise ValueError("Input shapes must list or tuple.")
|
|
||||||
for shape in [shape_1, shape_2]:
|
|
||||||
if not all([isinstance(x, int) or x is None for x in shape]):
|
|
||||||
raise ValueError(
|
|
||||||
"Input shapes must be list or tuple of int and None values.")
|
|
||||||
|
|
||||||
if len(shape_1) != len(shape_2):
|
|
||||||
return False
|
|
||||||
else:
|
|
||||||
for axis, value in enumerate(shape_1):
|
|
||||||
if value is not None and shape_2[axis] not in {value, None}:
|
|
||||||
return False
|
|
||||||
return True
|
|
||||||
|
|
||||||
|
|
||||||
def _check_shapes(signal_int_shape, proto_int_shape):
|
|
||||||
if len(signal_int_shape) < 4:
|
|
||||||
raise ValueError(
|
|
||||||
"The number of signal dimensions must be >=4. You provide: " +
|
|
||||||
str(len(signal_int_shape)))
|
|
||||||
|
|
||||||
if len(proto_int_shape) < 2:
|
|
||||||
raise ValueError(
|
|
||||||
"The number of proto dimensions must be >=2. You provide: " +
|
|
||||||
str(len(proto_int_shape)))
|
|
||||||
|
|
||||||
if not equal_int_shape(signal_int_shape[3:], proto_int_shape[1:]):
|
|
||||||
raise ValueError(
|
|
||||||
"The atom shape of signals must be equal protos. You provide: signals.shape[3:]="
|
|
||||||
+ str(signal_int_shape[3:]) + " != protos.shape[1:]=" +
|
|
||||||
str(proto_int_shape[1:]))
|
|
||||||
|
|
||||||
# not a sparse signal
|
|
||||||
if signal_int_shape[1] != 1:
|
|
||||||
if not equal_int_shape(signal_int_shape[1:2], proto_int_shape[0:1]):
|
|
||||||
raise ValueError(
|
|
||||||
"If the signal is not sparse, the number of prototypes must be equal in signals and "
|
|
||||||
"protos. You provide: " + str(signal_int_shape[1]) + " != " +
|
|
||||||
str(proto_int_shape[0]))
|
|
||||||
|
|
||||||
return True
|
|
||||||
|
|
||||||
|
|
||||||
def _int_and_mixed_shape(tensor):
|
|
||||||
shape = mixed_shape(tensor)
|
|
||||||
int_shape = tuple([i if isinstance(i, int) else None for i in shape])
|
|
||||||
|
|
||||||
return shape, int_shape
|
|
@ -1,107 +0,0 @@
|
|||||||
"""ProtoTorch initialization functions."""
|
|
||||||
|
|
||||||
from itertools import chain
|
|
||||||
|
|
||||||
import torch
|
|
||||||
|
|
||||||
INITIALIZERS = dict()
|
|
||||||
|
|
||||||
|
|
||||||
def register_initializer(function):
|
|
||||||
"""Add the initializer to the registry."""
|
|
||||||
INITIALIZERS[function.__name__] = function
|
|
||||||
return function
|
|
||||||
|
|
||||||
|
|
||||||
def labels_from(distribution, one_hot=True):
|
|
||||||
"""Takes a distribution tensor and returns a labels tensor."""
|
|
||||||
nclasses = distribution.shape[0]
|
|
||||||
llist = [[i] * n for i, n in zip(range(nclasses), distribution)]
|
|
||||||
# labels = [l for cl in llist for l in cl] # flatten the list of lists
|
|
||||||
flat_llist = list(chain(*llist)) # flatten label list with itertools.chain
|
|
||||||
plabels = torch.tensor(flat_llist, requires_grad=False)
|
|
||||||
if one_hot:
|
|
||||||
return torch.eye(nclasses)[plabels]
|
|
||||||
return plabels
|
|
||||||
|
|
||||||
|
|
||||||
@register_initializer
|
|
||||||
def ones(x_train, y_train, prototype_distribution, one_hot=True):
|
|
||||||
nprotos = torch.sum(prototype_distribution)
|
|
||||||
protos = torch.ones(nprotos, *x_train.shape[1:])
|
|
||||||
plabels = labels_from(prototype_distribution, one_hot)
|
|
||||||
return protos, plabels
|
|
||||||
|
|
||||||
|
|
||||||
@register_initializer
|
|
||||||
def zeros(x_train, y_train, prototype_distribution, one_hot=True):
|
|
||||||
nprotos = torch.sum(prototype_distribution)
|
|
||||||
protos = torch.zeros(nprotos, *x_train.shape[1:])
|
|
||||||
plabels = labels_from(prototype_distribution, one_hot)
|
|
||||||
return protos, plabels
|
|
||||||
|
|
||||||
|
|
||||||
@register_initializer
|
|
||||||
def rand(x_train, y_train, prototype_distribution, one_hot=True):
|
|
||||||
nprotos = torch.sum(prototype_distribution)
|
|
||||||
protos = torch.rand(nprotos, *x_train.shape[1:])
|
|
||||||
plabels = labels_from(prototype_distribution, one_hot)
|
|
||||||
return protos, plabels
|
|
||||||
|
|
||||||
|
|
||||||
@register_initializer
|
|
||||||
def randn(x_train, y_train, prototype_distribution, one_hot=True):
|
|
||||||
nprotos = torch.sum(prototype_distribution)
|
|
||||||
protos = torch.randn(nprotos, *x_train.shape[1:])
|
|
||||||
plabels = labels_from(prototype_distribution, one_hot)
|
|
||||||
return protos, plabels
|
|
||||||
|
|
||||||
|
|
||||||
@register_initializer
|
|
||||||
def stratified_mean(x_train, y_train, prototype_distribution, one_hot=True):
|
|
||||||
nprotos = torch.sum(prototype_distribution)
|
|
||||||
pdim = x_train.shape[1]
|
|
||||||
protos = torch.empty(nprotos, pdim)
|
|
||||||
plabels = labels_from(prototype_distribution, one_hot)
|
|
||||||
for i, label in enumerate(plabels):
|
|
||||||
matcher = torch.eq(label.unsqueeze(dim=0), y_train)
|
|
||||||
if one_hot:
|
|
||||||
nclasses = y_train.size()[1]
|
|
||||||
matcher = torch.eq(torch.sum(matcher, dim=-1), nclasses)
|
|
||||||
xl = x_train[matcher]
|
|
||||||
mean_xl = torch.mean(xl, dim=0)
|
|
||||||
protos[i] = mean_xl
|
|
||||||
plabels = labels_from(prototype_distribution, one_hot=one_hot)
|
|
||||||
return protos, plabels
|
|
||||||
|
|
||||||
|
|
||||||
@register_initializer
|
|
||||||
def stratified_random(x_train,
|
|
||||||
y_train,
|
|
||||||
prototype_distribution,
|
|
||||||
one_hot=True,
|
|
||||||
epsilon=1e-7):
|
|
||||||
nprotos = torch.sum(prototype_distribution)
|
|
||||||
pdim = x_train.shape[1]
|
|
||||||
protos = torch.empty(nprotos, pdim)
|
|
||||||
plabels = labels_from(prototype_distribution, one_hot)
|
|
||||||
for i, label in enumerate(plabels):
|
|
||||||
matcher = torch.eq(label.unsqueeze(dim=0), y_train)
|
|
||||||
if one_hot:
|
|
||||||
nclasses = y_train.size()[1]
|
|
||||||
matcher = torch.eq(torch.sum(matcher, dim=-1), nclasses)
|
|
||||||
xl = x_train[matcher]
|
|
||||||
rand_index = torch.zeros(1).long().random_(0, xl.shape[0] - 1)
|
|
||||||
random_xl = xl[rand_index]
|
|
||||||
protos[i] = random_xl + epsilon
|
|
||||||
plabels = labels_from(prototype_distribution, one_hot=one_hot)
|
|
||||||
return protos, plabels
|
|
||||||
|
|
||||||
|
|
||||||
def get_initializer(funcname):
|
|
||||||
"""Deserialize the initializer."""
|
|
||||||
if callable(funcname):
|
|
||||||
return funcname
|
|
||||||
if funcname in INITIALIZERS:
|
|
||||||
return INITIALIZERS.get(funcname)
|
|
||||||
raise NameError(f"Initializer {funcname} was not found.")
|
|
@ -1,56 +0,0 @@
|
|||||||
"""ProtoTorch loss functions."""
|
|
||||||
|
|
||||||
import torch
|
|
||||||
|
|
||||||
|
|
||||||
def _get_matcher(targets, labels):
|
|
||||||
"""Returns a boolean tensor."""
|
|
||||||
matcher = torch.eq(targets.unsqueeze(dim=1), labels)
|
|
||||||
if labels.ndim == 2:
|
|
||||||
# if the labels are one-hot vectors
|
|
||||||
nclasses = targets.size()[1]
|
|
||||||
matcher = torch.eq(torch.sum(matcher, dim=-1), nclasses)
|
|
||||||
return matcher
|
|
||||||
|
|
||||||
|
|
||||||
def _get_dp_dm(distances, targets, plabels):
|
|
||||||
"""Returns the d+ and d- values for a batch of distances."""
|
|
||||||
matcher = _get_matcher(targets, plabels)
|
|
||||||
not_matcher = torch.bitwise_not(matcher)
|
|
||||||
|
|
||||||
inf = torch.full_like(distances, fill_value=float("inf"))
|
|
||||||
d_matching = torch.where(matcher, distances, inf)
|
|
||||||
d_unmatching = torch.where(not_matcher, distances, inf)
|
|
||||||
dp = torch.min(d_matching, dim=1, keepdim=True).values
|
|
||||||
dm = torch.min(d_unmatching, dim=1, keepdim=True).values
|
|
||||||
return dp, dm
|
|
||||||
|
|
||||||
|
|
||||||
def glvq_loss(distances, target_labels, prototype_labels):
|
|
||||||
"""GLVQ loss function with support for one-hot labels."""
|
|
||||||
dp, dm = _get_dp_dm(distances, target_labels, prototype_labels)
|
|
||||||
mu = (dp - dm) / (dp + dm)
|
|
||||||
return mu
|
|
||||||
|
|
||||||
|
|
||||||
def lvq1_loss(distances, target_labels, prototype_labels):
|
|
||||||
"""LVQ1 loss function with support for one-hot labels.
|
|
||||||
|
|
||||||
See Section 4 [Sado&Yamada]
|
|
||||||
https://papers.nips.cc/paper/1995/file/9c3b1830513cc3b8fc4b76635d32e692-Paper.pdf
|
|
||||||
"""
|
|
||||||
dp, dm = _get_dp_dm(distances, target_labels, prototype_labels)
|
|
||||||
mu = dp
|
|
||||||
mu[dp > dm] = -dm[dp > dm]
|
|
||||||
return mu
|
|
||||||
|
|
||||||
|
|
||||||
def lvq21_loss(distances, target_labels, prototype_labels):
|
|
||||||
"""LVQ2.1 loss function with support for one-hot labels.
|
|
||||||
|
|
||||||
See Section 4 [Sado&Yamada]
|
|
||||||
https://papers.nips.cc/paper/1995/file/9c3b1830513cc3b8fc4b76635d32e692-Paper.pdf
|
|
||||||
"""
|
|
||||||
dp, dm = _get_dp_dm(distances, target_labels, prototype_labels)
|
|
||||||
mu = dp - dm
|
|
||||||
return mu
|
|
@ -1,35 +0,0 @@
|
|||||||
# -*- coding: utf-8 -*-
|
|
||||||
from __future__ import absolute_import, division, print_function
|
|
||||||
|
|
||||||
import torch
|
|
||||||
|
|
||||||
|
|
||||||
def orthogonalization(tensors):
|
|
||||||
r""" Orthogonalization of a given tensor via polar decomposition.
|
|
||||||
"""
|
|
||||||
u, _, v = torch.svd(tensors, compute_uv=True)
|
|
||||||
u_shape = tuple(list(u.shape))
|
|
||||||
v_shape = tuple(list(v.shape))
|
|
||||||
|
|
||||||
# reshape to (num x N x M)
|
|
||||||
u = torch.reshape(u, (-1, u_shape[-2], u_shape[-1]))
|
|
||||||
v = torch.reshape(v, (-1, v_shape[-2], v_shape[-1]))
|
|
||||||
|
|
||||||
out = u @ v.permute([0, 2, 1])
|
|
||||||
|
|
||||||
out = torch.reshape(out, u_shape[:-1] + (v_shape[-2], ))
|
|
||||||
|
|
||||||
return out
|
|
||||||
|
|
||||||
|
|
||||||
def trace_normalization(tensors):
|
|
||||||
r""" Trace normalization
|
|
||||||
"""
|
|
||||||
epsilon = torch.tensor([1e-10], dtype=torch.float64)
|
|
||||||
# Scope trace_normalization
|
|
||||||
constant = torch.trace(tensors)
|
|
||||||
|
|
||||||
if epsilon != 0:
|
|
||||||
constant = torch.max(constant, epsilon)
|
|
||||||
|
|
||||||
return tensors / constant
|
|
@ -1,7 +0,0 @@
|
|||||||
"""ProtoTorch modules."""
|
|
||||||
|
|
||||||
from .prototypes import Prototypes1D
|
|
||||||
|
|
||||||
__all__ = [
|
|
||||||
"Prototypes1D",
|
|
||||||
]
|
|
@ -1,40 +0,0 @@
|
|||||||
"""ProtoTorch losses."""
|
|
||||||
|
|
||||||
import torch
|
|
||||||
|
|
||||||
from prototorch.functions.activations import get_activation
|
|
||||||
from prototorch.functions.losses import glvq_loss
|
|
||||||
|
|
||||||
|
|
||||||
class GLVQLoss(torch.nn.Module):
|
|
||||||
def __init__(self, margin=0.0, squashing="identity", beta=10, **kwargs):
|
|
||||||
super().__init__(**kwargs)
|
|
||||||
self.margin = margin
|
|
||||||
self.squashing = get_activation(squashing)
|
|
||||||
self.beta = torch.tensor(beta)
|
|
||||||
|
|
||||||
def forward(self, outputs, targets):
|
|
||||||
distances, plabels = outputs
|
|
||||||
mu = glvq_loss(distances, targets, prototype_labels=plabels)
|
|
||||||
batch_loss = self.squashing(mu + self.margin, beta=self.beta)
|
|
||||||
return torch.sum(batch_loss, dim=0)
|
|
||||||
|
|
||||||
|
|
||||||
class NeuralGasEnergy(torch.nn.Module):
|
|
||||||
def __init__(self, lm):
|
|
||||||
super().__init__()
|
|
||||||
self.lm = lm
|
|
||||||
|
|
||||||
def forward(self, d):
|
|
||||||
order = torch.argsort(d, dim=1)
|
|
||||||
ranks = torch.argsort(order, dim=1)
|
|
||||||
cost = torch.sum(self._nghood_fn(ranks, self.lm) * d)
|
|
||||||
|
|
||||||
return cost, order
|
|
||||||
|
|
||||||
def extra_repr(self):
|
|
||||||
return f"lambda: {self.lm}"
|
|
||||||
|
|
||||||
@staticmethod
|
|
||||||
def _nghood_fn(rankings, lm):
|
|
||||||
return torch.exp(-rankings / lm)
|
|
@ -1,195 +0,0 @@
|
|||||||
import torch
|
|
||||||
from torch import nn
|
|
||||||
|
|
||||||
from prototorch.functions.distances import (euclidean_distance_matrix,
|
|
||||||
tangent_distance)
|
|
||||||
from prototorch.functions.helper import _check_shapes, _int_and_mixed_shape
|
|
||||||
from prototorch.functions.normalization import orthogonalization
|
|
||||||
from prototorch.modules.prototypes import Prototypes1D
|
|
||||||
|
|
||||||
|
|
||||||
class GTLVQ(nn.Module):
|
|
||||||
r""" Generalized Tangent Learning Vector Quantization
|
|
||||||
|
|
||||||
Parameters
|
|
||||||
----------
|
|
||||||
num_classes: int
|
|
||||||
Number of classes of the given classification problem.
|
|
||||||
|
|
||||||
subspace_data: torch.tensor of shape (n_batch,feature_dim,feature_dim)
|
|
||||||
Subspace data for the point approximation, required
|
|
||||||
|
|
||||||
prototype_data: torch.tensor of shape (n_init_data,feature_dim) (optional)
|
|
||||||
prototype data for initalization of the prototypes used in GTLVQ.
|
|
||||||
|
|
||||||
subspace_size: int (default=256,optional)
|
|
||||||
Subspace dimension of the Projectors. Currently only supported
|
|
||||||
with tagnent_projection_type=global.
|
|
||||||
|
|
||||||
tangent_projection_type: string
|
|
||||||
Specifies the tangent projection type
|
|
||||||
options: local
|
|
||||||
local_proj
|
|
||||||
global
|
|
||||||
local: computes the tangent distances without emphasizing projected
|
|
||||||
data. Only distances are available
|
|
||||||
local_proj: computs tangent distances and returns the projected data
|
|
||||||
for further use. Be careful: data is repeated by number of prototypes
|
|
||||||
global: Number of subspaces is set to one and every prototypes
|
|
||||||
uses the same.
|
|
||||||
|
|
||||||
prototypes_per_class: int (default=2,optional)
|
|
||||||
Number of prototypes per class
|
|
||||||
|
|
||||||
feature_dim: int (default=256)
|
|
||||||
Dimensionality of the feature space specified as integer.
|
|
||||||
Prototype dimension.
|
|
||||||
|
|
||||||
Notes
|
|
||||||
-----
|
|
||||||
The GTLVQ [1] is a prototype-based classification learning model. The
|
|
||||||
GTLVQ uses the Tangent-Distances for a local point approximation
|
|
||||||
of an assumed data manifold via prototypial representations.
|
|
||||||
|
|
||||||
The GTLVQ requires subspace projectors for transforming the data
|
|
||||||
and prototypes into the affine subspace. Every prototype is
|
|
||||||
equipped with a specific subpspace and represents a point
|
|
||||||
approximation of the assumed manifold.
|
|
||||||
|
|
||||||
In practice prototypes and data are projected on this manifold
|
|
||||||
and pairwise euclidean distance computes.
|
|
||||||
|
|
||||||
References
|
|
||||||
----------
|
|
||||||
.. [1] Saralajew, Sascha; Villmann, Thomas: Transfer learning
|
|
||||||
in classification based on manifolc. models and its relation
|
|
||||||
to tangent metric learning. In: 2017 International Joint
|
|
||||||
Conference on Neural Networks (IJCNN).
|
|
||||||
Bd. 2017-May : IEEE, 2017, S. 1756–1765
|
|
||||||
"""
|
|
||||||
def __init__(
|
|
||||||
self,
|
|
||||||
num_classes,
|
|
||||||
subspace_data=None,
|
|
||||||
prototype_data=None,
|
|
||||||
subspace_size=256,
|
|
||||||
tangent_projection_type="local",
|
|
||||||
prototypes_per_class=2,
|
|
||||||
feature_dim=256,
|
|
||||||
):
|
|
||||||
super(GTLVQ, self).__init__()
|
|
||||||
|
|
||||||
self.num_protos = num_classes * prototypes_per_class
|
|
||||||
self.subspace_size = feature_dim if subspace_size is None else subspace_size
|
|
||||||
self.feature_dim = feature_dim
|
|
||||||
|
|
||||||
if subspace_data is None:
|
|
||||||
raise ValueError("Init Data must be specified!")
|
|
||||||
|
|
||||||
self.tpt = tangent_projection_type
|
|
||||||
with torch.no_grad():
|
|
||||||
if self.tpt == "local" or self.tpt == "local_proj":
|
|
||||||
self.init_local_subspace(subspace_data)
|
|
||||||
elif self.tpt == "global":
|
|
||||||
self.init_gobal_subspace(subspace_data, subspace_size)
|
|
||||||
else:
|
|
||||||
self.subspaces = None
|
|
||||||
|
|
||||||
# Hypothesis-Margin-Classifier
|
|
||||||
self.cls = Prototypes1D(
|
|
||||||
input_dim=feature_dim,
|
|
||||||
prototypes_per_class=prototypes_per_class,
|
|
||||||
nclasses=num_classes,
|
|
||||||
prototype_initializer="stratified_mean",
|
|
||||||
data=prototype_data,
|
|
||||||
)
|
|
||||||
|
|
||||||
def forward(self, x):
|
|
||||||
# Tangent Projection
|
|
||||||
if self.tpt == "local_proj":
|
|
||||||
x_conform = (x.unsqueeze(1).repeat_interleave(self.num_protos,
|
|
||||||
1).unsqueeze(2))
|
|
||||||
dis, proj_x = self.local_tangent_projection(x_conform)
|
|
||||||
|
|
||||||
proj_x = proj_x.reshape(x.shape[0] * self.num_protos,
|
|
||||||
self.feature_dim)
|
|
||||||
return proj_x, dis
|
|
||||||
elif self.tpt == "local":
|
|
||||||
x_conform = (x.unsqueeze(1).repeat_interleave(self.num_protos,
|
|
||||||
1).unsqueeze(2))
|
|
||||||
dis = tangent_distance(x_conform, self.cls.prototypes,
|
|
||||||
self.subspaces)
|
|
||||||
elif self.tpt == "gloabl":
|
|
||||||
dis = self.global_tangent_distances(x)
|
|
||||||
else:
|
|
||||||
dis = (x @ self.cls.prototypes.T) / (
|
|
||||||
torch.norm(x, dim=1, keepdim=True) @ torch.norm(
|
|
||||||
self.cls.prototypes, dim=1, keepdim=True).T)
|
|
||||||
return dis
|
|
||||||
|
|
||||||
def init_gobal_subspace(self, data, num_subspaces):
|
|
||||||
_, _, v = torch.svd(data)
|
|
||||||
subspace = (torch.eye(v.shape[0]) - (v @ v.T)).T
|
|
||||||
subspaces = subspace[:, :num_subspaces]
|
|
||||||
self.subspaces = (torch.nn.Parameter(
|
|
||||||
subspaces).clone().detach().requires_grad_(True))
|
|
||||||
|
|
||||||
def init_local_subspace(self, data):
|
|
||||||
_, _, v = torch.svd(data)
|
|
||||||
inital_projector = (torch.eye(v.shape[0]) - (v @ v.T)).T
|
|
||||||
subspaces = inital_projector.unsqueeze(0).repeat_interleave(
|
|
||||||
self.num_protos, 0)
|
|
||||||
self.subspaces = (torch.nn.Parameter(
|
|
||||||
subspaces).clone().detach().requires_grad_(True))
|
|
||||||
|
|
||||||
def global_tangent_distances(self, x):
|
|
||||||
# Tangent Projection
|
|
||||||
x, projected_prototypes = (
|
|
||||||
x @ self.subspaces,
|
|
||||||
self.cls.prototypes @ self.subspaces,
|
|
||||||
)
|
|
||||||
# Euclidean Distance
|
|
||||||
return euclidean_distance_matrix(x, projected_prototypes)
|
|
||||||
|
|
||||||
def local_tangent_projection(self, signals):
|
|
||||||
# Note: subspaces is always assumed as transposed and must be orthogonal!
|
|
||||||
# shape(signals): batch x proto_number x channels x dim1 x dim2 x ... x dimN
|
|
||||||
# shape(protos): proto_number x dim1 x dim2 x ... x dimN
|
|
||||||
# shape(subspaces): (optional [proto_number]) x prod(dim1 * dim2 * ... * dimN) x prod(projected_atom_shape)
|
|
||||||
# subspace should be orthogonalized
|
|
||||||
# Origin Source Code
|
|
||||||
# Origin Author:
|
|
||||||
protos = self.cls.prototypes
|
|
||||||
subspaces = self.subspaces
|
|
||||||
signal_shape, signal_int_shape = _int_and_mixed_shape(signals)
|
|
||||||
_, proto_int_shape = _int_and_mixed_shape(protos)
|
|
||||||
|
|
||||||
# check if the shapes are correct
|
|
||||||
_check_shapes(signal_int_shape, proto_int_shape)
|
|
||||||
|
|
||||||
# Tangent Data Projections
|
|
||||||
projected_protos = torch.bmm(protos.unsqueeze(1), subspaces).squeeze(1)
|
|
||||||
data = signals.squeeze(2).permute([1, 0, 2])
|
|
||||||
projected_data = torch.bmm(data, subspaces)
|
|
||||||
projected_data = projected_data.permute([1, 0, 2]).unsqueeze(1)
|
|
||||||
diff = projected_data - projected_protos
|
|
||||||
projected_diff = torch.reshape(
|
|
||||||
diff, (signal_shape[1], signal_shape[0], signal_shape[2]) +
|
|
||||||
signal_shape[3:])
|
|
||||||
diss = torch.norm(projected_diff, 2, dim=-1)
|
|
||||||
return diss.permute([1, 0, 2]).squeeze(-1), projected_data.squeeze(1)
|
|
||||||
|
|
||||||
def get_parameters(self):
|
|
||||||
return {
|
|
||||||
"params": self.cls.prototypes,
|
|
||||||
}, {
|
|
||||||
"params": self.subspaces
|
|
||||||
}
|
|
||||||
|
|
||||||
def orthogonalize_subspace(self):
|
|
||||||
if self.subspaces is not None:
|
|
||||||
with torch.no_grad():
|
|
||||||
ortho_subpsaces = (orthogonalization(self.subspaces)
|
|
||||||
if self.tpt == "global" else
|
|
||||||
torch.nn.init.orthogonal_(self.subspaces))
|
|
||||||
self.subspaces.copy_(ortho_subpsaces)
|
|
@ -1,137 +0,0 @@
|
|||||||
"""ProtoTorch prototype modules."""
|
|
||||||
|
|
||||||
import warnings
|
|
||||||
|
|
||||||
import torch
|
|
||||||
|
|
||||||
from prototorch.functions.initializers import get_initializer
|
|
||||||
|
|
||||||
|
|
||||||
class _Prototypes(torch.nn.Module):
|
|
||||||
"""Abstract prototypes class."""
|
|
||||||
def __init__(self, *args, **kwargs):
|
|
||||||
super().__init__(*args, **kwargs)
|
|
||||||
|
|
||||||
def _validate_prototype_distribution(self):
|
|
||||||
if 0 in self.prototype_distribution:
|
|
||||||
warnings.warn("Are you sure about the `0` in "
|
|
||||||
"`prototype_distribution`?")
|
|
||||||
|
|
||||||
def extra_repr(self):
|
|
||||||
return f"prototypes.shape: {tuple(self.prototypes.shape)}"
|
|
||||||
|
|
||||||
def forward(self):
|
|
||||||
return self.prototypes, self.prototype_labels
|
|
||||||
|
|
||||||
|
|
||||||
class Prototypes1D(_Prototypes):
|
|
||||||
"""Create a learnable set of one-dimensional prototypes.
|
|
||||||
|
|
||||||
TODO Complete this doc-string.
|
|
||||||
"""
|
|
||||||
def __init__(
|
|
||||||
self,
|
|
||||||
prototypes_per_class=1,
|
|
||||||
prototype_initializer="ones",
|
|
||||||
prototype_distribution=None,
|
|
||||||
data=None,
|
|
||||||
dtype=torch.float32,
|
|
||||||
one_hot_labels=False,
|
|
||||||
**kwargs,
|
|
||||||
):
|
|
||||||
warnings.warn(
|
|
||||||
PendingDeprecationWarning(
|
|
||||||
"Prototypes1D will be replaced in future versions."))
|
|
||||||
|
|
||||||
# Convert tensors to python lists before processing
|
|
||||||
if prototype_distribution is not None:
|
|
||||||
if not isinstance(prototype_distribution, list):
|
|
||||||
prototype_distribution = prototype_distribution.tolist()
|
|
||||||
|
|
||||||
if data is None:
|
|
||||||
if "input_dim" not in kwargs:
|
|
||||||
raise NameError("`input_dim` required if "
|
|
||||||
"no `data` is provided.")
|
|
||||||
if prototype_distribution:
|
|
||||||
kwargs_nclasses = sum(prototype_distribution)
|
|
||||||
else:
|
|
||||||
if "nclasses" not in kwargs:
|
|
||||||
raise NameError("`prototype_distribution` required if "
|
|
||||||
"both `data` and `nclasses` are not "
|
|
||||||
"provided.")
|
|
||||||
kwargs_nclasses = kwargs.pop("nclasses")
|
|
||||||
input_dim = kwargs.pop("input_dim")
|
|
||||||
if prototype_initializer in [
|
|
||||||
"stratified_mean", "stratified_random"
|
|
||||||
]:
|
|
||||||
warnings.warn(
|
|
||||||
f"`prototype_initializer`: `{prototype_initializer}` "
|
|
||||||
"requires `data`, but `data` is not provided. "
|
|
||||||
"Using randomly generated data instead.")
|
|
||||||
x_train = torch.rand(kwargs_nclasses, input_dim)
|
|
||||||
y_train = torch.arange(kwargs_nclasses)
|
|
||||||
if one_hot_labels:
|
|
||||||
y_train = torch.eye(kwargs_nclasses)[y_train]
|
|
||||||
data = [x_train, y_train]
|
|
||||||
|
|
||||||
x_train, y_train = data
|
|
||||||
x_train = torch.as_tensor(x_train).type(dtype)
|
|
||||||
y_train = torch.as_tensor(y_train).type(torch.int)
|
|
||||||
nclasses = torch.unique(y_train, dim=-1).shape[-1]
|
|
||||||
|
|
||||||
if nclasses == 1:
|
|
||||||
warnings.warn("Are you sure about having one class only?")
|
|
||||||
|
|
||||||
if x_train.ndim != 2:
|
|
||||||
raise ValueError("`data[0].ndim != 2`.")
|
|
||||||
|
|
||||||
if y_train.ndim == 2:
|
|
||||||
if y_train.shape[1] == 1 and one_hot_labels:
|
|
||||||
raise ValueError("`one_hot_labels` is set to `True` "
|
|
||||||
"but target labels are not one-hot-encoded.")
|
|
||||||
if y_train.shape[1] != 1 and not one_hot_labels:
|
|
||||||
raise ValueError("`one_hot_labels` is set to `False` "
|
|
||||||
"but target labels in `data` "
|
|
||||||
"are one-hot-encoded.")
|
|
||||||
if y_train.ndim == 1 and one_hot_labels:
|
|
||||||
raise ValueError("`one_hot_labels` is set to `True` "
|
|
||||||
"but target labels are not one-hot-encoded.")
|
|
||||||
|
|
||||||
# Verify input dimension if `input_dim` is provided
|
|
||||||
if "input_dim" in kwargs:
|
|
||||||
input_dim = kwargs.pop("input_dim")
|
|
||||||
if input_dim != x_train.shape[1]:
|
|
||||||
raise ValueError(f"Provided `input_dim`={input_dim} does "
|
|
||||||
"not match data dimension "
|
|
||||||
f"`data[0].shape[1]`={x_train.shape[1]}")
|
|
||||||
|
|
||||||
# Verify the number of classes if `nclasses` is provided
|
|
||||||
if "nclasses" in kwargs:
|
|
||||||
kwargs_nclasses = kwargs.pop("nclasses")
|
|
||||||
if kwargs_nclasses != nclasses:
|
|
||||||
raise ValueError(f"Provided `nclasses={kwargs_nclasses}` does "
|
|
||||||
"not match data labels "
|
|
||||||
"`torch.unique(data[1]).shape[0]`"
|
|
||||||
f"={nclasses}")
|
|
||||||
|
|
||||||
super().__init__(**kwargs)
|
|
||||||
|
|
||||||
if not prototype_distribution:
|
|
||||||
prototype_distribution = [prototypes_per_class] * nclasses
|
|
||||||
with torch.no_grad():
|
|
||||||
self.prototype_distribution = torch.tensor(prototype_distribution)
|
|
||||||
|
|
||||||
self._validate_prototype_distribution()
|
|
||||||
|
|
||||||
self.prototype_initializer = get_initializer(prototype_initializer)
|
|
||||||
prototypes, prototype_labels = self.prototype_initializer(
|
|
||||||
x_train,
|
|
||||||
y_train,
|
|
||||||
prototype_distribution=self.prototype_distribution,
|
|
||||||
one_hot=one_hot_labels,
|
|
||||||
)
|
|
||||||
|
|
||||||
# Register module parameters
|
|
||||||
self.prototypes = torch.nn.Parameter(prototypes)
|
|
||||||
self.prototype_labels = torch.nn.Parameter(
|
|
||||||
prototype_labels.type(dtype)).requires_grad_(False)
|
|
4
prototorch/nn/__init__.py
Normal file
4
prototorch/nn/__init__.py
Normal file
@ -0,0 +1,4 @@
|
|||||||
|
"""ProtoTorch Neural Network Module"""
|
||||||
|
|
||||||
|
from .activations import *
|
||||||
|
from .wrappers import *
|
@ -1,21 +1,18 @@
|
|||||||
"""ProtoTorch activation functions."""
|
"""ProtoTorch activations"""
|
||||||
|
|
||||||
import torch
|
import torch
|
||||||
|
|
||||||
ACTIVATIONS = dict()
|
ACTIVATIONS = dict()
|
||||||
|
|
||||||
|
|
||||||
# def register_activation(scriptf):
|
def register_activation(fn):
|
||||||
# ACTIVATIONS[scriptf.name] = scriptf
|
|
||||||
# return scriptf
|
|
||||||
def register_activation(function):
|
|
||||||
"""Add the activation function to the registry."""
|
"""Add the activation function to the registry."""
|
||||||
ACTIVATIONS[function.__name__] = function
|
name = fn.__name__
|
||||||
return function
|
ACTIVATIONS[name] = fn
|
||||||
|
return fn
|
||||||
|
|
||||||
|
|
||||||
@register_activation
|
@register_activation
|
||||||
# @torch.jit.script
|
|
||||||
def identity(x, beta=0.0):
|
def identity(x, beta=0.0):
|
||||||
"""Identity activation function.
|
"""Identity activation function.
|
||||||
|
|
||||||
@ -29,7 +26,6 @@ def identity(x, beta=0.0):
|
|||||||
|
|
||||||
|
|
||||||
@register_activation
|
@register_activation
|
||||||
# @torch.jit.script
|
|
||||||
def sigmoid_beta(x, beta=10.0):
|
def sigmoid_beta(x, beta=10.0):
|
||||||
r"""Sigmoid activation function with scaling.
|
r"""Sigmoid activation function with scaling.
|
||||||
|
|
||||||
@ -44,7 +40,6 @@ def sigmoid_beta(x, beta=10.0):
|
|||||||
|
|
||||||
|
|
||||||
@register_activation
|
@register_activation
|
||||||
# @torch.jit.script
|
|
||||||
def swish_beta(x, beta=10.0):
|
def swish_beta(x, beta=10.0):
|
||||||
r"""Swish activation function with scaling.
|
r"""Swish activation function with scaling.
|
||||||
|
|
||||||
@ -62,6 +57,10 @@ def get_activation(funcname):
|
|||||||
"""Deserialize the activation function."""
|
"""Deserialize the activation function."""
|
||||||
if callable(funcname):
|
if callable(funcname):
|
||||||
return funcname
|
return funcname
|
||||||
if funcname in ACTIVATIONS:
|
elif funcname in ACTIVATIONS:
|
||||||
return ACTIVATIONS.get(funcname)
|
return ACTIVATIONS.get(funcname)
|
||||||
raise NameError(f"Activation {funcname} was not found.")
|
else:
|
||||||
|
emsg = f"Unable to find matching function for `{funcname}` " \
|
||||||
|
f"in `prototorch.nn.activations`. "
|
||||||
|
helpmsg = f"Possible values are {list(ACTIVATIONS.keys())}."
|
||||||
|
raise NameError(emsg + helpmsg)
|
38
prototorch/nn/wrappers.py
Normal file
38
prototorch/nn/wrappers.py
Normal file
@ -0,0 +1,38 @@
|
|||||||
|
"""ProtoTorch wrappers."""
|
||||||
|
|
||||||
|
import torch
|
||||||
|
|
||||||
|
|
||||||
|
class LambdaLayer(torch.nn.Module):
|
||||||
|
|
||||||
|
def __init__(self, fn, name=None):
|
||||||
|
super().__init__()
|
||||||
|
self.fn = fn
|
||||||
|
self.name = name or fn.__name__ # lambda fns get <lambda>
|
||||||
|
|
||||||
|
def forward(self, *args, **kwargs):
|
||||||
|
return self.fn(*args, **kwargs)
|
||||||
|
|
||||||
|
def extra_repr(self):
|
||||||
|
return self.name
|
||||||
|
|
||||||
|
|
||||||
|
class LossLayer(torch.nn.modules.loss._Loss):
|
||||||
|
|
||||||
|
def __init__(self,
|
||||||
|
fn,
|
||||||
|
name=None,
|
||||||
|
size_average=None,
|
||||||
|
reduce=None,
|
||||||
|
reduction: str = "mean") -> None:
|
||||||
|
super().__init__(size_average=size_average,
|
||||||
|
reduce=reduce,
|
||||||
|
reduction=reduction)
|
||||||
|
self.fn = fn
|
||||||
|
self.name = name or fn.__name__ # lambda fns get <lambda>
|
||||||
|
|
||||||
|
def forward(self, *args, **kwargs):
|
||||||
|
return self.fn(*args, **kwargs)
|
||||||
|
|
||||||
|
def extra_repr(self):
|
||||||
|
return self.name
|
@ -0,0 +1,13 @@
|
|||||||
|
"""ProtoTorch utils module"""
|
||||||
|
|
||||||
|
from .colors import (
|
||||||
|
get_colors,
|
||||||
|
get_legend_handles,
|
||||||
|
hex_to_rgb,
|
||||||
|
rgb_to_hex,
|
||||||
|
)
|
||||||
|
from .utils import (
|
||||||
|
mesh2d,
|
||||||
|
parse_data_arg,
|
||||||
|
parse_distribution,
|
||||||
|
)
|
@ -1,46 +0,0 @@
|
|||||||
"""Easy matplotlib animation. From https://github.com/jwkvam/celluloid."""
|
|
||||||
|
|
||||||
from collections import defaultdict
|
|
||||||
from typing import Dict, List
|
|
||||||
|
|
||||||
from matplotlib.animation import ArtistAnimation
|
|
||||||
from matplotlib.artist import Artist
|
|
||||||
from matplotlib.figure import Figure
|
|
||||||
|
|
||||||
__version__ = "0.2.0"
|
|
||||||
|
|
||||||
|
|
||||||
class Camera:
|
|
||||||
"""Make animations easier."""
|
|
||||||
def __init__(self, figure: Figure) -> None:
|
|
||||||
"""Create camera from matplotlib figure."""
|
|
||||||
self._figure = figure
|
|
||||||
# need to keep track off artists for each axis
|
|
||||||
self._offsets: Dict[str, Dict[int, int]] = {
|
|
||||||
k: defaultdict(int)
|
|
||||||
for k in
|
|
||||||
["collections", "patches", "lines", "texts", "artists", "images"]
|
|
||||||
}
|
|
||||||
self._photos: List[List[Artist]] = []
|
|
||||||
|
|
||||||
def snap(self) -> List[Artist]:
|
|
||||||
"""Capture current state of the figure."""
|
|
||||||
frame_artists: List[Artist] = []
|
|
||||||
for i, axis in enumerate(self._figure.axes):
|
|
||||||
if axis.legend_ is not None:
|
|
||||||
axis.add_artist(axis.legend_)
|
|
||||||
for name in self._offsets:
|
|
||||||
new_artists = getattr(axis, name)[self._offsets[name][i]:]
|
|
||||||
frame_artists += new_artists
|
|
||||||
self._offsets[name][i] += len(new_artists)
|
|
||||||
self._photos.append(frame_artists)
|
|
||||||
return frame_artists
|
|
||||||
|
|
||||||
def animate(self, *args, **kwargs) -> ArtistAnimation:
|
|
||||||
"""Animate the snapshots taken.
|
|
||||||
Uses matplotlib.animation.ArtistAnimation
|
|
||||||
Returns
|
|
||||||
-------
|
|
||||||
ArtistAnimation
|
|
||||||
"""
|
|
||||||
return ArtistAnimation(self._figure, self._photos, *args, **kwargs)
|
|
@ -1,78 +1,60 @@
|
|||||||
"""ProtoFlow color utilities."""
|
"""ProtoTorch color utilities"""
|
||||||
|
|
||||||
import matplotlib.lines as mlines
|
import matplotlib.lines as mlines
|
||||||
|
import torch
|
||||||
from matplotlib import cm
|
from matplotlib import cm
|
||||||
from matplotlib.colors import Normalize, to_hex, to_rgb
|
from matplotlib.colors import (
|
||||||
|
Normalize,
|
||||||
|
to_hex,
|
||||||
|
to_rgb,
|
||||||
|
)
|
||||||
|
|
||||||
|
|
||||||
def color_scheme(n,
|
def hex_to_rgb(hex_values):
|
||||||
cmap="viridis",
|
for v in hex_values:
|
||||||
form="hex",
|
v = v.lstrip('#')
|
||||||
tikz=False,
|
lv = len(v)
|
||||||
zero_indexed=False):
|
c = [int(v[i:i + lv // 3], 16) for i in range(0, lv, lv // 3)]
|
||||||
"""Return *n* colors from the color scheme.
|
yield c
|
||||||
|
|
||||||
Arguments:
|
|
||||||
n (int): number of colors to return
|
|
||||||
|
|
||||||
Keyword Arguments:
|
def rgb_to_hex(rgb_values):
|
||||||
cmap (str): Name of a matplotlib `colormap\
|
for v in rgb_values:
|
||||||
<https://matplotlib.org/3.1.1/gallery/color/colormap_reference.html>`_.
|
c = "%02x%02x%02x" % tuple(v)
|
||||||
form (str): Colorformat (supports "hex" and "rgb").
|
yield c
|
||||||
tikz (bool): Output as `TikZ <https://github.com/pgf-tikz/pgf>`_
|
|
||||||
command.
|
|
||||||
zero_indexed (bool): Use zero indexing for output array.
|
|
||||||
|
|
||||||
Returns:
|
|
||||||
(list): List of colors
|
def get_colors(vmax, vmin=0, cmap="viridis"):
|
||||||
"""
|
|
||||||
cmap = cm.get_cmap(cmap)
|
cmap = cm.get_cmap(cmap)
|
||||||
colornorm = Normalize(vmin=1, vmax=n)
|
colornorm = Normalize(vmin=vmin, vmax=vmax)
|
||||||
hex_map = dict()
|
colors = dict()
|
||||||
rgb_map = dict()
|
for c in range(vmin, vmax + 1):
|
||||||
for cl in range(1, n + 1):
|
colors[c] = to_hex(cmap(colornorm(c)))
|
||||||
if zero_indexed:
|
return colors
|
||||||
hex_map[cl - 1] = to_hex(cmap(colornorm(cl)))
|
|
||||||
rgb_map[cl - 1] = to_rgb(cmap(colornorm(cl)))
|
|
||||||
else:
|
|
||||||
hex_map[cl] = to_hex(cmap(colornorm(cl)))
|
|
||||||
rgb_map[cl] = to_rgb(cmap(colornorm(cl)))
|
|
||||||
if tikz:
|
|
||||||
for k, v in rgb_map.items():
|
|
||||||
print(f"\\definecolor{{color-{k}}}{{rgb}}{{{v[0]},{v[1]},{v[2]}}}")
|
|
||||||
if form == "hex":
|
|
||||||
return hex_map
|
|
||||||
elif form == "rgb":
|
|
||||||
return rgb_map
|
|
||||||
else:
|
|
||||||
return hex_map
|
|
||||||
|
|
||||||
|
|
||||||
def get_legend_handles(labels, marker="dots", zero_indexed=False):
|
def get_legend_handles(colors, labels, marker="dots", zero_indexed=False):
|
||||||
"""Return matplotlib legend handles and colors."""
|
|
||||||
handles = list()
|
handles = list()
|
||||||
n = len(labels)
|
for color, label in zip(colors.values(), labels):
|
||||||
colors = color_scheme(n,
|
|
||||||
cmap="viridis",
|
|
||||||
form="hex",
|
|
||||||
zero_indexed=zero_indexed)
|
|
||||||
for label, color in zip(labels, colors.values()):
|
|
||||||
if marker == "dots":
|
if marker == "dots":
|
||||||
handle = mlines.Line2D(
|
handle = mlines.Line2D(
|
||||||
[],
|
xdata=[],
|
||||||
[],
|
ydata=[],
|
||||||
|
label=label,
|
||||||
color="white",
|
color="white",
|
||||||
markerfacecolor=color,
|
markerfacecolor=color,
|
||||||
marker="o",
|
marker="o",
|
||||||
markersize=10,
|
markersize=10,
|
||||||
markeredgecolor="k",
|
markeredgecolor="k",
|
||||||
label=label,
|
|
||||||
)
|
)
|
||||||
else:
|
else:
|
||||||
handle = mlines.Line2D([], [],
|
handle = mlines.Line2D(
|
||||||
|
xdata=[],
|
||||||
|
ydata=[],
|
||||||
|
label=label,
|
||||||
color=color,
|
color=color,
|
||||||
marker="",
|
marker="",
|
||||||
markersize=15,
|
markersize=15,
|
||||||
label=label)
|
)
|
||||||
handles.append(handle)
|
handles.append(handle)
|
||||||
return handles, colors
|
return handles
|
||||||
|
@ -1,243 +1,136 @@
|
|||||||
"""Utilities that provide various small functionalities."""
|
"""ProtoTorch utilities"""
|
||||||
|
|
||||||
import os
|
import warnings
|
||||||
import pickle
|
from typing import (
|
||||||
import sys
|
Dict,
|
||||||
from time import time
|
Iterable,
|
||||||
|
List,
|
||||||
|
Optional,
|
||||||
|
Union,
|
||||||
|
)
|
||||||
|
|
||||||
import matplotlib.pyplot as plt
|
|
||||||
import numpy as np
|
import numpy as np
|
||||||
|
import torch
|
||||||
|
from torch.utils.data import DataLoader, Dataset
|
||||||
|
|
||||||
|
|
||||||
def progressbar(title, value, end, bar_width=20):
|
def generate_mesh(
|
||||||
percent = float(value) / end
|
minima: torch.TensorType,
|
||||||
arrow = "=" * int(round(percent * bar_width) - 1) + ">"
|
maxima: torch.TensorType,
|
||||||
spaces = "." * (bar_width - len(arrow))
|
border: float = 1.0,
|
||||||
sys.stdout.write("\r{}: [{}] {}%".format(title, arrow + spaces,
|
resolution: int = 100,
|
||||||
int(round(percent * 100))))
|
device: Optional[torch.device] = None,
|
||||||
sys.stdout.flush()
|
):
|
||||||
if percent == 1.0:
|
# Apply Border
|
||||||
print()
|
ptp = maxima - minima
|
||||||
|
shift = border * ptp
|
||||||
|
minima -= shift
|
||||||
|
maxima += shift
|
||||||
|
|
||||||
|
# Generate Mesh
|
||||||
|
minima = minima.to(device).unsqueeze(1)
|
||||||
|
maxima = maxima.to(device).unsqueeze(1)
|
||||||
|
|
||||||
|
factors = torch.linspace(0, 1, resolution, device=device)
|
||||||
|
marginals = factors * maxima + ((1 - factors) * minima)
|
||||||
|
|
||||||
|
single_dimensions = torch.meshgrid(*marginals)
|
||||||
|
mesh_input = torch.stack([dim.ravel() for dim in single_dimensions], dim=1)
|
||||||
|
|
||||||
|
return mesh_input, single_dimensions
|
||||||
|
|
||||||
|
|
||||||
def prettify_string(inputs, start="", sep=" ", end="\n"):
|
def mesh2d(x=None, border: float = 1.0, resolution: int = 100):
|
||||||
outputs = start + " ".join(inputs.split()) + end
|
if x is not None:
|
||||||
return outputs
|
x_shift = border * np.ptp(x[:, 0])
|
||||||
|
y_shift = border * np.ptp(x[:, 1])
|
||||||
|
x_min, x_max = x[:, 0].min() - x_shift, x[:, 0].max() + x_shift
|
||||||
def pretty_print(inputs):
|
y_min, y_max = x[:, 1].min() - y_shift, x[:, 1].max() + y_shift
|
||||||
print(prettify_string(inputs))
|
|
||||||
|
|
||||||
|
|
||||||
def writelog(self, *logs, logdir="./logs", logfile="run.txt"):
|
|
||||||
f = os.path.join(logdir, logfile)
|
|
||||||
with open(f, "a+") as fh:
|
|
||||||
for log in logs:
|
|
||||||
fh.write(log)
|
|
||||||
fh.write("\n")
|
|
||||||
|
|
||||||
|
|
||||||
def start_tensorboard(self, logdir="./logs"):
|
|
||||||
cmd = f"tensorboard --logdir={logdir} --port=6006"
|
|
||||||
os.system(cmd)
|
|
||||||
|
|
||||||
|
|
||||||
def make_directory(save_dir):
|
|
||||||
if not os.path.exists(save_dir):
|
|
||||||
print(f"Making directory {save_dir}.")
|
|
||||||
os.mkdir(save_dir)
|
|
||||||
|
|
||||||
|
|
||||||
def make_gif(filenames, duration, output_file=None):
|
|
||||||
try:
|
|
||||||
import imageio
|
|
||||||
except ModuleNotFoundError as e:
|
|
||||||
print("Please install Protoflow with [other] extra requirements.")
|
|
||||||
raise (e)
|
|
||||||
|
|
||||||
images = list()
|
|
||||||
for filename in filenames:
|
|
||||||
images.append(imageio.imread(filename))
|
|
||||||
if not output_file:
|
|
||||||
output_file = f"makegif.gif"
|
|
||||||
if images:
|
|
||||||
imageio.mimwrite(output_file, images, duration=duration)
|
|
||||||
|
|
||||||
|
|
||||||
def gif_from_dir(directory,
|
|
||||||
duration,
|
|
||||||
prefix="",
|
|
||||||
output_file=None,
|
|
||||||
verbose=True):
|
|
||||||
images = os.listdir(directory)
|
|
||||||
if verbose:
|
|
||||||
print(f"Making gif from {len(images)} images under {directory}.")
|
|
||||||
filenames = list()
|
|
||||||
# Sort images
|
|
||||||
images = sorted(
|
|
||||||
images,
|
|
||||||
key=lambda img: int(os.path.splitext(img)[0].replace(prefix, "")))
|
|
||||||
for image in images:
|
|
||||||
fname = os.path.join(directory, image)
|
|
||||||
filenames.append(fname)
|
|
||||||
if not output_file:
|
|
||||||
output_file = os.path.join(directory, "makegif.gif")
|
|
||||||
make_gif(filenames=filenames, duration=duration, output_file=output_file)
|
|
||||||
|
|
||||||
|
|
||||||
def accuracy_score(y_true, y_pred):
|
|
||||||
accuracy = np.sum(y_true == y_pred)
|
|
||||||
normalized_acc = accuracy / float(len(y_true))
|
|
||||||
return normalized_acc
|
|
||||||
|
|
||||||
|
|
||||||
def predict_and_score(clf,
|
|
||||||
x_test,
|
|
||||||
y_test,
|
|
||||||
verbose=False,
|
|
||||||
title="Test accuracy"):
|
|
||||||
y_pred = clf.predict(x_test)
|
|
||||||
accuracy = np.sum(y_test == y_pred)
|
|
||||||
normalized_acc = accuracy / float(len(y_test))
|
|
||||||
if verbose:
|
|
||||||
print(f"{title}: {normalized_acc * 100:06.04f}%")
|
|
||||||
return normalized_acc
|
|
||||||
|
|
||||||
|
|
||||||
def remove_nan_rows(arr):
|
|
||||||
"""Remove all rows with `nan` values in `arr`."""
|
|
||||||
mask = np.isnan(arr).any(axis=1)
|
|
||||||
return arr[~mask]
|
|
||||||
|
|
||||||
|
|
||||||
def remove_nan_cols(arr):
|
|
||||||
"""Remove all columns with `nan` values in `arr`."""
|
|
||||||
mask = np.isnan(arr).any(axis=0)
|
|
||||||
return arr[~mask]
|
|
||||||
|
|
||||||
|
|
||||||
def replace_in(arr, replacement_dict, inplace=False):
|
|
||||||
"""Replace the keys found in `arr` with the values from
|
|
||||||
the `replacement_dict`.
|
|
||||||
"""
|
|
||||||
if inplace:
|
|
||||||
new_arr = arr
|
|
||||||
else:
|
else:
|
||||||
import copy
|
x_min, x_max = -border, border
|
||||||
|
y_min, y_max = -border, border
|
||||||
new_arr = copy.deepcopy(arr)
|
xx, yy = np.meshgrid(np.linspace(x_min, x_max, resolution),
|
||||||
for k, v in replacement_dict.items():
|
np.linspace(y_min, y_max, resolution))
|
||||||
new_arr[arr == k] = v
|
mesh = np.c_[xx.ravel(), yy.ravel()]
|
||||||
return new_arr
|
return mesh, xx, yy
|
||||||
|
|
||||||
|
|
||||||
def train_test_split(data, train=0.7, val=0.15, shuffle=None, return_xy=False):
|
def distribution_from_list(list_dist: List[int],
|
||||||
"""Split a classification dataset in such a way so as to
|
clabels: Optional[Iterable[int]] = None):
|
||||||
preserve the class distribution in subsamples of the dataset.
|
clabels = clabels or list(range(len(list_dist)))
|
||||||
|
distribution = dict(zip(clabels, list_dist))
|
||||||
|
return distribution
|
||||||
|
|
||||||
|
|
||||||
|
def parse_distribution(
|
||||||
|
user_distribution,
|
||||||
|
clabels: Optional[Iterable[int]] = None) -> Dict[int, int]:
|
||||||
|
"""Parse user-provided distribution.
|
||||||
|
|
||||||
|
Return a dictionary with integer keys that represent the class labels and
|
||||||
|
values that denote the number of components/prototypes with that class
|
||||||
|
label.
|
||||||
|
|
||||||
|
The argument `user_distribution` could be any one of a number of allowed
|
||||||
|
formats. If it is a Python list, it is assumed that there are as many
|
||||||
|
entries in this list as there are classes, and the value at each index of
|
||||||
|
this list describes the number of prototypes for that particular class. So,
|
||||||
|
[1, 1, 1] implies that we have three classes with one prototype per class.
|
||||||
|
If it is a Python tuple, a shorthand of (num_classes, prototypes_per_class)
|
||||||
|
is assumed. If it is a Python dictionary, the key-value pairs describe the
|
||||||
|
class label and the number of prototypes for that class respectively. So,
|
||||||
|
{0: 2, 1: 2, 2: 2} implies that we have three classes with labels {1, 2,
|
||||||
|
3}, each equipped with two prototypes. If however, the dictionary contains
|
||||||
|
the keys "num_classes" and "per_class", they are parsed to use their values
|
||||||
|
as one might expect.
|
||||||
|
|
||||||
"""
|
"""
|
||||||
if train + val > 1.0:
|
if isinstance(user_distribution, dict):
|
||||||
raise ValueError("Invalid split values for train and val.")
|
if "num_classes" in user_distribution.keys():
|
||||||
Y = data[:, -1]
|
num_classes = int(user_distribution["num_classes"])
|
||||||
labels = set(Y)
|
per_class = int(user_distribution["per_class"])
|
||||||
hist = dict()
|
return distribution_from_list([per_class] * num_classes, clabels)
|
||||||
for l in labels:
|
|
||||||
data_l = data[Y == l]
|
|
||||||
nl = len(data_l)
|
|
||||||
nl_train = int(nl * train)
|
|
||||||
nl_val = int(nl * val)
|
|
||||||
nl_test = nl - (nl_train + nl_val)
|
|
||||||
hist[l] = (nl_train, nl_val, nl_test)
|
|
||||||
|
|
||||||
train_data = list()
|
|
||||||
val_data = list()
|
|
||||||
test_data = list()
|
|
||||||
for l, (nl_train, nl_val, nl_test) in hist.items():
|
|
||||||
data_l = data[Y == l]
|
|
||||||
if shuffle:
|
|
||||||
np.random.shuffle(data_l)
|
|
||||||
train_l = data_l[:nl_train]
|
|
||||||
val_l = data_l[nl_train:nl_train + nl_val]
|
|
||||||
test_l = data_l[nl_train + nl_val:nl_train + nl_val + nl_test]
|
|
||||||
train_data.append(train_l)
|
|
||||||
val_data.append(val_l)
|
|
||||||
test_data.append(test_l)
|
|
||||||
|
|
||||||
def _squash(data_list):
|
|
||||||
data = np.array(data_list[0])
|
|
||||||
for item in data_list[1:]:
|
|
||||||
data = np.vstack((data, np.array(item)))
|
|
||||||
return data
|
|
||||||
|
|
||||||
train_data = _squash(train_data)
|
|
||||||
if val_data:
|
|
||||||
val_data = _squash(val_data)
|
|
||||||
if test_data:
|
|
||||||
test_data = _squash(test_data)
|
|
||||||
if return_xy:
|
|
||||||
x_train = train_data[:, :-1]
|
|
||||||
y_train = train_data[:, -1]
|
|
||||||
x_val = val_data[:, :-1]
|
|
||||||
y_val = val_data[:, -1]
|
|
||||||
x_test = test_data[:, :-1]
|
|
||||||
y_test = test_data[:, -1]
|
|
||||||
return (x_train, y_train), (x_val, y_val), (x_test, y_test)
|
|
||||||
return train_data, val_data, test_data
|
|
||||||
|
|
||||||
|
|
||||||
def class_histogram(data, title="Untitled"):
|
|
||||||
plt.figure(title)
|
|
||||||
plt.clf()
|
|
||||||
plt.title(title)
|
|
||||||
dist, counts = np.unique(data[:, -1], return_counts=True)
|
|
||||||
plt.bar(dist, counts)
|
|
||||||
plt.xticks(dist)
|
|
||||||
print("Call matplotlib.pyplot.show() to see the plot.")
|
|
||||||
|
|
||||||
|
|
||||||
def ntimer(n=10):
|
|
||||||
"""Wraps a function which wraps another function to time it."""
|
|
||||||
if n < 1:
|
|
||||||
raise (Exception(f"Invalid n = {n} given."))
|
|
||||||
|
|
||||||
def timer(func):
|
|
||||||
"""Wraps `func` with a timer and returns the wrapped `func`."""
|
|
||||||
def wrapper(*args, **kwargs):
|
|
||||||
rv = None
|
|
||||||
before = time()
|
|
||||||
for _ in range(n):
|
|
||||||
rv = func(*args, **kwargs)
|
|
||||||
after = time()
|
|
||||||
elapsed = after - before
|
|
||||||
print(f"Elapsed: {elapsed*1e3:02.02f} ms")
|
|
||||||
return rv
|
|
||||||
|
|
||||||
return wrapper
|
|
||||||
|
|
||||||
return timer
|
|
||||||
|
|
||||||
|
|
||||||
def memoize(verbose=True):
|
|
||||||
"""Wraps a function which wraps another function that memoizes."""
|
|
||||||
def memoizer(func):
|
|
||||||
"""Memoize (cache) return values of `func`.
|
|
||||||
Wraps `func` and returns the wrapped `func` so that `func`
|
|
||||||
is executed when the results are not available in the cache.
|
|
||||||
"""
|
|
||||||
cache = {}
|
|
||||||
|
|
||||||
def wrapper(*args, **kwargs):
|
|
||||||
t = (pickle.dumps(args), pickle.dumps(kwargs))
|
|
||||||
if t not in cache:
|
|
||||||
if verbose:
|
|
||||||
print(f"Adding NEW rv {func.__name__}{args}{kwargs} "
|
|
||||||
"to cache.")
|
|
||||||
cache[t] = func(*args, **kwargs)
|
|
||||||
else:
|
else:
|
||||||
if verbose:
|
return user_distribution
|
||||||
print(f"Using OLD rv {func.__name__}{args}{kwargs} "
|
elif isinstance(user_distribution, tuple):
|
||||||
"from cache.")
|
assert len(user_distribution) == 2
|
||||||
return cache[t]
|
num_classes, per_class = user_distribution
|
||||||
|
num_classes, per_class = int(num_classes), int(per_class)
|
||||||
|
return distribution_from_list([per_class] * num_classes, clabels)
|
||||||
|
elif isinstance(user_distribution, list):
|
||||||
|
return distribution_from_list(user_distribution, clabels)
|
||||||
|
else:
|
||||||
|
msg = f"`distribution` was not understood." \
|
||||||
|
f"You have provided: {user_distribution}."
|
||||||
|
raise ValueError(msg)
|
||||||
|
|
||||||
return wrapper
|
|
||||||
|
|
||||||
return memoizer
|
def parse_data_arg(data_arg: Union[Dataset, DataLoader, list, tuple]):
|
||||||
|
"""Return data and target as torch tensors."""
|
||||||
|
if isinstance(data_arg, Dataset):
|
||||||
|
if hasattr(data_arg, "__len__"):
|
||||||
|
ds_size = len(data_arg) # type: ignore
|
||||||
|
loader = DataLoader(data_arg, batch_size=ds_size)
|
||||||
|
data, targets = next(iter(loader))
|
||||||
|
else:
|
||||||
|
emsg = f"Dataset {data_arg} is not sized (`__len__` unimplemented)."
|
||||||
|
raise TypeError(emsg)
|
||||||
|
|
||||||
|
elif isinstance(data_arg, DataLoader):
|
||||||
|
data = torch.tensor([])
|
||||||
|
targets = torch.tensor([])
|
||||||
|
for x, y in data_arg:
|
||||||
|
data = torch.cat([data, x])
|
||||||
|
targets = torch.cat([targets, y])
|
||||||
|
else:
|
||||||
|
assert len(data_arg) == 2
|
||||||
|
data, targets = data_arg
|
||||||
|
if not isinstance(data, torch.Tensor):
|
||||||
|
wmsg = f"Converting data to {torch.Tensor}..."
|
||||||
|
warnings.warn(wmsg)
|
||||||
|
data = torch.Tensor(data)
|
||||||
|
if not isinstance(targets, torch.LongTensor):
|
||||||
|
wmsg = f"Converting targets to {torch.LongTensor}..."
|
||||||
|
warnings.warn(wmsg)
|
||||||
|
targets = torch.LongTensor(targets)
|
||||||
|
return data, targets
|
||||||
|
16
setup.cfg
Normal file
16
setup.cfg
Normal file
@ -0,0 +1,16 @@
|
|||||||
|
[pylint]
|
||||||
|
disable =
|
||||||
|
too-many-arguments,
|
||||||
|
too-few-public-methods,
|
||||||
|
fixme,
|
||||||
|
|
||||||
|
|
||||||
|
[pycodestyle]
|
||||||
|
max-line-length = 79
|
||||||
|
|
||||||
|
[isort]
|
||||||
|
multi_line_output = 3
|
||||||
|
include_trailing_comma = True
|
||||||
|
force_grid_wrap = 3
|
||||||
|
use_parentheses = True
|
||||||
|
line_length = 79
|
60
setup.py
60
setup.py
@ -1,10 +1,12 @@
|
|||||||
"""
|
"""
|
||||||
_____ _ _______ _
|
|
||||||
| __ \ | | |__ __| | |
|
######
|
||||||
| |__) | __ ___ | |_ ___ | | ___ _ __ ___| |__
|
# # ##### #### ##### #### ##### #### ##### #### # #
|
||||||
| ___/ '__/ _ \| __/ _ \| |/ _ \| '__/ __| '_ \
|
# # # # # # # # # # # # # # # # # #
|
||||||
| | | | | (_) | || (_) | | (_) | | | (__| | | |
|
###### # # # # # # # # # # # # # ######
|
||||||
|_| |_| \___/ \__\___/|_|\___/|_| \___|_| |_|
|
# ##### # # # # # # # # ##### # # #
|
||||||
|
# # # # # # # # # # # # # # # # #
|
||||||
|
# # # #### # #### # #### # # #### # #
|
||||||
|
|
||||||
ProtoTorch Core Package
|
ProtoTorch Core Package
|
||||||
"""
|
"""
|
||||||
@ -13,36 +15,43 @@ from setuptools import find_packages, setup
|
|||||||
PROJECT_URL = "https://github.com/si-cim/prototorch"
|
PROJECT_URL = "https://github.com/si-cim/prototorch"
|
||||||
DOWNLOAD_URL = "https://github.com/si-cim/prototorch.git"
|
DOWNLOAD_URL = "https://github.com/si-cim/prototorch.git"
|
||||||
|
|
||||||
with open("README.md", "r") as fh:
|
with open("README.md", encoding="utf-8") as fh:
|
||||||
long_description = fh.read()
|
long_description = fh.read()
|
||||||
|
|
||||||
INSTALL_REQUIRES = [
|
INSTALL_REQUIRES = [
|
||||||
"torch>=1.3.1",
|
"torch>=2.0.0",
|
||||||
"torchvision>=0.5.0",
|
"torchvision",
|
||||||
"numpy>=1.9.1",
|
"numpy",
|
||||||
|
"scikit-learn",
|
||||||
|
"matplotlib",
|
||||||
]
|
]
|
||||||
DATASETS = [
|
DATASETS = [
|
||||||
"requests",
|
"requests",
|
||||||
"tqdm",
|
"tqdm",
|
||||||
]
|
]
|
||||||
DEV = ["bumpversion"]
|
DEV = [
|
||||||
|
"bump2version",
|
||||||
|
"pre-commit",
|
||||||
|
]
|
||||||
DOCS = [
|
DOCS = [
|
||||||
"recommonmark",
|
"recommonmark",
|
||||||
"sphinx",
|
"sphinx",
|
||||||
"sphinx_rtd_theme",
|
"sphinx_rtd_theme",
|
||||||
"sphinxcontrib-katex",
|
"sphinxcontrib-katex",
|
||||||
|
"sphinx-autodoc-typehints",
|
||||||
]
|
]
|
||||||
EXAMPLES = [
|
EXAMPLES = [
|
||||||
"sklearn",
|
|
||||||
"matplotlib",
|
|
||||||
"torchinfo",
|
"torchinfo",
|
||||||
]
|
]
|
||||||
TESTS = ["codecov", "pytest"]
|
TESTS = [
|
||||||
|
"flake8",
|
||||||
|
"pytest",
|
||||||
|
]
|
||||||
ALL = DATASETS + DEV + DOCS + EXAMPLES + TESTS
|
ALL = DATASETS + DEV + DOCS + EXAMPLES + TESTS
|
||||||
|
|
||||||
setup(
|
setup(
|
||||||
name="prototorch",
|
name="prototorch",
|
||||||
version="0.4.2",
|
version="0.7.6",
|
||||||
description="Highly extensible, GPU-supported "
|
description="Highly extensible, GPU-supported "
|
||||||
"Learning Vector Quantization (LVQ) toolbox "
|
"Learning Vector Quantization (LVQ) toolbox "
|
||||||
"built using PyTorch and its nn API.",
|
"built using PyTorch and its nn API.",
|
||||||
@ -53,30 +62,33 @@ setup(
|
|||||||
url=PROJECT_URL,
|
url=PROJECT_URL,
|
||||||
download_url=DOWNLOAD_URL,
|
download_url=DOWNLOAD_URL,
|
||||||
license="MIT",
|
license="MIT",
|
||||||
|
python_requires=">=3.8",
|
||||||
install_requires=INSTALL_REQUIRES,
|
install_requires=INSTALL_REQUIRES,
|
||||||
extras_require={
|
extras_require={
|
||||||
"docs": DOCS,
|
|
||||||
"datasets": DATASETS,
|
"datasets": DATASETS,
|
||||||
|
"dev": DEV,
|
||||||
|
"docs": DOCS,
|
||||||
"examples": EXAMPLES,
|
"examples": EXAMPLES,
|
||||||
"tests": TESTS,
|
"tests": TESTS,
|
||||||
"all": ALL,
|
"all": ALL,
|
||||||
},
|
},
|
||||||
classifiers=[
|
classifiers=[
|
||||||
"Development Status :: 2 - Pre-Alpha",
|
|
||||||
"Environment :: Console",
|
"Environment :: Console",
|
||||||
|
"Natural Language :: English",
|
||||||
|
"Development Status :: 4 - Beta",
|
||||||
"Intended Audience :: Developers",
|
"Intended Audience :: Developers",
|
||||||
"Intended Audience :: Education",
|
"Intended Audience :: Education",
|
||||||
"Intended Audience :: Science/Research",
|
"Intended Audience :: Science/Research",
|
||||||
"License :: OSI Approved :: MIT License",
|
|
||||||
"Natural Language :: English",
|
|
||||||
"Programming Language :: Python :: 3.6",
|
|
||||||
"Programming Language :: Python :: 3.7",
|
|
||||||
"Programming Language :: Python :: 3.8",
|
|
||||||
"Programming Language :: Python :: 3.9",
|
|
||||||
"Operating System :: OS Independent",
|
|
||||||
"Topic :: Scientific/Engineering :: Artificial Intelligence",
|
"Topic :: Scientific/Engineering :: Artificial Intelligence",
|
||||||
"Topic :: Software Development :: Libraries",
|
"Topic :: Software Development :: Libraries",
|
||||||
"Topic :: Software Development :: Libraries :: Python Modules",
|
"Topic :: Software Development :: Libraries :: Python Modules",
|
||||||
|
"License :: OSI Approved :: MIT License",
|
||||||
|
"Operating System :: OS Independent",
|
||||||
|
"Programming Language :: Python :: 3",
|
||||||
|
"Programming Language :: Python :: 3.8",
|
||||||
|
"Programming Language :: Python :: 3.9",
|
||||||
|
"Programming Language :: Python :: 3.10",
|
||||||
|
"Programming Language :: Python :: 3.11",
|
||||||
],
|
],
|
||||||
packages=find_packages(),
|
packages=find_packages(),
|
||||||
zip_safe=False,
|
zip_safe=False,
|
||||||
|
777
tests/test_core.py
Normal file
777
tests/test_core.py
Normal file
@ -0,0 +1,777 @@
|
|||||||
|
"""ProtoTorch core test suite"""
|
||||||
|
|
||||||
|
import unittest
|
||||||
|
|
||||||
|
import numpy as np
|
||||||
|
import pytest
|
||||||
|
import torch
|
||||||
|
|
||||||
|
import prototorch as pt
|
||||||
|
from prototorch.utils import parse_distribution
|
||||||
|
|
||||||
|
|
||||||
|
# Utils
|
||||||
|
def test_parse_distribution_dict_0():
|
||||||
|
distribution = {"num_classes": 1, "per_class": 0}
|
||||||
|
distribution = parse_distribution(distribution)
|
||||||
|
assert distribution == {0: 0}
|
||||||
|
|
||||||
|
|
||||||
|
def test_parse_distribution_dict_1():
|
||||||
|
distribution = dict(num_classes=3, per_class=2)
|
||||||
|
distribution = parse_distribution(distribution)
|
||||||
|
assert distribution == {0: 2, 1: 2, 2: 2}
|
||||||
|
|
||||||
|
|
||||||
|
def test_parse_distribution_dict_2():
|
||||||
|
distribution = {0: 1, 2: 2, -1: 3}
|
||||||
|
distribution = parse_distribution(distribution)
|
||||||
|
assert distribution == {0: 1, 2: 2, -1: 3}
|
||||||
|
|
||||||
|
|
||||||
|
def test_parse_distribution_tuple():
|
||||||
|
distribution = (2, 3)
|
||||||
|
distribution = parse_distribution(distribution)
|
||||||
|
assert distribution == {0: 3, 1: 3}
|
||||||
|
|
||||||
|
|
||||||
|
def test_parse_distribution_list():
|
||||||
|
distribution = [1, 1, 0, 2]
|
||||||
|
distribution = parse_distribution(distribution)
|
||||||
|
assert distribution == {0: 1, 1: 1, 2: 0, 3: 2}
|
||||||
|
|
||||||
|
|
||||||
|
def test_parse_distribution_custom_labels():
|
||||||
|
distribution = [1, 1, 0, 2]
|
||||||
|
clabels = [1, 2, 5, 3]
|
||||||
|
distribution = parse_distribution(distribution, clabels)
|
||||||
|
assert distribution == {1: 1, 2: 1, 5: 0, 3: 2}
|
||||||
|
|
||||||
|
|
||||||
|
# Components initializers
|
||||||
|
def test_literal_comp_generate():
|
||||||
|
protos = torch.rand(4, 3, 5, 5)
|
||||||
|
c = pt.initializers.LiteralCompInitializer(protos)
|
||||||
|
components = c.generate([])
|
||||||
|
assert torch.allclose(components, protos)
|
||||||
|
|
||||||
|
|
||||||
|
def test_literal_comp_generate_from_list():
|
||||||
|
protos = [[0, 1], [2, 3], [4, 5]]
|
||||||
|
c = pt.initializers.LiteralCompInitializer(protos)
|
||||||
|
with pytest.warns(UserWarning):
|
||||||
|
components = c.generate([])
|
||||||
|
assert torch.allclose(components, torch.Tensor(protos))
|
||||||
|
|
||||||
|
|
||||||
|
def test_shape_aware_raises_error():
|
||||||
|
with pytest.raises(TypeError):
|
||||||
|
_ = pt.initializers.ShapeAwareCompInitializer(shape=(2, ))
|
||||||
|
|
||||||
|
|
||||||
|
def test_data_aware_comp_generate():
|
||||||
|
protos = torch.rand(4, 3, 5, 5)
|
||||||
|
c = pt.initializers.DataAwareCompInitializer(protos)
|
||||||
|
components = c.generate(num_components="IgnoreMe!")
|
||||||
|
assert torch.allclose(components, protos)
|
||||||
|
|
||||||
|
|
||||||
|
def test_class_aware_comp_generate():
|
||||||
|
protos = torch.rand(4, 2, 3, 5, 5)
|
||||||
|
plabels = torch.tensor([0, 0, 1, 1]).long()
|
||||||
|
c = pt.initializers.ClassAwareCompInitializer([protos, plabels])
|
||||||
|
components = c.generate(distribution=[])
|
||||||
|
assert torch.allclose(components, protos)
|
||||||
|
|
||||||
|
|
||||||
|
def test_zeros_comp_generate():
|
||||||
|
shape = (3, 5, 5)
|
||||||
|
c = pt.initializers.ZerosCompInitializer(shape)
|
||||||
|
components = c.generate(num_components=4)
|
||||||
|
assert torch.allclose(components, torch.zeros(4, 3, 5, 5))
|
||||||
|
|
||||||
|
|
||||||
|
def test_ones_comp_generate():
|
||||||
|
c = pt.initializers.OnesCompInitializer(2)
|
||||||
|
components = c.generate(num_components=3)
|
||||||
|
assert torch.allclose(components, torch.ones(3, 2))
|
||||||
|
|
||||||
|
|
||||||
|
def test_fill_value_comp_generate():
|
||||||
|
c = pt.initializers.FillValueCompInitializer(2, 0.0)
|
||||||
|
components = c.generate(num_components=3)
|
||||||
|
assert torch.allclose(components, torch.zeros(3, 2))
|
||||||
|
|
||||||
|
|
||||||
|
def test_uniform_comp_generate_min_max_bound():
|
||||||
|
c = pt.initializers.UniformCompInitializer(2, -1.0, 1.0)
|
||||||
|
components = c.generate(num_components=1024)
|
||||||
|
assert components.min() >= -1.0
|
||||||
|
assert components.max() <= 1.0
|
||||||
|
|
||||||
|
|
||||||
|
def test_random_comp_generate_mean():
|
||||||
|
c = pt.initializers.RandomNormalCompInitializer(2, -1.0)
|
||||||
|
components = c.generate(num_components=1024)
|
||||||
|
assert torch.allclose(components.mean(),
|
||||||
|
torch.tensor(-1.0),
|
||||||
|
rtol=1e-05,
|
||||||
|
atol=1e-01)
|
||||||
|
|
||||||
|
|
||||||
|
def test_comp_generate_0_components():
|
||||||
|
c = pt.initializers.ZerosCompInitializer(2)
|
||||||
|
_ = c.generate(num_components=0)
|
||||||
|
|
||||||
|
|
||||||
|
def test_stratified_mean_comp_generate():
|
||||||
|
# yapf: disable
|
||||||
|
x = torch.Tensor(
|
||||||
|
[[0, -1, -2],
|
||||||
|
[10, 11, 12],
|
||||||
|
[0, 0, 0],
|
||||||
|
[2, 2, 2]])
|
||||||
|
y = torch.LongTensor([0, 0, 1, 1])
|
||||||
|
desired = torch.Tensor(
|
||||||
|
[[5.0, 5.0, 5.0],
|
||||||
|
[1.0, 1.0, 1.0]])
|
||||||
|
# yapf: enable
|
||||||
|
c = pt.initializers.StratifiedMeanCompInitializer(data=[x, y])
|
||||||
|
actual = c.generate([1, 1])
|
||||||
|
assert torch.allclose(actual, desired)
|
||||||
|
|
||||||
|
|
||||||
|
def test_stratified_selection_comp_generate():
|
||||||
|
# yapf: disable
|
||||||
|
x = torch.Tensor(
|
||||||
|
[[0, 0, 0],
|
||||||
|
[1, 1, 1],
|
||||||
|
[0, 0, 0],
|
||||||
|
[1, 1, 1]])
|
||||||
|
y = torch.LongTensor([0, 1, 0, 1])
|
||||||
|
desired = torch.Tensor(
|
||||||
|
[[0, 0, 0],
|
||||||
|
[1, 1, 1]])
|
||||||
|
# yapf: enable
|
||||||
|
c = pt.initializers.StratifiedSelectionCompInitializer(data=[x, y])
|
||||||
|
actual = c.generate([1, 1])
|
||||||
|
assert torch.allclose(actual, desired)
|
||||||
|
|
||||||
|
|
||||||
|
# Labels initializers
|
||||||
|
def test_literal_labels_init():
|
||||||
|
l = pt.initializers.LiteralLabelsInitializer([0, 0, 1, 2])
|
||||||
|
with pytest.warns(UserWarning):
|
||||||
|
labels = l.generate([])
|
||||||
|
assert torch.allclose(labels, torch.LongTensor([0, 0, 1, 2]))
|
||||||
|
|
||||||
|
|
||||||
|
def test_labels_init_from_list():
|
||||||
|
l = pt.initializers.LabelsInitializer()
|
||||||
|
components = l.generate(distribution=[1, 1, 1])
|
||||||
|
assert torch.allclose(components, torch.LongTensor([0, 1, 2]))
|
||||||
|
|
||||||
|
|
||||||
|
def test_labels_init_from_tuple_legal():
|
||||||
|
l = pt.initializers.LabelsInitializer()
|
||||||
|
components = l.generate(distribution=(3, 1))
|
||||||
|
assert torch.allclose(components, torch.LongTensor([0, 1, 2]))
|
||||||
|
|
||||||
|
|
||||||
|
def test_labels_init_from_tuple_illegal():
|
||||||
|
l = pt.initializers.LabelsInitializer()
|
||||||
|
with pytest.raises(AssertionError):
|
||||||
|
_ = l.generate(distribution=(1, 1, 1))
|
||||||
|
|
||||||
|
|
||||||
|
def test_data_aware_labels_init():
|
||||||
|
data, targets = [0, 1, 2, 3], [0, 0, 1, 1]
|
||||||
|
ds = pt.datasets.NumpyDataset(data, targets)
|
||||||
|
l = pt.initializers.DataAwareLabelsInitializer(ds)
|
||||||
|
labels = l.generate([])
|
||||||
|
assert torch.allclose(labels, torch.LongTensor(targets))
|
||||||
|
|
||||||
|
|
||||||
|
# Reasonings initializers
|
||||||
|
def test_literal_reasonings_init():
|
||||||
|
r = pt.initializers.LiteralReasoningsInitializer([0, 0, 1, 2])
|
||||||
|
with pytest.warns(UserWarning):
|
||||||
|
reasonings = r.generate([])
|
||||||
|
assert torch.allclose(reasonings, torch.Tensor([0, 0, 1, 2]))
|
||||||
|
|
||||||
|
|
||||||
|
def test_random_reasonings_init():
|
||||||
|
r = pt.initializers.RandomReasoningsInitializer(0.2, 0.8)
|
||||||
|
reasonings = r.generate(distribution=[0, 1])
|
||||||
|
assert torch.numel(reasonings) == 1 * 2 * 2
|
||||||
|
assert reasonings.min() >= 0.2
|
||||||
|
assert reasonings.max() <= 0.8
|
||||||
|
|
||||||
|
|
||||||
|
def test_zeros_reasonings_init():
|
||||||
|
r = pt.initializers.ZerosReasoningsInitializer()
|
||||||
|
reasonings = r.generate(distribution=[0, 1])
|
||||||
|
assert torch.allclose(reasonings, torch.zeros(1, 2, 2))
|
||||||
|
|
||||||
|
|
||||||
|
def test_ones_reasonings_init():
|
||||||
|
r = pt.initializers.ZerosReasoningsInitializer()
|
||||||
|
reasonings = r.generate(distribution=[1, 2, 3])
|
||||||
|
assert torch.allclose(reasonings, torch.zeros(6, 3, 2))
|
||||||
|
|
||||||
|
|
||||||
|
def test_pure_positive_reasonings_init_one_per_class():
|
||||||
|
r = pt.initializers.PurePositiveReasoningsInitializer(
|
||||||
|
components_first=False)
|
||||||
|
reasonings = r.generate(distribution=(4, 1))
|
||||||
|
assert torch.allclose(reasonings[0], torch.eye(4))
|
||||||
|
|
||||||
|
|
||||||
|
def test_pure_positive_reasonings_init_unrepresented_classes():
|
||||||
|
r = pt.initializers.PurePositiveReasoningsInitializer()
|
||||||
|
reasonings = r.generate(distribution=[9, 0, 0, 0])
|
||||||
|
assert reasonings.shape[0] == 9
|
||||||
|
assert reasonings.shape[1] == 4
|
||||||
|
assert reasonings.shape[2] == 2
|
||||||
|
|
||||||
|
|
||||||
|
def test_random_reasonings_init_channels_not_first():
|
||||||
|
r = pt.initializers.RandomReasoningsInitializer(components_first=False)
|
||||||
|
reasonings = r.generate(distribution=[0, 0, 0, 1])
|
||||||
|
assert reasonings.shape[0] == 2
|
||||||
|
assert reasonings.shape[1] == 4
|
||||||
|
assert reasonings.shape[2] == 1
|
||||||
|
|
||||||
|
|
||||||
|
# Transform initializers
|
||||||
|
def test_eye_transform_init_square():
|
||||||
|
t = pt.initializers.EyeLinearTransformInitializer()
|
||||||
|
I = t.generate(3, 3)
|
||||||
|
assert torch.allclose(I, torch.eye(3))
|
||||||
|
|
||||||
|
|
||||||
|
def test_eye_transform_init_narrow():
|
||||||
|
t = pt.initializers.EyeLinearTransformInitializer()
|
||||||
|
actual = t.generate(3, 2)
|
||||||
|
desired = torch.Tensor([[1, 0], [0, 1], [0, 0]])
|
||||||
|
assert torch.allclose(actual, desired)
|
||||||
|
|
||||||
|
|
||||||
|
def test_eye_transform_init_wide():
|
||||||
|
t = pt.initializers.EyeLinearTransformInitializer()
|
||||||
|
actual = t.generate(2, 3)
|
||||||
|
desired = torch.Tensor([[1, 0, 0], [0, 1, 0]])
|
||||||
|
assert torch.allclose(actual, desired)
|
||||||
|
|
||||||
|
|
||||||
|
# Transforms
|
||||||
|
def test_linear_transform_default_eye_init():
|
||||||
|
l = pt.transforms.LinearTransform(2, 4)
|
||||||
|
actual = l.weights
|
||||||
|
desired = torch.Tensor([[1, 0, 0, 0], [0, 1, 0, 0]])
|
||||||
|
assert torch.allclose(actual, desired)
|
||||||
|
|
||||||
|
|
||||||
|
def test_linear_transform_forward():
|
||||||
|
l = pt.transforms.LinearTransform(4, 2)
|
||||||
|
actual_weights = l.weights
|
||||||
|
desired_weights = torch.Tensor([[1, 0], [0, 1], [0, 0], [0, 0]])
|
||||||
|
assert torch.allclose(actual_weights, desired_weights)
|
||||||
|
actual_outputs = l(torch.Tensor([[1.1, 2.2, 3.3, 4.4], \
|
||||||
|
[1.1, 2.2, 3.3, 4.4], \
|
||||||
|
[5.5, 6.6, 7.7, 8.8]]))
|
||||||
|
desired_outputs = torch.Tensor([[1.1, 2.2], [1.1, 2.2], [5.5, 6.6]])
|
||||||
|
assert torch.allclose(actual_outputs, desired_outputs)
|
||||||
|
|
||||||
|
|
||||||
|
def test_linear_transform_zeros_init():
|
||||||
|
l = pt.transforms.LinearTransform(
|
||||||
|
in_dim=2,
|
||||||
|
out_dim=4,
|
||||||
|
initializer=pt.initializers.ZerosLinearTransformInitializer(),
|
||||||
|
)
|
||||||
|
actual = l.weights
|
||||||
|
desired = torch.zeros(2, 4)
|
||||||
|
assert torch.allclose(actual, desired)
|
||||||
|
|
||||||
|
|
||||||
|
def test_linear_transform_out_dim_first():
|
||||||
|
l = pt.transforms.LinearTransform(
|
||||||
|
in_dim=2,
|
||||||
|
out_dim=4,
|
||||||
|
initializer=pt.initializers.OLTI(out_dim_first=True),
|
||||||
|
)
|
||||||
|
assert l.weights.shape[0] == 4
|
||||||
|
assert l.weights.shape[1] == 2
|
||||||
|
|
||||||
|
|
||||||
|
# Components
|
||||||
|
def test_components_no_initializer():
|
||||||
|
with pytest.raises(TypeError):
|
||||||
|
_ = pt.components.Components(3, None)
|
||||||
|
|
||||||
|
|
||||||
|
def test_components_no_num_components():
|
||||||
|
with pytest.raises(TypeError):
|
||||||
|
_ = pt.components.Components(initializer=pt.initializers.OCI(2))
|
||||||
|
|
||||||
|
|
||||||
|
def test_components_none_num_components():
|
||||||
|
with pytest.raises(TypeError):
|
||||||
|
_ = pt.components.Components(None, initializer=pt.initializers.OCI(2))
|
||||||
|
|
||||||
|
|
||||||
|
def test_components_no_args():
|
||||||
|
with pytest.raises(TypeError):
|
||||||
|
_ = pt.components.Components()
|
||||||
|
|
||||||
|
|
||||||
|
def test_components_zeros_init():
|
||||||
|
c = pt.components.Components(3, pt.initializers.ZCI(2))
|
||||||
|
assert torch.allclose(c.components, torch.zeros(3, 2))
|
||||||
|
|
||||||
|
|
||||||
|
def test_labeled_components_dict_init():
|
||||||
|
c = pt.components.LabeledComponents({0: 3}, pt.initializers.OCI(2))
|
||||||
|
assert torch.allclose(c.components, torch.ones(3, 2))
|
||||||
|
assert torch.allclose(c.labels, torch.zeros(3, dtype=torch.long))
|
||||||
|
|
||||||
|
|
||||||
|
def test_labeled_components_list_init():
|
||||||
|
c = pt.components.LabeledComponents([3], pt.initializers.OCI(2))
|
||||||
|
assert torch.allclose(c.components, torch.ones(3, 2))
|
||||||
|
assert torch.allclose(c.labels, torch.zeros(3, dtype=torch.long))
|
||||||
|
|
||||||
|
|
||||||
|
def test_labeled_components_tuple_init():
|
||||||
|
c = pt.components.LabeledComponents({0: 1, 1: 2}, pt.initializers.OCI(2))
|
||||||
|
assert torch.allclose(c.components, torch.ones(3, 2))
|
||||||
|
assert torch.allclose(c.labels, torch.LongTensor([0, 1, 1]))
|
||||||
|
|
||||||
|
|
||||||
|
# Labels
|
||||||
|
def test_standalone_labels_dict_init():
|
||||||
|
l = pt.components.Labels({0: 3})
|
||||||
|
assert torch.allclose(l.labels, torch.zeros(3, dtype=torch.long))
|
||||||
|
|
||||||
|
|
||||||
|
def test_standalone_labels_list_init():
|
||||||
|
l = pt.components.Labels([3])
|
||||||
|
assert torch.allclose(l.labels, torch.zeros(3, dtype=torch.long))
|
||||||
|
|
||||||
|
|
||||||
|
def test_standalone_labels_tuple_init():
|
||||||
|
l = pt.components.Labels({0: 1, 1: 2})
|
||||||
|
assert torch.allclose(l.labels, torch.LongTensor([0, 1, 1]))
|
||||||
|
|
||||||
|
|
||||||
|
# Losses
|
||||||
|
def test_glvq_loss_int_labels():
|
||||||
|
d = torch.stack([torch.ones(100), torch.zeros(100)], dim=1)
|
||||||
|
labels = torch.tensor([0, 1])
|
||||||
|
targets = torch.ones(100)
|
||||||
|
batch_loss = pt.losses.glvq_loss(distances=d,
|
||||||
|
target_labels=targets,
|
||||||
|
prototype_labels=labels)
|
||||||
|
loss_value = torch.sum(batch_loss, dim=0)
|
||||||
|
assert loss_value == -100
|
||||||
|
|
||||||
|
|
||||||
|
def test_glvq_loss_one_hot_labels():
|
||||||
|
d = torch.stack([torch.ones(100), torch.zeros(100)], dim=1)
|
||||||
|
labels = torch.tensor([[0, 1], [1, 0]])
|
||||||
|
wl = torch.tensor([1, 0])
|
||||||
|
targets = torch.stack([wl for _ in range(100)], dim=0)
|
||||||
|
batch_loss = pt.losses.glvq_loss(distances=d,
|
||||||
|
target_labels=targets,
|
||||||
|
prototype_labels=labels)
|
||||||
|
loss_value = torch.sum(batch_loss, dim=0)
|
||||||
|
assert loss_value == -100
|
||||||
|
|
||||||
|
|
||||||
|
def test_glvq_loss_one_hot_unequal():
|
||||||
|
dlist = [torch.ones(100), torch.zeros(100), torch.zeros(100)]
|
||||||
|
d = torch.stack(dlist, dim=1)
|
||||||
|
labels = torch.tensor([[0, 1], [1, 0], [1, 0]])
|
||||||
|
wl = torch.tensor([1, 0])
|
||||||
|
targets = torch.stack([wl for _ in range(100)], dim=0)
|
||||||
|
batch_loss = pt.losses.glvq_loss(distances=d,
|
||||||
|
target_labels=targets,
|
||||||
|
prototype_labels=labels)
|
||||||
|
loss_value = torch.sum(batch_loss, dim=0)
|
||||||
|
assert loss_value == -100
|
||||||
|
|
||||||
|
|
||||||
|
# Activations
|
||||||
|
class TestActivations(unittest.TestCase):
|
||||||
|
|
||||||
|
def setUp(self):
|
||||||
|
self.flist = ["identity", "sigmoid_beta", "swish_beta"]
|
||||||
|
self.x = torch.randn(1024, 1)
|
||||||
|
|
||||||
|
def test_registry(self):
|
||||||
|
self.assertIsNotNone(pt.nn.ACTIVATIONS)
|
||||||
|
|
||||||
|
def test_funcname_deserialization(self):
|
||||||
|
for funcname in self.flist:
|
||||||
|
f = pt.nn.get_activation(funcname)
|
||||||
|
iscallable = callable(f)
|
||||||
|
self.assertTrue(iscallable)
|
||||||
|
|
||||||
|
def test_callable_deserialization(self):
|
||||||
|
|
||||||
|
def dummy(x, **kwargs):
|
||||||
|
return x
|
||||||
|
|
||||||
|
for f in [dummy, lambda x: x]:
|
||||||
|
f = pt.nn.get_activation(f)
|
||||||
|
iscallable = callable(f)
|
||||||
|
self.assertTrue(iscallable)
|
||||||
|
self.assertEqual(1, f(1))
|
||||||
|
|
||||||
|
def test_unknown_deserialization(self):
|
||||||
|
for funcname in ["blubb", "foobar"]:
|
||||||
|
with self.assertRaises(NameError):
|
||||||
|
_ = pt.nn.get_activation(funcname)
|
||||||
|
|
||||||
|
def test_identity(self):
|
||||||
|
actual = pt.nn.identity(self.x)
|
||||||
|
desired = self.x
|
||||||
|
mismatch = np.testing.assert_array_almost_equal(actual,
|
||||||
|
desired,
|
||||||
|
decimal=5)
|
||||||
|
self.assertIsNone(mismatch)
|
||||||
|
|
||||||
|
def test_sigmoid_beta1(self):
|
||||||
|
actual = pt.nn.sigmoid_beta(self.x, beta=1.0)
|
||||||
|
desired = torch.sigmoid(self.x)
|
||||||
|
mismatch = np.testing.assert_array_almost_equal(actual,
|
||||||
|
desired,
|
||||||
|
decimal=5)
|
||||||
|
self.assertIsNone(mismatch)
|
||||||
|
|
||||||
|
def test_swish_beta1(self):
|
||||||
|
actual = pt.nn.swish_beta(self.x, beta=1.0)
|
||||||
|
desired = self.x * torch.sigmoid(self.x)
|
||||||
|
mismatch = np.testing.assert_array_almost_equal(actual,
|
||||||
|
desired,
|
||||||
|
decimal=5)
|
||||||
|
self.assertIsNone(mismatch)
|
||||||
|
|
||||||
|
def tearDown(self):
|
||||||
|
del self.x
|
||||||
|
|
||||||
|
|
||||||
|
# Competitions
|
||||||
|
class TestCompetitions(unittest.TestCase):
|
||||||
|
|
||||||
|
def setUp(self):
|
||||||
|
pass
|
||||||
|
|
||||||
|
def test_wtac(self):
|
||||||
|
d = torch.tensor([[2.0, 3.0, 1.99, 3.01], [2.0, 3.0, 2.01, 3.0]])
|
||||||
|
labels = torch.tensor([0, 1, 2, 3])
|
||||||
|
competition_layer = pt.competitions.WTAC()
|
||||||
|
actual = competition_layer(d, labels)
|
||||||
|
desired = torch.tensor([2, 0])
|
||||||
|
mismatch = np.testing.assert_array_almost_equal(actual,
|
||||||
|
desired,
|
||||||
|
decimal=5)
|
||||||
|
self.assertIsNone(mismatch)
|
||||||
|
|
||||||
|
def test_wtac_unequal_dist(self):
|
||||||
|
d = torch.tensor([[2.0, 3.0, 4.0], [2.0, 3.0, 1.0]])
|
||||||
|
labels = torch.tensor([0, 1, 1])
|
||||||
|
competition_layer = pt.competitions.WTAC()
|
||||||
|
actual = competition_layer(d, labels)
|
||||||
|
desired = torch.tensor([0, 1])
|
||||||
|
mismatch = np.testing.assert_array_almost_equal(actual,
|
||||||
|
desired,
|
||||||
|
decimal=5)
|
||||||
|
self.assertIsNone(mismatch)
|
||||||
|
|
||||||
|
def test_wtac_one_hot(self):
|
||||||
|
d = torch.tensor([[1.99, 3.01], [3.0, 2.01]])
|
||||||
|
labels = torch.tensor([[0, 1], [1, 0]])
|
||||||
|
competition_layer = pt.competitions.WTAC()
|
||||||
|
actual = competition_layer(d, labels)
|
||||||
|
desired = torch.tensor([[0, 1], [1, 0]])
|
||||||
|
mismatch = np.testing.assert_array_almost_equal(actual,
|
||||||
|
desired,
|
||||||
|
decimal=5)
|
||||||
|
self.assertIsNone(mismatch)
|
||||||
|
|
||||||
|
def test_knnc_k1(self):
|
||||||
|
d = torch.tensor([[2.0, 3.0, 1.99, 3.01], [2.0, 3.0, 2.01, 3.0]])
|
||||||
|
labels = torch.tensor([0, 1, 2, 3])
|
||||||
|
competition_layer = pt.competitions.KNNC(k=1)
|
||||||
|
actual = competition_layer(d, labels)
|
||||||
|
desired = torch.tensor([2, 0])
|
||||||
|
mismatch = np.testing.assert_array_almost_equal(actual,
|
||||||
|
desired,
|
||||||
|
decimal=5)
|
||||||
|
self.assertIsNone(mismatch)
|
||||||
|
|
||||||
|
def tearDown(self):
|
||||||
|
pass
|
||||||
|
|
||||||
|
|
||||||
|
# Pooling
|
||||||
|
class TestPooling(unittest.TestCase):
|
||||||
|
|
||||||
|
def setUp(self):
|
||||||
|
pass
|
||||||
|
|
||||||
|
def test_stratified_min(self):
|
||||||
|
d = torch.tensor([[1.0, 0.0, 2.0, 3.0], [9.0, 8.0, 0, 1]])
|
||||||
|
labels = torch.tensor([0, 0, 1, 2])
|
||||||
|
pooling_layer = pt.pooling.StratifiedMinPooling()
|
||||||
|
actual = pooling_layer(d, labels)
|
||||||
|
desired = torch.tensor([[0.0, 2.0, 3.0], [8.0, 0.0, 1.0]])
|
||||||
|
mismatch = np.testing.assert_array_almost_equal(actual,
|
||||||
|
desired,
|
||||||
|
decimal=5)
|
||||||
|
self.assertIsNone(mismatch)
|
||||||
|
|
||||||
|
def test_stratified_min_one_hot(self):
|
||||||
|
d = torch.tensor([[1.0, 0.0, 2.0, 3.0], [9.0, 8.0, 0, 1]])
|
||||||
|
labels = torch.tensor([0, 0, 1, 2])
|
||||||
|
labels = torch.eye(3)[labels]
|
||||||
|
pooling_layer = pt.pooling.StratifiedMinPooling()
|
||||||
|
actual = pooling_layer(d, labels)
|
||||||
|
desired = torch.tensor([[0.0, 2.0, 3.0], [8.0, 0.0, 1.0]])
|
||||||
|
mismatch = np.testing.assert_array_almost_equal(actual,
|
||||||
|
desired,
|
||||||
|
decimal=5)
|
||||||
|
self.assertIsNone(mismatch)
|
||||||
|
|
||||||
|
def test_stratified_min_trivial(self):
|
||||||
|
d = torch.tensor([[0.0, 2.0, 3.0], [8.0, 0, 1]])
|
||||||
|
labels = torch.tensor([0, 1, 2])
|
||||||
|
pooling_layer = pt.pooling.StratifiedMinPooling()
|
||||||
|
actual = pooling_layer(d, labels)
|
||||||
|
desired = torch.tensor([[0.0, 2.0, 3.0], [8.0, 0.0, 1.0]])
|
||||||
|
mismatch = np.testing.assert_array_almost_equal(actual,
|
||||||
|
desired,
|
||||||
|
decimal=5)
|
||||||
|
self.assertIsNone(mismatch)
|
||||||
|
|
||||||
|
def test_stratified_max(self):
|
||||||
|
d = torch.tensor([[1.0, 0.0, 2.0, 3.0, 9.0], [9.0, 8.0, 0, 1, 7.0]])
|
||||||
|
labels = torch.tensor([0, 0, 3, 2, 0])
|
||||||
|
pooling_layer = pt.pooling.StratifiedMaxPooling()
|
||||||
|
actual = pooling_layer(d, labels)
|
||||||
|
desired = torch.tensor([[9.0, 3.0, 2.0], [9.0, 1.0, 0.0]])
|
||||||
|
mismatch = np.testing.assert_array_almost_equal(actual,
|
||||||
|
desired,
|
||||||
|
decimal=5)
|
||||||
|
self.assertIsNone(mismatch)
|
||||||
|
|
||||||
|
def test_stratified_max_one_hot(self):
|
||||||
|
d = torch.tensor([[1.0, 0.0, 2.0, 3.0, 9.0], [9.0, 8.0, 0, 1, 7.0]])
|
||||||
|
labels = torch.tensor([0, 0, 2, 1, 0])
|
||||||
|
labels = torch.nn.functional.one_hot(labels, num_classes=3)
|
||||||
|
pooling_layer = pt.pooling.StratifiedMaxPooling()
|
||||||
|
actual = pooling_layer(d, labels)
|
||||||
|
desired = torch.tensor([[9.0, 3.0, 2.0], [9.0, 1.0, 0.0]])
|
||||||
|
mismatch = np.testing.assert_array_almost_equal(actual,
|
||||||
|
desired,
|
||||||
|
decimal=5)
|
||||||
|
self.assertIsNone(mismatch)
|
||||||
|
|
||||||
|
def test_stratified_sum(self):
|
||||||
|
d = torch.tensor([[1.0, 0.0, 2.0, 3.0], [9.0, 8.0, 0, 1]])
|
||||||
|
labels = torch.LongTensor([0, 0, 1, 2])
|
||||||
|
pooling_layer = pt.pooling.StratifiedSumPooling()
|
||||||
|
actual = pooling_layer(d, labels)
|
||||||
|
desired = torch.tensor([[1.0, 2.0, 3.0], [17.0, 0.0, 1.0]])
|
||||||
|
mismatch = np.testing.assert_array_almost_equal(actual,
|
||||||
|
desired,
|
||||||
|
decimal=5)
|
||||||
|
self.assertIsNone(mismatch)
|
||||||
|
|
||||||
|
def test_stratified_sum_one_hot(self):
|
||||||
|
d = torch.tensor([[1.0, 0.0, 2.0, 3.0], [9.0, 8.0, 0, 1]])
|
||||||
|
labels = torch.tensor([0, 0, 1, 2])
|
||||||
|
labels = torch.eye(3)[labels]
|
||||||
|
pooling_layer = pt.pooling.StratifiedSumPooling()
|
||||||
|
actual = pooling_layer(d, labels)
|
||||||
|
desired = torch.tensor([[1.0, 2.0, 3.0], [17.0, 0.0, 1.0]])
|
||||||
|
mismatch = np.testing.assert_array_almost_equal(actual,
|
||||||
|
desired,
|
||||||
|
decimal=5)
|
||||||
|
self.assertIsNone(mismatch)
|
||||||
|
|
||||||
|
def test_stratified_prod(self):
|
||||||
|
d = torch.tensor([[1.0, 0.0, 2.0, 3.0, 9.0], [9.0, 8.0, 0, 1, 7.0]])
|
||||||
|
labels = torch.tensor([0, 0, 3, 2, 0])
|
||||||
|
pooling_layer = pt.pooling.StratifiedProdPooling()
|
||||||
|
actual = pooling_layer(d, labels)
|
||||||
|
desired = torch.tensor([[0.0, 3.0, 2.0], [504.0, 1.0, 0.0]])
|
||||||
|
mismatch = np.testing.assert_array_almost_equal(actual,
|
||||||
|
desired,
|
||||||
|
decimal=5)
|
||||||
|
self.assertIsNone(mismatch)
|
||||||
|
|
||||||
|
def tearDown(self):
|
||||||
|
pass
|
||||||
|
|
||||||
|
|
||||||
|
# Distances
|
||||||
|
class TestDistances(unittest.TestCase):
|
||||||
|
|
||||||
|
def setUp(self):
|
||||||
|
self.nx, self.mx = 32, 2048
|
||||||
|
self.ny, self.my = 8, 2048
|
||||||
|
self.x = torch.randn(self.nx, self.mx)
|
||||||
|
self.y = torch.randn(self.ny, self.my)
|
||||||
|
|
||||||
|
def test_manhattan(self):
|
||||||
|
actual = pt.distances.lpnorm_distance(self.x, self.y, p=1)
|
||||||
|
desired = torch.empty(self.nx, self.ny)
|
||||||
|
for i in range(self.nx):
|
||||||
|
for j in range(self.ny):
|
||||||
|
desired[i][j] = torch.nn.functional.pairwise_distance(
|
||||||
|
self.x[i].reshape(1, -1),
|
||||||
|
self.y[j].reshape(1, -1),
|
||||||
|
p=1,
|
||||||
|
keepdim=False,
|
||||||
|
)
|
||||||
|
mismatch = np.testing.assert_array_almost_equal(actual,
|
||||||
|
desired,
|
||||||
|
decimal=2)
|
||||||
|
self.assertIsNone(mismatch)
|
||||||
|
|
||||||
|
def test_euclidean(self):
|
||||||
|
actual = pt.distances.euclidean_distance(self.x, self.y)
|
||||||
|
desired = torch.empty(self.nx, self.ny)
|
||||||
|
for i in range(self.nx):
|
||||||
|
for j in range(self.ny):
|
||||||
|
desired[i][j] = torch.nn.functional.pairwise_distance(
|
||||||
|
self.x[i].reshape(1, -1),
|
||||||
|
self.y[j].reshape(1, -1),
|
||||||
|
p=2,
|
||||||
|
keepdim=False,
|
||||||
|
)
|
||||||
|
mismatch = np.testing.assert_array_almost_equal(actual,
|
||||||
|
desired,
|
||||||
|
decimal=3)
|
||||||
|
self.assertIsNone(mismatch)
|
||||||
|
|
||||||
|
def test_squared_euclidean(self):
|
||||||
|
actual = pt.distances.squared_euclidean_distance(self.x, self.y)
|
||||||
|
desired = torch.empty(self.nx, self.ny)
|
||||||
|
for i in range(self.nx):
|
||||||
|
for j in range(self.ny):
|
||||||
|
desired[i][j] = (torch.nn.functional.pairwise_distance(
|
||||||
|
self.x[i].reshape(1, -1),
|
||||||
|
self.y[j].reshape(1, -1),
|
||||||
|
p=2,
|
||||||
|
keepdim=False,
|
||||||
|
)**2)
|
||||||
|
mismatch = np.testing.assert_array_almost_equal(actual,
|
||||||
|
desired,
|
||||||
|
decimal=2)
|
||||||
|
self.assertIsNone(mismatch)
|
||||||
|
|
||||||
|
def test_lpnorm_p0(self):
|
||||||
|
actual = pt.distances.lpnorm_distance(self.x, self.y, p=0)
|
||||||
|
desired = torch.empty(self.nx, self.ny)
|
||||||
|
for i in range(self.nx):
|
||||||
|
for j in range(self.ny):
|
||||||
|
desired[i][j] = torch.nn.functional.pairwise_distance(
|
||||||
|
self.x[i].reshape(1, -1),
|
||||||
|
self.y[j].reshape(1, -1),
|
||||||
|
p=0,
|
||||||
|
keepdim=False,
|
||||||
|
)
|
||||||
|
mismatch = np.testing.assert_array_almost_equal(actual,
|
||||||
|
desired,
|
||||||
|
decimal=4)
|
||||||
|
self.assertIsNone(mismatch)
|
||||||
|
|
||||||
|
def test_lpnorm_p2(self):
|
||||||
|
actual = pt.distances.lpnorm_distance(self.x, self.y, p=2)
|
||||||
|
desired = torch.empty(self.nx, self.ny)
|
||||||
|
for i in range(self.nx):
|
||||||
|
for j in range(self.ny):
|
||||||
|
desired[i][j] = torch.nn.functional.pairwise_distance(
|
||||||
|
self.x[i].reshape(1, -1),
|
||||||
|
self.y[j].reshape(1, -1),
|
||||||
|
p=2,
|
||||||
|
keepdim=False,
|
||||||
|
)
|
||||||
|
mismatch = np.testing.assert_array_almost_equal(actual,
|
||||||
|
desired,
|
||||||
|
decimal=4)
|
||||||
|
self.assertIsNone(mismatch)
|
||||||
|
|
||||||
|
def test_lpnorm_p3(self):
|
||||||
|
actual = pt.distances.lpnorm_distance(self.x, self.y, p=3)
|
||||||
|
desired = torch.empty(self.nx, self.ny)
|
||||||
|
for i in range(self.nx):
|
||||||
|
for j in range(self.ny):
|
||||||
|
desired[i][j] = torch.nn.functional.pairwise_distance(
|
||||||
|
self.x[i].reshape(1, -1),
|
||||||
|
self.y[j].reshape(1, -1),
|
||||||
|
p=3,
|
||||||
|
keepdim=False,
|
||||||
|
)
|
||||||
|
mismatch = np.testing.assert_array_almost_equal(actual,
|
||||||
|
desired,
|
||||||
|
decimal=4)
|
||||||
|
self.assertIsNone(mismatch)
|
||||||
|
|
||||||
|
def test_lpnorm_pinf(self):
|
||||||
|
actual = pt.distances.lpnorm_distance(self.x, self.y, p=float("inf"))
|
||||||
|
desired = torch.empty(self.nx, self.ny)
|
||||||
|
for i in range(self.nx):
|
||||||
|
for j in range(self.ny):
|
||||||
|
desired[i][j] = torch.nn.functional.pairwise_distance(
|
||||||
|
self.x[i].reshape(1, -1),
|
||||||
|
self.y[j].reshape(1, -1),
|
||||||
|
p=float("inf"),
|
||||||
|
keepdim=False,
|
||||||
|
)
|
||||||
|
mismatch = np.testing.assert_array_almost_equal(actual,
|
||||||
|
desired,
|
||||||
|
decimal=4)
|
||||||
|
self.assertIsNone(mismatch)
|
||||||
|
|
||||||
|
def test_omega_identity(self):
|
||||||
|
omega = torch.eye(self.mx, self.my)
|
||||||
|
actual = pt.distances.omega_distance(self.x, self.y, omega=omega)
|
||||||
|
desired = torch.empty(self.nx, self.ny)
|
||||||
|
for i in range(self.nx):
|
||||||
|
for j in range(self.ny):
|
||||||
|
desired[i][j] = (torch.nn.functional.pairwise_distance(
|
||||||
|
self.x[i].reshape(1, -1),
|
||||||
|
self.y[j].reshape(1, -1),
|
||||||
|
p=2,
|
||||||
|
keepdim=False,
|
||||||
|
)**2)
|
||||||
|
mismatch = np.testing.assert_array_almost_equal(actual,
|
||||||
|
desired,
|
||||||
|
decimal=2)
|
||||||
|
self.assertIsNone(mismatch)
|
||||||
|
|
||||||
|
def test_lomega_identity(self):
|
||||||
|
omega = torch.eye(self.mx, self.my)
|
||||||
|
omegas = torch.stack([omega for _ in range(self.ny)], dim=0)
|
||||||
|
actual = pt.distances.lomega_distance(self.x, self.y, omegas=omegas)
|
||||||
|
desired = torch.empty(self.nx, self.ny)
|
||||||
|
for i in range(self.nx):
|
||||||
|
for j in range(self.ny):
|
||||||
|
desired[i][j] = (torch.nn.functional.pairwise_distance(
|
||||||
|
self.x[i].reshape(1, -1),
|
||||||
|
self.y[j].reshape(1, -1),
|
||||||
|
p=2,
|
||||||
|
keepdim=False,
|
||||||
|
)**2)
|
||||||
|
mismatch = np.testing.assert_array_almost_equal(actual,
|
||||||
|
desired,
|
||||||
|
decimal=2)
|
||||||
|
self.assertIsNone(mismatch)
|
||||||
|
|
||||||
|
def tearDown(self):
|
||||||
|
del self.x, self.y
|
@ -1,95 +1,186 @@
|
|||||||
"""ProtoTorch datasets test suite."""
|
"""ProtoTorch datasets test suite"""
|
||||||
|
|
||||||
import os
|
import os
|
||||||
import shutil
|
|
||||||
import unittest
|
import unittest
|
||||||
|
|
||||||
|
import numpy as np
|
||||||
import torch
|
import torch
|
||||||
|
|
||||||
from prototorch.datasets import abstract, tecator
|
import prototorch as pt
|
||||||
|
from prototorch.datasets.abstract import Dataset, ProtoDataset
|
||||||
|
|
||||||
|
|
||||||
class TestAbstract(unittest.TestCase):
|
class TestAbstract(unittest.TestCase):
|
||||||
|
|
||||||
|
def setUp(self):
|
||||||
|
self.ds = Dataset("./artifacts")
|
||||||
|
|
||||||
def test_getitem(self):
|
def test_getitem(self):
|
||||||
with self.assertRaises(NotImplementedError):
|
with self.assertRaises(NotImplementedError):
|
||||||
abstract.Dataset("./artifacts")[0]
|
_ = self.ds[0]
|
||||||
|
|
||||||
def test_len(self):
|
def test_len(self):
|
||||||
with self.assertRaises(NotImplementedError):
|
with self.assertRaises(NotImplementedError):
|
||||||
len(abstract.Dataset("./artifacts"))
|
_ = len(self.ds)
|
||||||
|
|
||||||
|
def tearDown(self):
|
||||||
|
del self.ds
|
||||||
|
|
||||||
|
|
||||||
class TestProtoDataset(unittest.TestCase):
|
class TestProtoDataset(unittest.TestCase):
|
||||||
def test_getitem(self):
|
|
||||||
with self.assertRaises(NotImplementedError):
|
|
||||||
abstract.ProtoDataset("./artifacts")[0]
|
|
||||||
|
|
||||||
def test_download(self):
|
def test_download(self):
|
||||||
with self.assertRaises(NotImplementedError):
|
with self.assertRaises(NotImplementedError):
|
||||||
abstract.ProtoDataset("./artifacts").download()
|
_ = ProtoDataset("./artifacts", download=True)
|
||||||
|
|
||||||
|
def test_exists(self):
|
||||||
class TestTecator(unittest.TestCase):
|
|
||||||
def setUp(self):
|
|
||||||
self.artifacts_dir = "./artifacts/Tecator"
|
|
||||||
self._remove_artifacts()
|
|
||||||
|
|
||||||
def _remove_artifacts(self):
|
|
||||||
if os.path.exists(self.artifacts_dir):
|
|
||||||
shutil.rmtree(self.artifacts_dir)
|
|
||||||
|
|
||||||
def test_download_false(self):
|
|
||||||
rootdir = self.artifacts_dir.rpartition("/")[0]
|
|
||||||
self._remove_artifacts()
|
|
||||||
with self.assertRaises(RuntimeError):
|
with self.assertRaises(RuntimeError):
|
||||||
_ = tecator.Tecator(rootdir, download=False)
|
_ = ProtoDataset("./artifacts", download=False)
|
||||||
|
|
||||||
def test_download_caching(self):
|
|
||||||
rootdir = self.artifacts_dir.rpartition("/")[0]
|
|
||||||
_ = tecator.Tecator(rootdir, download=True, verbose=False)
|
|
||||||
_ = tecator.Tecator(rootdir, download=False, verbose=False)
|
|
||||||
|
|
||||||
def test_repr(self):
|
class TestNumpyDataset(unittest.TestCase):
|
||||||
rootdir = self.artifacts_dir.rpartition("/")[0]
|
|
||||||
train = tecator.Tecator(rootdir, download=True, verbose=True)
|
|
||||||
self.assertTrue("Split: Train" in train.__repr__())
|
|
||||||
|
|
||||||
def test_download_train(self):
|
def test_list_init(self):
|
||||||
rootdir = self.artifacts_dir.rpartition("/")[0]
|
ds = pt.datasets.NumpyDataset([1], [1])
|
||||||
train = tecator.Tecator(root=rootdir,
|
self.assertEqual(len(ds), 1)
|
||||||
train=True,
|
|
||||||
download=True,
|
|
||||||
verbose=False)
|
|
||||||
train = tecator.Tecator(root=rootdir, download=True, verbose=False)
|
|
||||||
x_train, y_train = train.data, train.targets
|
|
||||||
self.assertEqual(x_train.shape[0], 144)
|
|
||||||
self.assertEqual(y_train.shape[0], 144)
|
|
||||||
self.assertEqual(x_train.shape[1], 100)
|
|
||||||
|
|
||||||
def test_download_test(self):
|
def test_numpy_init(self):
|
||||||
rootdir = self.artifacts_dir.rpartition("/")[0]
|
data = np.random.randn(3, 2)
|
||||||
test = tecator.Tecator(root=rootdir, train=False, verbose=False)
|
targets = np.array([0, 1, 2])
|
||||||
x_test, y_test = test.data, test.targets
|
ds = pt.datasets.NumpyDataset(data, targets)
|
||||||
self.assertEqual(x_test.shape[0], 71)
|
self.assertEqual(len(ds), 3)
|
||||||
self.assertEqual(y_test.shape[0], 71)
|
|
||||||
self.assertEqual(x_test.shape[1], 100)
|
|
||||||
|
|
||||||
def test_class_to_idx(self):
|
|
||||||
rootdir = self.artifacts_dir.rpartition("/")[0]
|
|
||||||
test = tecator.Tecator(root=rootdir, train=False, verbose=False)
|
|
||||||
_ = test.class_to_idx
|
|
||||||
|
|
||||||
def test_getitem(self):
|
class TestCSVDataset(unittest.TestCase):
|
||||||
rootdir = self.artifacts_dir.rpartition("/")[0]
|
|
||||||
test = tecator.Tecator(root=rootdir, train=False, verbose=False)
|
|
||||||
x, y = test[0]
|
|
||||||
self.assertEqual(x.shape[0], 100)
|
|
||||||
self.assertIsInstance(y, int)
|
|
||||||
|
|
||||||
def test_loadable_with_dataloader(self):
|
def setUp(self):
|
||||||
rootdir = self.artifacts_dir.rpartition("/")[0]
|
data = np.random.rand(100, 4)
|
||||||
test = tecator.Tecator(root=rootdir, train=False, verbose=False)
|
targets = np.random.randint(2, size=(100, 1))
|
||||||
_ = torch.utils.data.DataLoader(test, batch_size=64, shuffle=True)
|
arr = np.hstack([data, targets])
|
||||||
|
if not os.path.exists("./artifacts"):
|
||||||
|
os.mkdir("./artifacts")
|
||||||
|
np.savetxt("./artifacts/test.csv", arr, delimiter=",")
|
||||||
|
|
||||||
|
def test_len(self):
|
||||||
|
ds = pt.datasets.CSVDataset("./artifacts/test.csv")
|
||||||
|
self.assertEqual(len(ds), 100)
|
||||||
|
|
||||||
def tearDown(self):
|
def tearDown(self):
|
||||||
pass
|
os.remove("./artifacts/test.csv")
|
||||||
|
|
||||||
|
|
||||||
|
class TestSpiral(unittest.TestCase):
|
||||||
|
|
||||||
|
def test_init(self):
|
||||||
|
ds = pt.datasets.Spiral(num_samples=10)
|
||||||
|
self.assertEqual(len(ds), 10)
|
||||||
|
|
||||||
|
|
||||||
|
class TestIris(unittest.TestCase):
|
||||||
|
|
||||||
|
def setUp(self):
|
||||||
|
self.ds = pt.datasets.Iris()
|
||||||
|
|
||||||
|
def test_size(self):
|
||||||
|
self.assertEqual(len(self.ds), 150)
|
||||||
|
|
||||||
|
def test_dims(self):
|
||||||
|
self.assertEqual(self.ds.data.shape[1], 4)
|
||||||
|
|
||||||
|
def test_dims_selection(self):
|
||||||
|
ds = pt.datasets.Iris(dims=[0, 1])
|
||||||
|
self.assertEqual(ds.data.shape[1], 2)
|
||||||
|
|
||||||
|
|
||||||
|
class TestBlobs(unittest.TestCase):
|
||||||
|
|
||||||
|
def test_size(self):
|
||||||
|
ds = pt.datasets.Blobs(num_samples=10)
|
||||||
|
self.assertEqual(len(ds), 10)
|
||||||
|
|
||||||
|
|
||||||
|
class TestRandom(unittest.TestCase):
|
||||||
|
|
||||||
|
def test_size(self):
|
||||||
|
ds = pt.datasets.Random(num_samples=10)
|
||||||
|
self.assertEqual(len(ds), 10)
|
||||||
|
|
||||||
|
|
||||||
|
class TestCircles(unittest.TestCase):
|
||||||
|
|
||||||
|
def test_size(self):
|
||||||
|
ds = pt.datasets.Circles(num_samples=10)
|
||||||
|
self.assertEqual(len(ds), 10)
|
||||||
|
|
||||||
|
|
||||||
|
class TestMoons(unittest.TestCase):
|
||||||
|
|
||||||
|
def test_size(self):
|
||||||
|
ds = pt.datasets.Moons(num_samples=10)
|
||||||
|
self.assertEqual(len(ds), 10)
|
||||||
|
|
||||||
|
|
||||||
|
# class TestTecator(unittest.TestCase):
|
||||||
|
# def setUp(self):
|
||||||
|
# self.artifacts_dir = "./artifacts/Tecator"
|
||||||
|
# self._remove_artifacts()
|
||||||
|
|
||||||
|
# def _remove_artifacts(self):
|
||||||
|
# if os.path.exists(self.artifacts_dir):
|
||||||
|
# shutil.rmtree(self.artifacts_dir)
|
||||||
|
|
||||||
|
# def test_download_false(self):
|
||||||
|
# rootdir = self.artifacts_dir.rpartition("/")[0]
|
||||||
|
# self._remove_artifacts()
|
||||||
|
# with self.assertRaises(RuntimeError):
|
||||||
|
# _ = pt.datasets.Tecator(rootdir, download=False)
|
||||||
|
|
||||||
|
# def test_download_caching(self):
|
||||||
|
# rootdir = self.artifacts_dir.rpartition("/")[0]
|
||||||
|
# _ = pt.datasets.Tecator(rootdir, download=True, verbose=False)
|
||||||
|
# _ = pt.datasets.Tecator(rootdir, download=False, verbose=False)
|
||||||
|
|
||||||
|
# def test_repr(self):
|
||||||
|
# rootdir = self.artifacts_dir.rpartition("/")[0]
|
||||||
|
# train = pt.datasets.Tecator(rootdir, download=True, verbose=True)
|
||||||
|
# self.assertTrue("Split: Train" in train.__repr__())
|
||||||
|
|
||||||
|
# def test_download_train(self):
|
||||||
|
# rootdir = self.artifacts_dir.rpartition("/")[0]
|
||||||
|
# train = pt.datasets.Tecator(root=rootdir,
|
||||||
|
# train=True,
|
||||||
|
# download=True,
|
||||||
|
# verbose=False)
|
||||||
|
# train = pt.datasets.Tecator(root=rootdir, download=True, verbose=False)
|
||||||
|
# x_train, y_train = train.data, train.targets
|
||||||
|
# self.assertEqual(x_train.shape[0], 144)
|
||||||
|
# self.assertEqual(y_train.shape[0], 144)
|
||||||
|
# self.assertEqual(x_train.shape[1], 100)
|
||||||
|
|
||||||
|
# def test_download_test(self):
|
||||||
|
# rootdir = self.artifacts_dir.rpartition("/")[0]
|
||||||
|
# test = pt.datasets.Tecator(root=rootdir, train=False, verbose=False)
|
||||||
|
# x_test, y_test = test.data, test.targets
|
||||||
|
# self.assertEqual(x_test.shape[0], 71)
|
||||||
|
# self.assertEqual(y_test.shape[0], 71)
|
||||||
|
# self.assertEqual(x_test.shape[1], 100)
|
||||||
|
|
||||||
|
# def test_class_to_idx(self):
|
||||||
|
# rootdir = self.artifacts_dir.rpartition("/")[0]
|
||||||
|
# test = pt.datasets.Tecator(root=rootdir, train=False, verbose=False)
|
||||||
|
# _ = test.class_to_idx
|
||||||
|
|
||||||
|
# def test_getitem(self):
|
||||||
|
# rootdir = self.artifacts_dir.rpartition("/")[0]
|
||||||
|
# test = pt.datasets.Tecator(root=rootdir, train=False, verbose=False)
|
||||||
|
# x, y = test[0]
|
||||||
|
# self.assertEqual(x.shape[0], 100)
|
||||||
|
# self.assertIsInstance(y, int)
|
||||||
|
|
||||||
|
# def test_loadable_with_dataloader(self):
|
||||||
|
# rootdir = self.artifacts_dir.rpartition("/")[0]
|
||||||
|
# test = pt.datasets.Tecator(root=rootdir, train=False, verbose=False)
|
||||||
|
# _ = torch.utils.data.DataLoader(test, batch_size=64, shuffle=True)
|
||||||
|
|
||||||
|
# def tearDown(self):
|
||||||
|
# self._remove_artifacts()
|
||||||
|
@ -1,521 +0,0 @@
|
|||||||
"""ProtoTorch functions test suite."""
|
|
||||||
|
|
||||||
import unittest
|
|
||||||
|
|
||||||
import numpy as np
|
|
||||||
import torch
|
|
||||||
|
|
||||||
from prototorch.functions import (activations, competitions, distances,
|
|
||||||
initializers, losses)
|
|
||||||
|
|
||||||
|
|
||||||
class TestActivations(unittest.TestCase):
|
|
||||||
def setUp(self):
|
|
||||||
self.flist = ["identity", "sigmoid_beta", "swish_beta"]
|
|
||||||
self.x = torch.randn(1024, 1)
|
|
||||||
|
|
||||||
def test_registry(self):
|
|
||||||
self.assertIsNotNone(activations.ACTIVATIONS)
|
|
||||||
|
|
||||||
def test_funcname_deserialization(self):
|
|
||||||
for funcname in self.flist:
|
|
||||||
f = activations.get_activation(funcname)
|
|
||||||
iscallable = callable(f)
|
|
||||||
self.assertTrue(iscallable)
|
|
||||||
|
|
||||||
# def test_torch_script(self):
|
|
||||||
# for funcname in self.flist:
|
|
||||||
# f = activations.get_activation(funcname)
|
|
||||||
# self.assertIsInstance(f, torch.jit.ScriptFunction)
|
|
||||||
|
|
||||||
def test_callable_deserialization(self):
|
|
||||||
def dummy(x, **kwargs):
|
|
||||||
return x
|
|
||||||
|
|
||||||
for f in [dummy, lambda x: x]:
|
|
||||||
f = activations.get_activation(f)
|
|
||||||
iscallable = callable(f)
|
|
||||||
self.assertTrue(iscallable)
|
|
||||||
self.assertEqual(1, f(1))
|
|
||||||
|
|
||||||
def test_unknown_deserialization(self):
|
|
||||||
for funcname in ["blubb", "foobar"]:
|
|
||||||
with self.assertRaises(NameError):
|
|
||||||
_ = activations.get_activation(funcname)
|
|
||||||
|
|
||||||
def test_identity(self):
|
|
||||||
actual = activations.identity(self.x)
|
|
||||||
desired = self.x
|
|
||||||
mismatch = np.testing.assert_array_almost_equal(actual,
|
|
||||||
desired,
|
|
||||||
decimal=5)
|
|
||||||
self.assertIsNone(mismatch)
|
|
||||||
|
|
||||||
def test_sigmoid_beta1(self):
|
|
||||||
actual = activations.sigmoid_beta(self.x, beta=1.0)
|
|
||||||
desired = torch.sigmoid(self.x)
|
|
||||||
mismatch = np.testing.assert_array_almost_equal(actual,
|
|
||||||
desired,
|
|
||||||
decimal=5)
|
|
||||||
self.assertIsNone(mismatch)
|
|
||||||
|
|
||||||
def test_swish_beta1(self):
|
|
||||||
actual = activations.swish_beta(self.x, beta=1.0)
|
|
||||||
desired = self.x * torch.sigmoid(self.x)
|
|
||||||
mismatch = np.testing.assert_array_almost_equal(actual,
|
|
||||||
desired,
|
|
||||||
decimal=5)
|
|
||||||
self.assertIsNone(mismatch)
|
|
||||||
|
|
||||||
def tearDown(self):
|
|
||||||
del self.x
|
|
||||||
|
|
||||||
|
|
||||||
class TestCompetitions(unittest.TestCase):
|
|
||||||
def setUp(self):
|
|
||||||
pass
|
|
||||||
|
|
||||||
def test_wtac(self):
|
|
||||||
d = torch.tensor([[2.0, 3.0, 1.99, 3.01], [2.0, 3.0, 2.01, 3.0]])
|
|
||||||
labels = torch.tensor([0, 1, 2, 3])
|
|
||||||
actual = competitions.wtac(d, labels)
|
|
||||||
desired = torch.tensor([2, 0])
|
|
||||||
mismatch = np.testing.assert_array_almost_equal(actual,
|
|
||||||
desired,
|
|
||||||
decimal=5)
|
|
||||||
self.assertIsNone(mismatch)
|
|
||||||
|
|
||||||
def test_wtac_unequal_dist(self):
|
|
||||||
d = torch.tensor([[2.0, 3.0, 4.0], [2.0, 3.0, 1.0]])
|
|
||||||
labels = torch.tensor([0, 1, 1])
|
|
||||||
actual = competitions.wtac(d, labels)
|
|
||||||
desired = torch.tensor([0, 1])
|
|
||||||
mismatch = np.testing.assert_array_almost_equal(actual,
|
|
||||||
desired,
|
|
||||||
decimal=5)
|
|
||||||
self.assertIsNone(mismatch)
|
|
||||||
|
|
||||||
def test_wtac_one_hot(self):
|
|
||||||
d = torch.tensor([[1.99, 3.01], [3.0, 2.01]])
|
|
||||||
labels = torch.tensor([[0, 1], [1, 0]])
|
|
||||||
actual = competitions.wtac(d, labels)
|
|
||||||
desired = torch.tensor([[0, 1], [1, 0]])
|
|
||||||
mismatch = np.testing.assert_array_almost_equal(actual,
|
|
||||||
desired,
|
|
||||||
decimal=5)
|
|
||||||
self.assertIsNone(mismatch)
|
|
||||||
|
|
||||||
def test_stratified_min(self):
|
|
||||||
d = torch.tensor([[1.0, 0.0, 2.0, 3.0], [9.0, 8.0, 0, 1]])
|
|
||||||
labels = torch.tensor([0, 0, 1, 2])
|
|
||||||
actual = competitions.stratified_min(d, labels)
|
|
||||||
desired = torch.tensor([[0.0, 2.0, 3.0], [8.0, 0.0, 1.0]])
|
|
||||||
mismatch = np.testing.assert_array_almost_equal(actual,
|
|
||||||
desired,
|
|
||||||
decimal=5)
|
|
||||||
self.assertIsNone(mismatch)
|
|
||||||
|
|
||||||
def test_stratified_min_one_hot(self):
|
|
||||||
d = torch.tensor([[1.0, 0.0, 2.0, 3.0], [9.0, 8.0, 0, 1]])
|
|
||||||
labels = torch.tensor([0, 0, 1, 2])
|
|
||||||
labels = torch.eye(3)[labels]
|
|
||||||
actual = competitions.stratified_min(d, labels)
|
|
||||||
desired = torch.tensor([[0.0, 2.0, 3.0], [8.0, 0.0, 1.0]])
|
|
||||||
mismatch = np.testing.assert_array_almost_equal(actual,
|
|
||||||
desired,
|
|
||||||
decimal=5)
|
|
||||||
self.assertIsNone(mismatch)
|
|
||||||
|
|
||||||
def test_stratified_min_simple(self):
|
|
||||||
d = torch.tensor([[0.0, 2.0, 3.0], [8.0, 0, 1]])
|
|
||||||
labels = torch.tensor([0, 1, 2])
|
|
||||||
actual = competitions.stratified_min(d, labels)
|
|
||||||
desired = torch.tensor([[0.0, 2.0, 3.0], [8.0, 0.0, 1.0]])
|
|
||||||
mismatch = np.testing.assert_array_almost_equal(actual,
|
|
||||||
desired,
|
|
||||||
decimal=5)
|
|
||||||
self.assertIsNone(mismatch)
|
|
||||||
|
|
||||||
def test_knnc_k1(self):
|
|
||||||
d = torch.tensor([[2.0, 3.0, 1.99, 3.01], [2.0, 3.0, 2.01, 3.0]])
|
|
||||||
labels = torch.tensor([0, 1, 2, 3])
|
|
||||||
actual = competitions.knnc(d, labels, k=torch.tensor([1]))
|
|
||||||
desired = torch.tensor([2, 0])
|
|
||||||
mismatch = np.testing.assert_array_almost_equal(actual,
|
|
||||||
desired,
|
|
||||||
decimal=5)
|
|
||||||
self.assertIsNone(mismatch)
|
|
||||||
|
|
||||||
def tearDown(self):
|
|
||||||
pass
|
|
||||||
|
|
||||||
|
|
||||||
class TestDistances(unittest.TestCase):
|
|
||||||
def setUp(self):
|
|
||||||
self.nx, self.mx = 32, 2048
|
|
||||||
self.ny, self.my = 8, 2048
|
|
||||||
self.x = torch.randn(self.nx, self.mx)
|
|
||||||
self.y = torch.randn(self.ny, self.my)
|
|
||||||
|
|
||||||
def test_manhattan(self):
|
|
||||||
actual = distances.lpnorm_distance(self.x, self.y, p=1)
|
|
||||||
desired = torch.empty(self.nx, self.ny)
|
|
||||||
for i in range(self.nx):
|
|
||||||
for j in range(self.ny):
|
|
||||||
desired[i][j] = torch.nn.functional.pairwise_distance(
|
|
||||||
self.x[i].reshape(1, -1),
|
|
||||||
self.y[j].reshape(1, -1),
|
|
||||||
p=1,
|
|
||||||
keepdim=False,
|
|
||||||
)
|
|
||||||
mismatch = np.testing.assert_array_almost_equal(actual,
|
|
||||||
desired,
|
|
||||||
decimal=2)
|
|
||||||
self.assertIsNone(mismatch)
|
|
||||||
|
|
||||||
def test_euclidean(self):
|
|
||||||
actual = distances.euclidean_distance(self.x, self.y)
|
|
||||||
desired = torch.empty(self.nx, self.ny)
|
|
||||||
for i in range(self.nx):
|
|
||||||
for j in range(self.ny):
|
|
||||||
desired[i][j] = torch.nn.functional.pairwise_distance(
|
|
||||||
self.x[i].reshape(1, -1),
|
|
||||||
self.y[j].reshape(1, -1),
|
|
||||||
p=2,
|
|
||||||
keepdim=False,
|
|
||||||
)
|
|
||||||
mismatch = np.testing.assert_array_almost_equal(actual,
|
|
||||||
desired,
|
|
||||||
decimal=3)
|
|
||||||
self.assertIsNone(mismatch)
|
|
||||||
|
|
||||||
def test_squared_euclidean(self):
|
|
||||||
actual = distances.squared_euclidean_distance(self.x, self.y)
|
|
||||||
desired = torch.empty(self.nx, self.ny)
|
|
||||||
for i in range(self.nx):
|
|
||||||
for j in range(self.ny):
|
|
||||||
desired[i][j] = (torch.nn.functional.pairwise_distance(
|
|
||||||
self.x[i].reshape(1, -1),
|
|
||||||
self.y[j].reshape(1, -1),
|
|
||||||
p=2,
|
|
||||||
keepdim=False,
|
|
||||||
)**2)
|
|
||||||
mismatch = np.testing.assert_array_almost_equal(actual,
|
|
||||||
desired,
|
|
||||||
decimal=2)
|
|
||||||
self.assertIsNone(mismatch)
|
|
||||||
|
|
||||||
def test_lpnorm_p0(self):
|
|
||||||
actual = distances.lpnorm_distance(self.x, self.y, p=0)
|
|
||||||
desired = torch.empty(self.nx, self.ny)
|
|
||||||
for i in range(self.nx):
|
|
||||||
for j in range(self.ny):
|
|
||||||
desired[i][j] = torch.nn.functional.pairwise_distance(
|
|
||||||
self.x[i].reshape(1, -1),
|
|
||||||
self.y[j].reshape(1, -1),
|
|
||||||
p=0,
|
|
||||||
keepdim=False,
|
|
||||||
)
|
|
||||||
mismatch = np.testing.assert_array_almost_equal(actual,
|
|
||||||
desired,
|
|
||||||
decimal=4)
|
|
||||||
self.assertIsNone(mismatch)
|
|
||||||
|
|
||||||
def test_lpnorm_p2(self):
|
|
||||||
actual = distances.lpnorm_distance(self.x, self.y, p=2)
|
|
||||||
desired = torch.empty(self.nx, self.ny)
|
|
||||||
for i in range(self.nx):
|
|
||||||
for j in range(self.ny):
|
|
||||||
desired[i][j] = torch.nn.functional.pairwise_distance(
|
|
||||||
self.x[i].reshape(1, -1),
|
|
||||||
self.y[j].reshape(1, -1),
|
|
||||||
p=2,
|
|
||||||
keepdim=False,
|
|
||||||
)
|
|
||||||
mismatch = np.testing.assert_array_almost_equal(actual,
|
|
||||||
desired,
|
|
||||||
decimal=4)
|
|
||||||
self.assertIsNone(mismatch)
|
|
||||||
|
|
||||||
def test_lpnorm_p3(self):
|
|
||||||
actual = distances.lpnorm_distance(self.x, self.y, p=3)
|
|
||||||
desired = torch.empty(self.nx, self.ny)
|
|
||||||
for i in range(self.nx):
|
|
||||||
for j in range(self.ny):
|
|
||||||
desired[i][j] = torch.nn.functional.pairwise_distance(
|
|
||||||
self.x[i].reshape(1, -1),
|
|
||||||
self.y[j].reshape(1, -1),
|
|
||||||
p=3,
|
|
||||||
keepdim=False,
|
|
||||||
)
|
|
||||||
mismatch = np.testing.assert_array_almost_equal(actual,
|
|
||||||
desired,
|
|
||||||
decimal=4)
|
|
||||||
self.assertIsNone(mismatch)
|
|
||||||
|
|
||||||
def test_lpnorm_pinf(self):
|
|
||||||
actual = distances.lpnorm_distance(self.x, self.y, p=float("inf"))
|
|
||||||
desired = torch.empty(self.nx, self.ny)
|
|
||||||
for i in range(self.nx):
|
|
||||||
for j in range(self.ny):
|
|
||||||
desired[i][j] = torch.nn.functional.pairwise_distance(
|
|
||||||
self.x[i].reshape(1, -1),
|
|
||||||
self.y[j].reshape(1, -1),
|
|
||||||
p=float("inf"),
|
|
||||||
keepdim=False,
|
|
||||||
)
|
|
||||||
mismatch = np.testing.assert_array_almost_equal(actual,
|
|
||||||
desired,
|
|
||||||
decimal=4)
|
|
||||||
self.assertIsNone(mismatch)
|
|
||||||
|
|
||||||
def test_omega_identity(self):
|
|
||||||
omega = torch.eye(self.mx, self.my)
|
|
||||||
actual = distances.omega_distance(self.x, self.y, omega=omega)
|
|
||||||
desired = torch.empty(self.nx, self.ny)
|
|
||||||
for i in range(self.nx):
|
|
||||||
for j in range(self.ny):
|
|
||||||
desired[i][j] = (torch.nn.functional.pairwise_distance(
|
|
||||||
self.x[i].reshape(1, -1),
|
|
||||||
self.y[j].reshape(1, -1),
|
|
||||||
p=2,
|
|
||||||
keepdim=False,
|
|
||||||
)**2)
|
|
||||||
mismatch = np.testing.assert_array_almost_equal(actual,
|
|
||||||
desired,
|
|
||||||
decimal=2)
|
|
||||||
self.assertIsNone(mismatch)
|
|
||||||
|
|
||||||
def test_lomega_identity(self):
|
|
||||||
omega = torch.eye(self.mx, self.my)
|
|
||||||
omegas = torch.stack([omega for _ in range(self.ny)], dim=0)
|
|
||||||
actual = distances.lomega_distance(self.x, self.y, omegas=omegas)
|
|
||||||
desired = torch.empty(self.nx, self.ny)
|
|
||||||
for i in range(self.nx):
|
|
||||||
for j in range(self.ny):
|
|
||||||
desired[i][j] = (torch.nn.functional.pairwise_distance(
|
|
||||||
self.x[i].reshape(1, -1),
|
|
||||||
self.y[j].reshape(1, -1),
|
|
||||||
p=2,
|
|
||||||
keepdim=False,
|
|
||||||
)**2)
|
|
||||||
mismatch = np.testing.assert_array_almost_equal(actual,
|
|
||||||
desired,
|
|
||||||
decimal=2)
|
|
||||||
self.assertIsNone(mismatch)
|
|
||||||
|
|
||||||
def tearDown(self):
|
|
||||||
del self.x, self.y
|
|
||||||
|
|
||||||
|
|
||||||
class TestInitializers(unittest.TestCase):
|
|
||||||
def setUp(self):
|
|
||||||
self.flist = [
|
|
||||||
"zeros",
|
|
||||||
"ones",
|
|
||||||
"rand",
|
|
||||||
"randn",
|
|
||||||
"stratified_mean",
|
|
||||||
"stratified_random",
|
|
||||||
]
|
|
||||||
self.x = torch.tensor(
|
|
||||||
[[0, -1, -2], [10, 11, 12], [0, 0, 0], [2, 2, 2]],
|
|
||||||
dtype=torch.float32)
|
|
||||||
self.y = torch.tensor([0, 0, 1, 1])
|
|
||||||
self.gen = torch.manual_seed(42)
|
|
||||||
|
|
||||||
def test_registry(self):
|
|
||||||
self.assertIsNotNone(initializers.INITIALIZERS)
|
|
||||||
|
|
||||||
def test_funcname_deserialization(self):
|
|
||||||
for funcname in self.flist:
|
|
||||||
f = initializers.get_initializer(funcname)
|
|
||||||
iscallable = callable(f)
|
|
||||||
self.assertTrue(iscallable)
|
|
||||||
|
|
||||||
def test_callable_deserialization(self):
|
|
||||||
def dummy(x):
|
|
||||||
return x
|
|
||||||
|
|
||||||
for f in [dummy, lambda x: x]:
|
|
||||||
f = initializers.get_initializer(f)
|
|
||||||
iscallable = callable(f)
|
|
||||||
self.assertTrue(iscallable)
|
|
||||||
self.assertEqual(1, f(1))
|
|
||||||
|
|
||||||
def test_unknown_deserialization(self):
|
|
||||||
for funcname in ["blubb", "foobar"]:
|
|
||||||
with self.assertRaises(NameError):
|
|
||||||
_ = initializers.get_initializer(funcname)
|
|
||||||
|
|
||||||
def test_zeros(self):
|
|
||||||
pdist = torch.tensor([1, 1])
|
|
||||||
actual, _ = initializers.zeros(self.x, self.y, pdist)
|
|
||||||
desired = torch.zeros(2, 3)
|
|
||||||
mismatch = np.testing.assert_array_almost_equal(actual,
|
|
||||||
desired,
|
|
||||||
decimal=5)
|
|
||||||
self.assertIsNone(mismatch)
|
|
||||||
|
|
||||||
def test_ones(self):
|
|
||||||
pdist = torch.tensor([1, 1])
|
|
||||||
actual, _ = initializers.ones(self.x, self.y, pdist)
|
|
||||||
desired = torch.ones(2, 3)
|
|
||||||
mismatch = np.testing.assert_array_almost_equal(actual,
|
|
||||||
desired,
|
|
||||||
decimal=5)
|
|
||||||
self.assertIsNone(mismatch)
|
|
||||||
|
|
||||||
def test_rand(self):
|
|
||||||
pdist = torch.tensor([1, 1])
|
|
||||||
actual, _ = initializers.rand(self.x, self.y, pdist)
|
|
||||||
desired = torch.rand(2, 3, generator=torch.manual_seed(42))
|
|
||||||
mismatch = np.testing.assert_array_almost_equal(actual,
|
|
||||||
desired,
|
|
||||||
decimal=5)
|
|
||||||
self.assertIsNone(mismatch)
|
|
||||||
|
|
||||||
def test_randn(self):
|
|
||||||
pdist = torch.tensor([1, 1])
|
|
||||||
actual, _ = initializers.randn(self.x, self.y, pdist)
|
|
||||||
desired = torch.randn(2, 3, generator=torch.manual_seed(42))
|
|
||||||
mismatch = np.testing.assert_array_almost_equal(actual,
|
|
||||||
desired,
|
|
||||||
decimal=5)
|
|
||||||
self.assertIsNone(mismatch)
|
|
||||||
|
|
||||||
def test_stratified_mean_equal1(self):
|
|
||||||
pdist = torch.tensor([1, 1])
|
|
||||||
actual, _ = initializers.stratified_mean(self.x, self.y, pdist, False)
|
|
||||||
desired = torch.tensor([[5.0, 5.0, 5.0], [1.0, 1.0, 1.0]])
|
|
||||||
mismatch = np.testing.assert_array_almost_equal(actual,
|
|
||||||
desired,
|
|
||||||
decimal=5)
|
|
||||||
self.assertIsNone(mismatch)
|
|
||||||
|
|
||||||
def test_stratified_random_equal1(self):
|
|
||||||
pdist = torch.tensor([1, 1])
|
|
||||||
actual, _ = initializers.stratified_random(self.x, self.y, pdist,
|
|
||||||
False)
|
|
||||||
desired = torch.tensor([[0.0, -1.0, -2.0], [0.0, 0.0, 0.0]])
|
|
||||||
mismatch = np.testing.assert_array_almost_equal(actual,
|
|
||||||
desired,
|
|
||||||
decimal=5)
|
|
||||||
self.assertIsNone(mismatch)
|
|
||||||
|
|
||||||
def test_stratified_mean_equal2(self):
|
|
||||||
pdist = torch.tensor([2, 2])
|
|
||||||
actual, _ = initializers.stratified_mean(self.x, self.y, pdist, False)
|
|
||||||
desired = torch.tensor([[5.0, 5.0, 5.0], [5.0, 5.0, 5.0],
|
|
||||||
[1.0, 1.0, 1.0], [1.0, 1.0, 1.0]])
|
|
||||||
mismatch = np.testing.assert_array_almost_equal(actual,
|
|
||||||
desired,
|
|
||||||
decimal=5)
|
|
||||||
self.assertIsNone(mismatch)
|
|
||||||
|
|
||||||
def test_stratified_random_equal2(self):
|
|
||||||
pdist = torch.tensor([2, 2])
|
|
||||||
actual, _ = initializers.stratified_random(self.x, self.y, pdist,
|
|
||||||
False)
|
|
||||||
desired = torch.tensor([[0.0, -1.0, -2.0], [0.0, -1.0, -2.0],
|
|
||||||
[0.0, 0.0, 0.0], [0.0, 0.0, 0.0]])
|
|
||||||
mismatch = np.testing.assert_array_almost_equal(actual,
|
|
||||||
desired,
|
|
||||||
decimal=5)
|
|
||||||
self.assertIsNone(mismatch)
|
|
||||||
|
|
||||||
def test_stratified_mean_unequal(self):
|
|
||||||
pdist = torch.tensor([1, 3])
|
|
||||||
actual, _ = initializers.stratified_mean(self.x, self.y, pdist, False)
|
|
||||||
desired = torch.tensor([[5.0, 5.0, 5.0], [1.0, 1.0, 1.0],
|
|
||||||
[1.0, 1.0, 1.0], [1.0, 1.0, 1.0]])
|
|
||||||
mismatch = np.testing.assert_array_almost_equal(actual,
|
|
||||||
desired,
|
|
||||||
decimal=5)
|
|
||||||
self.assertIsNone(mismatch)
|
|
||||||
|
|
||||||
def test_stratified_random_unequal(self):
|
|
||||||
pdist = torch.tensor([1, 3])
|
|
||||||
actual, _ = initializers.stratified_random(self.x, self.y, pdist,
|
|
||||||
False)
|
|
||||||
desired = torch.tensor([[0.0, -1.0, -2.0], [0.0, 0.0, 0.0],
|
|
||||||
[0.0, 0.0, 0.0], [0.0, 0.0, 0.0]])
|
|
||||||
mismatch = np.testing.assert_array_almost_equal(actual,
|
|
||||||
desired,
|
|
||||||
decimal=5)
|
|
||||||
self.assertIsNone(mismatch)
|
|
||||||
|
|
||||||
def test_stratified_mean_unequal_one_hot(self):
|
|
||||||
pdist = torch.tensor([1, 3])
|
|
||||||
y = torch.eye(2)[self.y]
|
|
||||||
desired1 = torch.tensor([[5.0, 5.0, 5.0], [1.0, 1.0, 1.0],
|
|
||||||
[1.0, 1.0, 1.0], [1.0, 1.0, 1.0]])
|
|
||||||
actual1, actual2 = initializers.stratified_mean(self.x, y, pdist)
|
|
||||||
desired2 = torch.tensor([[1, 0], [0, 1], [0, 1], [0, 1]])
|
|
||||||
mismatch = np.testing.assert_array_almost_equal(actual1,
|
|
||||||
desired1,
|
|
||||||
decimal=5)
|
|
||||||
mismatch = np.testing.assert_array_almost_equal(actual2,
|
|
||||||
desired2,
|
|
||||||
decimal=5)
|
|
||||||
self.assertIsNone(mismatch)
|
|
||||||
|
|
||||||
def test_stratified_random_unequal_one_hot(self):
|
|
||||||
pdist = torch.tensor([1, 3])
|
|
||||||
y = torch.eye(2)[self.y]
|
|
||||||
actual1, actual2 = initializers.stratified_random(self.x, y, pdist)
|
|
||||||
desired1 = torch.tensor([[0.0, -1.0, -2.0], [0.0, 0.0, 0.0],
|
|
||||||
[0.0, 0.0, 0.0], [0.0, 0.0, 0.0]])
|
|
||||||
desired2 = torch.tensor([[1, 0], [0, 1], [0, 1], [0, 1]])
|
|
||||||
mismatch = np.testing.assert_array_almost_equal(actual1,
|
|
||||||
desired1,
|
|
||||||
decimal=5)
|
|
||||||
mismatch = np.testing.assert_array_almost_equal(actual2,
|
|
||||||
desired2,
|
|
||||||
decimal=5)
|
|
||||||
self.assertIsNone(mismatch)
|
|
||||||
|
|
||||||
def tearDown(self):
|
|
||||||
del self.x, self.y, self.gen
|
|
||||||
_ = torch.seed()
|
|
||||||
|
|
||||||
|
|
||||||
class TestLosses(unittest.TestCase):
|
|
||||||
def setUp(self):
|
|
||||||
pass
|
|
||||||
|
|
||||||
def test_glvq_loss_int_labels(self):
|
|
||||||
d = torch.stack([torch.ones(100), torch.zeros(100)], dim=1)
|
|
||||||
labels = torch.tensor([0, 1])
|
|
||||||
targets = torch.ones(100)
|
|
||||||
batch_loss = losses.glvq_loss(distances=d,
|
|
||||||
target_labels=targets,
|
|
||||||
prototype_labels=labels)
|
|
||||||
loss_value = torch.sum(batch_loss, dim=0)
|
|
||||||
self.assertEqual(loss_value, -100)
|
|
||||||
|
|
||||||
def test_glvq_loss_one_hot_labels(self):
|
|
||||||
d = torch.stack([torch.ones(100), torch.zeros(100)], dim=1)
|
|
||||||
labels = torch.tensor([[0, 1], [1, 0]])
|
|
||||||
wl = torch.tensor([1, 0])
|
|
||||||
targets = torch.stack([wl for _ in range(100)], dim=0)
|
|
||||||
batch_loss = losses.glvq_loss(distances=d,
|
|
||||||
target_labels=targets,
|
|
||||||
prototype_labels=labels)
|
|
||||||
loss_value = torch.sum(batch_loss, dim=0)
|
|
||||||
self.assertEqual(loss_value, -100)
|
|
||||||
|
|
||||||
def test_glvq_loss_one_hot_unequal(self):
|
|
||||||
dlist = [torch.ones(100), torch.zeros(100), torch.zeros(100)]
|
|
||||||
d = torch.stack(dlist, dim=1)
|
|
||||||
labels = torch.tensor([[0, 1], [1, 0], [1, 0]])
|
|
||||||
wl = torch.tensor([1, 0])
|
|
||||||
targets = torch.stack([wl for _ in range(100)], dim=0)
|
|
||||||
batch_loss = losses.glvq_loss(distances=d,
|
|
||||||
target_labels=targets,
|
|
||||||
prototype_labels=labels)
|
|
||||||
loss_value = torch.sum(batch_loss, dim=0)
|
|
||||||
self.assertEqual(loss_value, -100)
|
|
||||||
|
|
||||||
def tearDown(self):
|
|
||||||
pass
|
|
@ -1,298 +0,0 @@
|
|||||||
"""ProtoTorch modules test suite."""
|
|
||||||
|
|
||||||
import unittest
|
|
||||||
|
|
||||||
import numpy as np
|
|
||||||
import torch
|
|
||||||
|
|
||||||
from prototorch.modules import losses, prototypes
|
|
||||||
|
|
||||||
|
|
||||||
class TestPrototypes(unittest.TestCase):
|
|
||||||
def setUp(self):
|
|
||||||
self.x = torch.tensor(
|
|
||||||
[[0, -1, -2], [10, 11, 12], [0, 0, 0], [2, 2, 2]],
|
|
||||||
dtype=torch.float32)
|
|
||||||
self.y = torch.tensor([0, 0, 1, 1])
|
|
||||||
self.gen = torch.manual_seed(42)
|
|
||||||
|
|
||||||
def test_prototypes1d_init_without_input_dim(self):
|
|
||||||
with self.assertRaises(NameError):
|
|
||||||
_ = prototypes.Prototypes1D(nclasses=2)
|
|
||||||
|
|
||||||
def test_prototypes1d_init_without_nclasses(self):
|
|
||||||
with self.assertRaises(NameError):
|
|
||||||
_ = prototypes.Prototypes1D(input_dim=1)
|
|
||||||
|
|
||||||
def test_prototypes1d_init_with_nclasses_1(self):
|
|
||||||
with self.assertWarns(UserWarning):
|
|
||||||
_ = prototypes.Prototypes1D(nclasses=1, input_dim=1)
|
|
||||||
|
|
||||||
def test_prototypes1d_init_without_pdist(self):
|
|
||||||
p1 = prototypes.Prototypes1D(
|
|
||||||
input_dim=6,
|
|
||||||
nclasses=2,
|
|
||||||
prototypes_per_class=4,
|
|
||||||
prototype_initializer="ones",
|
|
||||||
)
|
|
||||||
protos = p1.prototypes
|
|
||||||
actual = protos.detach().numpy()
|
|
||||||
desired = torch.ones(8, 6)
|
|
||||||
mismatch = np.testing.assert_array_almost_equal(actual,
|
|
||||||
desired,
|
|
||||||
decimal=5)
|
|
||||||
self.assertIsNone(mismatch)
|
|
||||||
|
|
||||||
def test_prototypes1d_init_without_data(self):
|
|
||||||
pdist = [2, 2]
|
|
||||||
p1 = prototypes.Prototypes1D(input_dim=3,
|
|
||||||
prototype_distribution=pdist,
|
|
||||||
prototype_initializer="zeros")
|
|
||||||
protos = p1.prototypes
|
|
||||||
actual = protos.detach().numpy()
|
|
||||||
desired = torch.zeros(4, 3)
|
|
||||||
mismatch = np.testing.assert_array_almost_equal(actual,
|
|
||||||
desired,
|
|
||||||
decimal=5)
|
|
||||||
self.assertIsNone(mismatch)
|
|
||||||
|
|
||||||
def test_prototypes1d_proto_init_without_data(self):
|
|
||||||
with self.assertWarns(UserWarning):
|
|
||||||
_ = prototypes.Prototypes1D(
|
|
||||||
input_dim=3,
|
|
||||||
nclasses=2,
|
|
||||||
prototypes_per_class=1,
|
|
||||||
prototype_initializer="stratified_mean",
|
|
||||||
data=None,
|
|
||||||
)
|
|
||||||
|
|
||||||
def test_prototypes1d_init_torch_pdist(self):
|
|
||||||
pdist = torch.tensor([2, 2])
|
|
||||||
p1 = prototypes.Prototypes1D(input_dim=3,
|
|
||||||
prototype_distribution=pdist,
|
|
||||||
prototype_initializer="zeros")
|
|
||||||
protos = p1.prototypes
|
|
||||||
actual = protos.detach().numpy()
|
|
||||||
desired = torch.zeros(4, 3)
|
|
||||||
mismatch = np.testing.assert_array_almost_equal(actual,
|
|
||||||
desired,
|
|
||||||
decimal=5)
|
|
||||||
self.assertIsNone(mismatch)
|
|
||||||
|
|
||||||
def test_prototypes1d_init_without_inputdim_with_data(self):
|
|
||||||
_ = prototypes.Prototypes1D(
|
|
||||||
nclasses=2,
|
|
||||||
prototypes_per_class=1,
|
|
||||||
prototype_initializer="stratified_mean",
|
|
||||||
data=[[[1.0], [0.0]], [1, 0]],
|
|
||||||
)
|
|
||||||
|
|
||||||
def test_prototypes1d_init_with_int_data(self):
|
|
||||||
_ = prototypes.Prototypes1D(
|
|
||||||
nclasses=2,
|
|
||||||
prototypes_per_class=1,
|
|
||||||
prototype_initializer="stratified_mean",
|
|
||||||
data=[[[1], [0]], [1, 0]],
|
|
||||||
)
|
|
||||||
|
|
||||||
def test_prototypes1d_init_one_hot_without_data(self):
|
|
||||||
_ = prototypes.Prototypes1D(
|
|
||||||
input_dim=1,
|
|
||||||
nclasses=2,
|
|
||||||
prototypes_per_class=1,
|
|
||||||
prototype_initializer="stratified_mean",
|
|
||||||
data=None,
|
|
||||||
one_hot_labels=True,
|
|
||||||
)
|
|
||||||
|
|
||||||
def test_prototypes1d_init_one_hot_labels_false(self):
|
|
||||||
"""Test if ValueError is raised when `one_hot_labels` is set to `False`
|
|
||||||
but the provided `data` has one-hot encoded labels.
|
|
||||||
"""
|
|
||||||
with self.assertRaises(ValueError):
|
|
||||||
_ = prototypes.Prototypes1D(
|
|
||||||
input_dim=1,
|
|
||||||
nclasses=2,
|
|
||||||
prototypes_per_class=1,
|
|
||||||
prototype_initializer="stratified_mean",
|
|
||||||
data=([[0.0], [1.0]], [[0, 1], [1, 0]]),
|
|
||||||
one_hot_labels=False,
|
|
||||||
)
|
|
||||||
|
|
||||||
def test_prototypes1d_init_1d_y_data_one_hot_labels_true(self):
|
|
||||||
"""Test if ValueError is raised when `one_hot_labels` is set to `True`
|
|
||||||
but the provided `data` does not contain one-hot encoded labels.
|
|
||||||
"""
|
|
||||||
with self.assertRaises(ValueError):
|
|
||||||
_ = prototypes.Prototypes1D(
|
|
||||||
input_dim=1,
|
|
||||||
nclasses=2,
|
|
||||||
prototypes_per_class=1,
|
|
||||||
prototype_initializer="stratified_mean",
|
|
||||||
data=([[0.0], [1.0]], [0, 1]),
|
|
||||||
one_hot_labels=True,
|
|
||||||
)
|
|
||||||
|
|
||||||
def test_prototypes1d_init_one_hot_labels_true(self):
|
|
||||||
"""Test if ValueError is raised when `one_hot_labels` is set to `True`
|
|
||||||
but the provided `data` contains 2D targets but
|
|
||||||
does not contain one-hot encoded labels.
|
|
||||||
"""
|
|
||||||
with self.assertRaises(ValueError):
|
|
||||||
_ = prototypes.Prototypes1D(
|
|
||||||
input_dim=1,
|
|
||||||
nclasses=2,
|
|
||||||
prototypes_per_class=1,
|
|
||||||
prototype_initializer="stratified_mean",
|
|
||||||
data=([[0.0], [1.0]], [[0], [1]]),
|
|
||||||
one_hot_labels=True,
|
|
||||||
)
|
|
||||||
|
|
||||||
def test_prototypes1d_init_with_int_dtype(self):
|
|
||||||
with self.assertRaises(RuntimeError):
|
|
||||||
_ = prototypes.Prototypes1D(
|
|
||||||
nclasses=2,
|
|
||||||
prototypes_per_class=1,
|
|
||||||
prototype_initializer="stratified_mean",
|
|
||||||
data=[[[1], [0]], [1, 0]],
|
|
||||||
dtype=torch.int32,
|
|
||||||
)
|
|
||||||
|
|
||||||
def test_prototypes1d_inputndim_with_data(self):
|
|
||||||
with self.assertRaises(ValueError):
|
|
||||||
_ = prototypes.Prototypes1D(input_dim=1,
|
|
||||||
nclasses=1,
|
|
||||||
prototypes_per_class=1,
|
|
||||||
data=[[1.0], [1]])
|
|
||||||
|
|
||||||
def test_prototypes1d_inputdim_with_data(self):
|
|
||||||
with self.assertRaises(ValueError):
|
|
||||||
_ = prototypes.Prototypes1D(
|
|
||||||
input_dim=2,
|
|
||||||
nclasses=2,
|
|
||||||
prototypes_per_class=1,
|
|
||||||
prototype_initializer="stratified_mean",
|
|
||||||
data=[[[1.0], [0.0]], [1, 0]],
|
|
||||||
)
|
|
||||||
|
|
||||||
def test_prototypes1d_nclasses_with_data(self):
|
|
||||||
"""Test ValueError raise if provided `nclasses` is not the same
|
|
||||||
as the one computed from the provided `data`.
|
|
||||||
"""
|
|
||||||
with self.assertRaises(ValueError):
|
|
||||||
_ = prototypes.Prototypes1D(
|
|
||||||
input_dim=1,
|
|
||||||
nclasses=1,
|
|
||||||
prototypes_per_class=1,
|
|
||||||
prototype_initializer="stratified_mean",
|
|
||||||
data=[[[1.0], [2.0]], [1, 2]],
|
|
||||||
)
|
|
||||||
|
|
||||||
def test_prototypes1d_init_with_ppc(self):
|
|
||||||
p1 = prototypes.Prototypes1D(data=[self.x, self.y],
|
|
||||||
prototypes_per_class=2,
|
|
||||||
prototype_initializer="zeros")
|
|
||||||
protos = p1.prototypes
|
|
||||||
actual = protos.detach().numpy()
|
|
||||||
desired = torch.zeros(4, 3)
|
|
||||||
mismatch = np.testing.assert_array_almost_equal(actual,
|
|
||||||
desired,
|
|
||||||
decimal=5)
|
|
||||||
self.assertIsNone(mismatch)
|
|
||||||
|
|
||||||
def test_prototypes1d_init_with_pdist(self):
|
|
||||||
p1 = prototypes.Prototypes1D(
|
|
||||||
data=[self.x, self.y],
|
|
||||||
prototype_distribution=[6, 9],
|
|
||||||
prototype_initializer="zeros",
|
|
||||||
)
|
|
||||||
protos = p1.prototypes
|
|
||||||
actual = protos.detach().numpy()
|
|
||||||
desired = torch.zeros(15, 3)
|
|
||||||
mismatch = np.testing.assert_array_almost_equal(actual,
|
|
||||||
desired,
|
|
||||||
decimal=5)
|
|
||||||
self.assertIsNone(mismatch)
|
|
||||||
|
|
||||||
def test_prototypes1d_func_initializer(self):
|
|
||||||
def my_initializer(*args, **kwargs):
|
|
||||||
return torch.full((2, 99), 99.0), torch.tensor([0, 1])
|
|
||||||
|
|
||||||
p1 = prototypes.Prototypes1D(
|
|
||||||
input_dim=99,
|
|
||||||
nclasses=2,
|
|
||||||
prototypes_per_class=1,
|
|
||||||
prototype_initializer=my_initializer,
|
|
||||||
)
|
|
||||||
protos = p1.prototypes
|
|
||||||
actual = protos.detach().numpy()
|
|
||||||
desired = 99 * torch.ones(2, 99)
|
|
||||||
mismatch = np.testing.assert_array_almost_equal(actual,
|
|
||||||
desired,
|
|
||||||
decimal=5)
|
|
||||||
self.assertIsNone(mismatch)
|
|
||||||
|
|
||||||
def test_prototypes1d_forward(self):
|
|
||||||
p1 = prototypes.Prototypes1D(data=[self.x, self.y])
|
|
||||||
protos, _ = p1()
|
|
||||||
actual = protos.detach().numpy()
|
|
||||||
desired = torch.ones(2, 3)
|
|
||||||
mismatch = np.testing.assert_array_almost_equal(actual,
|
|
||||||
desired,
|
|
||||||
decimal=5)
|
|
||||||
self.assertIsNone(mismatch)
|
|
||||||
|
|
||||||
def test_prototypes1d_dist_validate(self):
|
|
||||||
p1 = prototypes.Prototypes1D(input_dim=0, prototype_distribution=[0])
|
|
||||||
with self.assertWarns(UserWarning):
|
|
||||||
_ = p1._validate_prototype_distribution()
|
|
||||||
|
|
||||||
def test_prototypes1d_validate_extra_repr_not_empty(self):
|
|
||||||
p1 = prototypes.Prototypes1D(input_dim=0, prototype_distribution=[0])
|
|
||||||
rep = p1.extra_repr()
|
|
||||||
self.assertNotEqual(rep, "")
|
|
||||||
|
|
||||||
def tearDown(self):
|
|
||||||
del self.x, self.y, self.gen
|
|
||||||
_ = torch.seed()
|
|
||||||
|
|
||||||
|
|
||||||
class TestLosses(unittest.TestCase):
|
|
||||||
def setUp(self):
|
|
||||||
pass
|
|
||||||
|
|
||||||
def test_glvqloss_init(self):
|
|
||||||
_ = losses.GLVQLoss(0, "swish_beta", beta=20)
|
|
||||||
|
|
||||||
def test_glvqloss_forward_1ppc(self):
|
|
||||||
criterion = losses.GLVQLoss(margin=0,
|
|
||||||
squashing="sigmoid_beta",
|
|
||||||
beta=100)
|
|
||||||
d = torch.stack([torch.ones(100), torch.zeros(100)], dim=1)
|
|
||||||
labels = torch.tensor([0, 1])
|
|
||||||
targets = torch.ones(100)
|
|
||||||
outputs = [d, labels]
|
|
||||||
loss = criterion(outputs, targets)
|
|
||||||
loss_value = loss.item()
|
|
||||||
self.assertAlmostEqual(loss_value, 0.0)
|
|
||||||
|
|
||||||
def test_glvqloss_forward_2ppc(self):
|
|
||||||
criterion = losses.GLVQLoss(margin=0,
|
|
||||||
squashing="sigmoid_beta",
|
|
||||||
beta=100)
|
|
||||||
d = torch.stack([
|
|
||||||
torch.ones(100),
|
|
||||||
torch.ones(100),
|
|
||||||
torch.zeros(100),
|
|
||||||
torch.ones(100)
|
|
||||||
],
|
|
||||||
dim=1)
|
|
||||||
labels = torch.tensor([0, 0, 1, 1])
|
|
||||||
targets = torch.ones(100)
|
|
||||||
outputs = [d, labels]
|
|
||||||
loss = criterion(outputs, targets)
|
|
||||||
loss_value = loss.item()
|
|
||||||
self.assertAlmostEqual(loss_value, 0.0)
|
|
||||||
|
|
||||||
def tearDown(self):
|
|
||||||
pass
|
|
47
tests/test_utils.py
Normal file
47
tests/test_utils.py
Normal file
@ -0,0 +1,47 @@
|
|||||||
|
"""ProtoTorch utils test suite"""
|
||||||
|
|
||||||
|
import numpy as np
|
||||||
|
import torch
|
||||||
|
|
||||||
|
import prototorch as pt
|
||||||
|
|
||||||
|
|
||||||
|
def test_mesh2d_without_input():
|
||||||
|
mesh, xx, yy = pt.utils.mesh2d(border=2.0, resolution=10)
|
||||||
|
assert mesh.shape[0] == 100
|
||||||
|
assert mesh.shape[1] == 2
|
||||||
|
assert xx.shape[0] == 10
|
||||||
|
assert xx.shape[1] == 10
|
||||||
|
assert yy.shape[0] == 10
|
||||||
|
assert yy.shape[1] == 10
|
||||||
|
assert np.min(xx) == -2.0
|
||||||
|
assert np.max(xx) == 2.0
|
||||||
|
assert np.min(yy) == -2.0
|
||||||
|
assert np.max(yy) == 2.0
|
||||||
|
|
||||||
|
|
||||||
|
def test_mesh2d_with_torch_input():
|
||||||
|
x = 10 * torch.rand(5, 2)
|
||||||
|
mesh, xx, yy = pt.utils.mesh2d(x, border=0.0, resolution=100)
|
||||||
|
assert mesh.shape[0] == 100 * 100
|
||||||
|
assert mesh.shape[1] == 2
|
||||||
|
assert xx.shape[0] == 100
|
||||||
|
assert xx.shape[1] == 100
|
||||||
|
assert yy.shape[0] == 100
|
||||||
|
assert yy.shape[1] == 100
|
||||||
|
assert np.min(xx) == x[:, 0].min()
|
||||||
|
assert np.max(xx) == x[:, 0].max()
|
||||||
|
assert np.min(yy) == x[:, 1].min()
|
||||||
|
assert np.max(yy) == x[:, 1].max()
|
||||||
|
|
||||||
|
|
||||||
|
def test_hex_to_rgb():
|
||||||
|
red_rgb = list(pt.utils.hex_to_rgb(["#ff0000"]))[0]
|
||||||
|
assert red_rgb[0] == 255
|
||||||
|
assert red_rgb[1] == 0
|
||||||
|
assert red_rgb[2] == 0
|
||||||
|
|
||||||
|
|
||||||
|
def test_rgb_to_hex():
|
||||||
|
blue_hex = list(pt.utils.rgb_to_hex([(0, 0, 255)]))[0]
|
||||||
|
assert blue_hex.lower() == "0000ff"
|
Loading…
Reference in New Issue
Block a user