Compare commits
No commits in common. "master" and "v0.1.0-dev0" have entirely different histories.
master
...
v0.1.0-dev
@ -1,13 +1,21 @@
|
||||
[bumpversion]
|
||||
current_version = 0.7.6
|
||||
current_version = 0.1.0-dev0
|
||||
commit = True
|
||||
tag = True
|
||||
parse = (?P<major>\d+)\.(?P<minor>\d+)\.(?P<patch>\d+)
|
||||
serialize = {major}.{minor}.{patch}
|
||||
message = build: bump version {current_version} → {new_version}
|
||||
parse = (?P<major>\d+)\.(?P<minor>\d+)\.(?P<patch>\d+)(\-(?P<release>[a-z]+)(?P<build>\d+))?
|
||||
serialize =
|
||||
{major}.{minor}.{patch}-{release}{build}
|
||||
{major}.{minor}.{patch}
|
||||
|
||||
[bumpversion:part:release]
|
||||
optional_value = prod
|
||||
first_value = dev
|
||||
values =
|
||||
dev
|
||||
rc
|
||||
prod
|
||||
|
||||
[bumpversion:file:setup.py]
|
||||
|
||||
[bumpversion:file:./prototorch/__init__.py]
|
||||
|
||||
[bumpversion:file:./docs/source/conf.py]
|
||||
|
2
.codecov.yml
Normal file
2
.codecov.yml
Normal file
@ -0,0 +1,2 @@
|
||||
comment:
|
||||
require_changes: yes
|
38
.github/ISSUE_TEMPLATE/bug_report.md
vendored
38
.github/ISSUE_TEMPLATE/bug_report.md
vendored
@ -1,38 +0,0 @@
|
||||
---
|
||||
name: Bug report
|
||||
about: Create a report to help us improve
|
||||
title: ''
|
||||
labels: ''
|
||||
assignees: ''
|
||||
|
||||
---
|
||||
|
||||
**Describe the bug**
|
||||
A clear and concise description of what the bug is.
|
||||
|
||||
**Steps to reproduce the behavior**
|
||||
1. ...
|
||||
2. Run script '...' or this snippet:
|
||||
```python
|
||||
import prototorch as pt
|
||||
|
||||
...
|
||||
```
|
||||
3. See errors
|
||||
|
||||
**Expected behavior**
|
||||
A clear and concise description of what you expected to happen.
|
||||
|
||||
**Observed behavior**
|
||||
A clear and concise description of what actually happened.
|
||||
|
||||
**Screenshots**
|
||||
If applicable, add screenshots to help explain your problem.
|
||||
|
||||
**System and version information**
|
||||
- OS: [e.g. Ubuntu 20.10]
|
||||
- ProtoTorch Version: [e.g. 0.4.0]
|
||||
- Python Version: [e.g. 3.9.5]
|
||||
|
||||
**Additional context**
|
||||
Add any other context about the problem here.
|
20
.github/ISSUE_TEMPLATE/feature_request.md
vendored
20
.github/ISSUE_TEMPLATE/feature_request.md
vendored
@ -1,20 +0,0 @@
|
||||
---
|
||||
name: Feature request
|
||||
about: Suggest an idea for this project
|
||||
title: ''
|
||||
labels: ''
|
||||
assignees: ''
|
||||
|
||||
---
|
||||
|
||||
**Is your feature request related to a problem? Please describe.**
|
||||
A clear and concise description of what the problem is. Ex. I'm always frustrated when [...]
|
||||
|
||||
**Describe the solution you'd like**
|
||||
A clear and concise description of what you want to happen.
|
||||
|
||||
**Describe alternatives you've considered**
|
||||
A clear and concise description of any alternative solutions or features you've considered.
|
||||
|
||||
**Additional context**
|
||||
Add any other context or screenshots about the feature request here.
|
76
.github/workflows/pythonapp.yml
vendored
76
.github/workflows/pythonapp.yml
vendored
@ -1,75 +1,37 @@
|
||||
# This workflow will install Python dependencies, run tests and lint with a single version of Python
|
||||
# For more information see: https://help.github.com/actions/language-and-framework-guides/using-python-with-github-actions
|
||||
|
||||
name: tests
|
||||
name: Tests
|
||||
|
||||
on:
|
||||
push:
|
||||
branches: [ master, dev ]
|
||||
pull_request:
|
||||
branches: [master]
|
||||
branches: [ master ]
|
||||
|
||||
jobs:
|
||||
style:
|
||||
runs-on: ubuntu-latest
|
||||
steps:
|
||||
- uses: actions/checkout@v3
|
||||
- name: Set up Python 3.11
|
||||
uses: actions/setup-python@v4
|
||||
with:
|
||||
python-version: "3.11"
|
||||
- name: Install dependencies
|
||||
run: |
|
||||
python -m pip install --upgrade pip
|
||||
pip install .[all]
|
||||
- uses: pre-commit/action@v3.0.0
|
||||
compatibility:
|
||||
needs: style
|
||||
strategy:
|
||||
fail-fast: false
|
||||
matrix:
|
||||
python-version: ["3.8", "3.9", "3.10", "3.11"]
|
||||
os: [ubuntu-latest, windows-latest]
|
||||
exclude:
|
||||
- os: windows-latest
|
||||
python-version: "3.8"
|
||||
- os: windows-latest
|
||||
python-version: "3.9"
|
||||
- os: windows-latest
|
||||
python-version: "3.10"
|
||||
build:
|
||||
|
||||
runs-on: ubuntu-latest
|
||||
|
||||
runs-on: ${{ matrix.os }}
|
||||
steps:
|
||||
- uses: actions/checkout@v3
|
||||
- name: Set up Python ${{ matrix.python-version }}
|
||||
uses: actions/setup-python@v4
|
||||
- uses: actions/checkout@v2
|
||||
- name: Set up Python 3.8
|
||||
uses: actions/setup-python@v1
|
||||
with:
|
||||
python-version: ${{ matrix.python-version }}
|
||||
python-version: 3.8
|
||||
- name: Install dependencies
|
||||
run: |
|
||||
python -m pip install --upgrade pip
|
||||
pip install .[all]
|
||||
pip install .
|
||||
- name: Lint with flake8
|
||||
run: |
|
||||
pip install flake8
|
||||
# stop the build if there are Python syntax errors or undefined names
|
||||
flake8 . --count --select=E9,F63,F7,F82 --show-source --statistics
|
||||
# exit-zero treats all errors as warnings. The GitHub editor is 127 chars wide
|
||||
flake8 . --count --exit-zero --max-complexity=10 --max-line-length=127 --statistics
|
||||
- name: Test with pytest
|
||||
run: |
|
||||
pip install pytest
|
||||
pytest
|
||||
publish_pypi:
|
||||
if: github.event_name == 'push' && startsWith(github.ref, 'refs/tags')
|
||||
needs: compatibility
|
||||
runs-on: ubuntu-latest
|
||||
steps:
|
||||
- uses: actions/checkout@v3
|
||||
- name: Set up Python 3.10
|
||||
uses: actions/setup-python@v4
|
||||
with:
|
||||
python-version: "3.11"
|
||||
- name: Install dependencies
|
||||
run: |
|
||||
python -m pip install --upgrade pip
|
||||
pip install .[all]
|
||||
pip install wheel
|
||||
- name: Build package
|
||||
run: python setup.py sdist bdist_wheel
|
||||
- name: Publish a Python distribution to PyPI
|
||||
uses: pypa/gh-action-pypi-publish@release/v1
|
||||
with:
|
||||
user: __token__
|
||||
password: ${{ secrets.PYPI_API_TOKEN }}
|
||||
|
17
.gitignore
vendored
17
.gitignore
vendored
@ -129,6 +129,14 @@ dmypy.json
|
||||
|
||||
# End of https://www.gitignore.io/api/python
|
||||
|
||||
# ProtoFlow
|
||||
core
|
||||
checkpoint
|
||||
logs/
|
||||
saved_weights/
|
||||
scratch*
|
||||
|
||||
|
||||
# Created by https://www.gitignore.io/api/visualstudiocode
|
||||
# Edit at https://www.gitignore.io/?templates=visualstudiocode
|
||||
|
||||
@ -146,13 +154,4 @@ dmypy.json
|
||||
# End of https://www.gitignore.io/api/visualstudiocode
|
||||
.vscode/
|
||||
|
||||
# Vim
|
||||
*~
|
||||
*.swp
|
||||
*.swo
|
||||
|
||||
# Artifacts created by ProtoTorch
|
||||
reports
|
||||
artifacts
|
||||
examples/_*.py
|
||||
examples/_*.ipynb
|
||||
|
@ -1,53 +0,0 @@
|
||||
# See https://pre-commit.com for more information
|
||||
# See https://pre-commit.com/hooks.html for more hooks
|
||||
|
||||
repos:
|
||||
- repo: https://github.com/pre-commit/pre-commit-hooks
|
||||
rev: v4.4.0
|
||||
hooks:
|
||||
- id: trailing-whitespace
|
||||
- id: end-of-file-fixer
|
||||
- id: check-yaml
|
||||
- id: check-added-large-files
|
||||
- id: check-ast
|
||||
- id: check-case-conflict
|
||||
|
||||
- repo: https://github.com/myint/autoflake
|
||||
rev: v2.1.1
|
||||
hooks:
|
||||
- id: autoflake
|
||||
|
||||
- repo: http://github.com/PyCQA/isort
|
||||
rev: 5.12.0
|
||||
hooks:
|
||||
- id: isort
|
||||
|
||||
- repo: https://github.com/pre-commit/mirrors-mypy
|
||||
rev: v1.3.0
|
||||
hooks:
|
||||
- id: mypy
|
||||
files: prototorch
|
||||
additional_dependencies: [types-pkg_resources]
|
||||
|
||||
- repo: https://github.com/pre-commit/mirrors-yapf
|
||||
rev: v0.32.0
|
||||
hooks:
|
||||
- id: yapf
|
||||
|
||||
- repo: https://github.com/pre-commit/pygrep-hooks
|
||||
rev: v1.10.0
|
||||
hooks:
|
||||
- id: python-use-type-annotations
|
||||
- id: python-no-log-warn
|
||||
- id: python-check-blanket-noqa
|
||||
|
||||
- repo: https://github.com/asottile/pyupgrade
|
||||
rev: v3.7.0
|
||||
hooks:
|
||||
- id: pyupgrade
|
||||
|
||||
- repo: https://github.com/si-cim/gitlint
|
||||
rev: v0.15.2-unofficial
|
||||
hooks:
|
||||
- id: gitlint
|
||||
args: [--contrib=CT1, --ignore=B6, --msg-filename]
|
@ -1,27 +0,0 @@
|
||||
# .readthedocs.yml
|
||||
# Read the Docs configuration file
|
||||
# See https://docs.readthedocs.io/en/stable/config-file/v2.html for details
|
||||
|
||||
# Required
|
||||
version: 2
|
||||
|
||||
# Build documentation in the docs/ directory with Sphinx
|
||||
sphinx:
|
||||
configuration: docs/source/conf.py
|
||||
fail_on_warning: true
|
||||
|
||||
# Build documentation with MkDocs
|
||||
# mkdocs:
|
||||
# configuration: mkdocs.yml
|
||||
|
||||
# Optionally build your docs in additional formats such as PDF and ePub
|
||||
formats: all
|
||||
|
||||
# Optionally set the version of Python and requirements required to build your docs
|
||||
python:
|
||||
version: 3.9
|
||||
install:
|
||||
- method: pip
|
||||
path: .
|
||||
extra_requirements:
|
||||
- all
|
@ -1,7 +0,0 @@
|
||||
{
|
||||
"plugins": [
|
||||
"remark-preset-lint-recommended",
|
||||
["remark-lint-list-item-indent", false],
|
||||
["no-emphasis-as-header", false]
|
||||
]
|
||||
}
|
3
LICENSE
3
LICENSE
@ -1,7 +1,6 @@
|
||||
MIT License
|
||||
|
||||
Copyright (c) 2020 Saxon Institute for Computational Intelligence and Machine
|
||||
Learning (SICIM)
|
||||
Copyright (c) 2020 si-cim
|
||||
|
||||
Permission is hereby granted, free of charge, to any person obtaining a copy
|
||||
of this software and associated documentation files (the "Software"), to deal
|
||||
|
@ -1,13 +1,9 @@
|
||||
include .bumpversion.cfg
|
||||
include LICENSE
|
||||
include tox.ini
|
||||
include *.md
|
||||
include *.txt
|
||||
include *.yml
|
||||
recursive-include docs *.bat
|
||||
recursive-include docs *.png
|
||||
recursive-include docs *.py
|
||||
recursive-include docs *.rst
|
||||
recursive-include docs Makefile
|
||||
recursive-include examples *.py
|
||||
recursive-include tests *.py
|
||||
|
84
README.md
84
README.md
@ -1,75 +1,49 @@
|
||||
# ProtoTorch: Prototype Learning in PyTorch
|
||||
# ProtoTorch
|
||||
|
||||
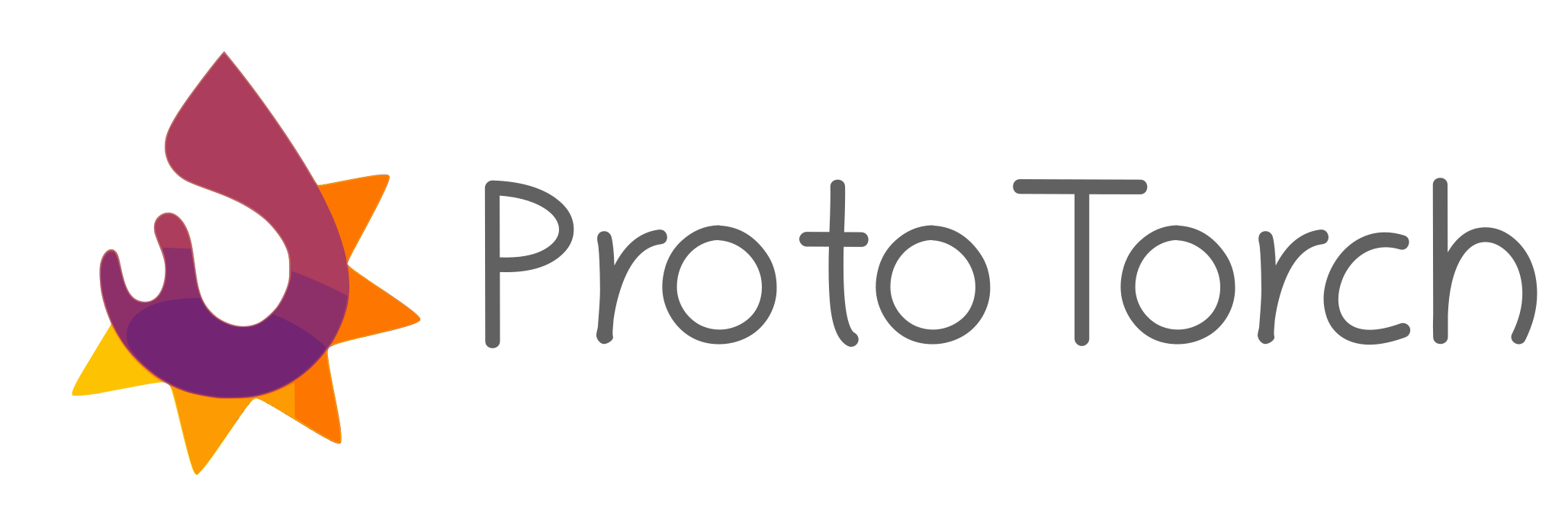
|
||||
ProtoTorch is a PyTorch-based Python toolbox for bleeding-edge research in
|
||||
prototype-based machine learning algorithms.
|
||||
|
||||

|
||||
[](https://github.com/si-cim/prototorch/releases)
|
||||
[](https://pypi.org/project/prototorch/)
|
||||
[](https://github.com/si-cim/prototorch/blob/master/LICENSE)
|
||||
|
||||
*Tensorflow users, see:* [ProtoFlow](https://github.com/si-cim/protoflow)
|
||||

|
||||
[](https://codecov.io/gh/si-cim/prototorch)
|
||||
|
||||
## Description
|
||||
|
||||
This is a Python toolbox brewed at the Mittweida University of Applied Sciences
|
||||
in Germany for bleeding-edge research in Prototype-based Machine Learning
|
||||
methods and other interpretable models. The focus of ProtoTorch is ease-of-use,
|
||||
extensibility and speed.
|
||||
in Germany for bleeding-edge research in Learning Vector Quantization (LVQ)
|
||||
and potentially other prototype-based methods. Although, there are
|
||||
other (perhaps more extensive) LVQ toolboxes available out there, the focus of
|
||||
ProtoTorch is ease-of-use, extensibility and speed.
|
||||
|
||||
Many popular prototype-based Machine Learning (ML) algorithms like K-Nearest
|
||||
Neighbors (KNN), Generalized Learning Vector Quantization (GLVQ) and Generalized
|
||||
Matrix Learning Vector Quantization (GMLVQ) are implemented using the "nn" API
|
||||
provided by PyTorch.
|
||||
|
||||
## Installation
|
||||
|
||||
ProtoTorch can be installed using `pip`.
|
||||
```bash
|
||||
pip install -U prototorch
|
||||
```
|
||||
To also install the extras, use
|
||||
```bash
|
||||
pip install -U prototorch[all]
|
||||
pip install prototorch
|
||||
```
|
||||
|
||||
*Note: If you're using [ZSH](https://www.zsh.org/) (which is also the default
|
||||
shell on MacOS now), the square brackets `[ ]` have to be escaped like so:
|
||||
`\[\]`, making the install command `pip install -U prototorch\[all\]`.*
|
||||
|
||||
To install the bleeding-edge features and improvements:
|
||||
```bash
|
||||
```
|
||||
git clone https://github.com/si-cim/prototorch.git
|
||||
cd prototorch
|
||||
git checkout dev
|
||||
pip install -e .[all]
|
||||
cd prototorch
|
||||
pip install -e .
|
||||
```
|
||||
|
||||
## Documentation
|
||||
## Usage
|
||||
|
||||
The documentation is available at <https://www.prototorch.ml/en/latest/>. Should
|
||||
that link not work try <https://prototorch.readthedocs.io/en/latest/>.
|
||||
ProtoTorch is modular. It is very easy to use the modular pieces provided by
|
||||
ProtoTorch, like the layers, losses, callbacks and metrics to build your own
|
||||
prototype-based(instance-based) models. These pieces blend-in seamlessly with
|
||||
numpy and PyTorch to allow you mix and match the modules from ProtoTorch with
|
||||
other PyTorch modules.
|
||||
|
||||
## Contribution
|
||||
|
||||
This repository contains definition for [git hooks](https://githooks.com).
|
||||
[Pre-commit](https://pre-commit.com) is automatically installed as development
|
||||
dependency with prototorch or you can install it manually with `pip install
|
||||
pre-commit`.
|
||||
|
||||
Please install the hooks by running:
|
||||
```bash
|
||||
pre-commit install
|
||||
pre-commit install --hook-type commit-msg
|
||||
```
|
||||
before creating the first commit.
|
||||
|
||||
The commit will fail if the commit message does not follow the specification
|
||||
provided [here](https://www.conventionalcommits.org/en/v1.0.0/#specification).
|
||||
|
||||
## Bibtex
|
||||
|
||||
If you would like to cite the package, please use this:
|
||||
```bibtex
|
||||
@misc{Ravichandran2020b,
|
||||
author = {Ravichandran, J},
|
||||
title = {ProtoTorch},
|
||||
year = {2020},
|
||||
publisher = {GitHub},
|
||||
journal = {GitHub repository},
|
||||
howpublished = {\url{https://github.com/si-cim/prototorch}}
|
||||
}
|
||||
ProtoTorch comes prepackaged with many popular LVQ algorithms in a convenient
|
||||
API, with more algorithms and techniques coming soon. If you would simply like
|
||||
to be able to use those algorithms to train large ML models on a GPU, ProtoTorch
|
||||
lets you do this without requiring a black-belt in high-performance Tensor
|
||||
computation.
|
||||
|
19
RELEASE.md
19
RELEASE.md
@ -1,19 +0,0 @@
|
||||
# ProtoTorch Releases
|
||||
|
||||
## Release 0.5.0
|
||||
|
||||
- Breaking: Removed deprecated `prototorch.modules.Prototypes1D`.
|
||||
- Use `prototorch.components.LabeledComponents` instead.
|
||||
|
||||
## Release 0.2.0
|
||||
|
||||
- Fixes in example scripts.
|
||||
|
||||
## Release 0.1.1-dev0
|
||||
|
||||
- Minor bugfixes.
|
||||
- 100% line coverage.
|
||||
|
||||
## Release 0.1.0-dev0
|
||||
|
||||
Initial public release of ProtoTorch.
|
@ -1,20 +0,0 @@
|
||||
# Minimal makefile for Sphinx documentation
|
||||
#
|
||||
|
||||
# You can set these variables from the command line, and also
|
||||
# from the environment for the first two.
|
||||
SPHINXOPTS ?=
|
||||
SPHINXBUILD ?= python3 -m sphinx
|
||||
SOURCEDIR = source
|
||||
BUILDDIR = build
|
||||
|
||||
# Put it first so that "make" without argument is like "make help".
|
||||
help:
|
||||
@$(SPHINXBUILD) -M help "$(SOURCEDIR)" "$(BUILDDIR)" $(SPHINXOPTS) $(O)
|
||||
|
||||
.PHONY: help Makefile
|
||||
|
||||
# Catch-all target: route all unknown targets to Sphinx using the new
|
||||
# "make mode" option. $(O) is meant as a shortcut for $(SPHINXOPTS).
|
||||
%: Makefile
|
||||
@$(SPHINXBUILD) -M $@ "$(SOURCEDIR)" "$(BUILDDIR)" $(SPHINXOPTS) $(O)
|
@ -1,35 +0,0 @@
|
||||
@ECHO OFF
|
||||
|
||||
pushd %~dp0
|
||||
|
||||
REM Command file for Sphinx documentation
|
||||
|
||||
if "%SPHINXBUILD%" == "" (
|
||||
set SPHINXBUILD=sphinx-build
|
||||
)
|
||||
set SOURCEDIR=source
|
||||
set BUILDDIR=build
|
||||
|
||||
if "%1" == "" goto help
|
||||
|
||||
%SPHINXBUILD% >NUL 2>NUL
|
||||
if errorlevel 9009 (
|
||||
echo.
|
||||
echo.The 'sphinx-build' command was not found. Make sure you have Sphinx
|
||||
echo.installed, then set the SPHINXBUILD environment variable to point
|
||||
echo.to the full path of the 'sphinx-build' executable. Alternatively you
|
||||
echo.may add the Sphinx directory to PATH.
|
||||
echo.
|
||||
echo.If you don't have Sphinx installed, grab it from
|
||||
echo.http://sphinx-doc.org/
|
||||
exit /b 1
|
||||
)
|
||||
|
||||
%SPHINXBUILD% -M %1 %SOURCEDIR% %BUILDDIR% %SPHINXOPTS% %O%
|
||||
goto end
|
||||
|
||||
:help
|
||||
%SPHINXBUILD% -M help %SOURCEDIR% %BUILDDIR% %SPHINXOPTS% %O%
|
||||
|
||||
:end
|
||||
popd
|
@ -1,4 +0,0 @@
|
||||
torch==1.6.0
|
||||
matplotlib==3.1.2
|
||||
sphinx_rtd_theme==0.5.0
|
||||
sphinxcontrib-katex==0.6.1
|
Binary file not shown.
Before Width: | Height: | Size: 88 KiB |
@ -1,57 +0,0 @@
|
||||
.. ProtoTorch API Reference
|
||||
|
||||
ProtoTorch API Reference
|
||||
======================================
|
||||
|
||||
Datasets
|
||||
--------------------------------------
|
||||
|
||||
Common Datasets
|
||||
~~~~~~~~~~~~~~~~~~~~~~~~~~~~~~~~~~~~~~
|
||||
.. automodule:: prototorch.datasets
|
||||
:members:
|
||||
|
||||
|
||||
Abstract Datasets
|
||||
~~~~~~~~~~~~~~~~~~~~~~~~~~~~~~~~~~~~~~
|
||||
|
||||
Abstract Datasets are used to build your own datasets.
|
||||
|
||||
.. autoclass:: prototorch.datasets.abstract.NumpyDataset
|
||||
:members:
|
||||
|
||||
Functions
|
||||
--------------------------------------
|
||||
|
||||
**Dimensions:**
|
||||
|
||||
- :math:`B` ... Batch size
|
||||
- :math:`P` ... Number of prototypes
|
||||
- :math:`n_x` ... Data dimension for vectorial data
|
||||
- :math:`n_w` ... Data dimension for vectorial prototypes
|
||||
|
||||
Activations
|
||||
~~~~~~~~~~~~~~~~~~~~~~~~~~~~~~~~~~~~~~
|
||||
.. automodule:: prototorch.functions.activations
|
||||
:members:
|
||||
:exclude-members: register_activation, get_activation
|
||||
:undoc-members:
|
||||
|
||||
Distances
|
||||
~~~~~~~~~~~~~~~~~~~~~~~~~~~~~~~~~~~~~~
|
||||
.. automodule:: prototorch.functions.distances
|
||||
:members:
|
||||
:exclude-members: sed
|
||||
:undoc-members:
|
||||
|
||||
Modules
|
||||
--------------------------------------
|
||||
.. automodule:: prototorch.modules
|
||||
:members:
|
||||
:undoc-members:
|
||||
|
||||
Utilities
|
||||
--------------------------------------
|
||||
.. automodule:: prototorch.utils
|
||||
:members:
|
||||
:undoc-members:
|
@ -1,192 +0,0 @@
|
||||
# Configuration file for the Sphinx documentation builder.
|
||||
#
|
||||
# This file only contains a selection of the most common options. For a full
|
||||
# list see the documentation:
|
||||
# https://www.sphinx-doc.org/en/master/usage/configuration.html
|
||||
|
||||
# -- Path setup --------------------------------------------------------------
|
||||
|
||||
# If extensions (or modules to document with autodoc) are in another directory,
|
||||
# add these directories to sys.path here. If the directory is relative to the
|
||||
# documentation root, use os.path.abspath to make it absolute, like shown here.
|
||||
#
|
||||
import os
|
||||
import sys
|
||||
|
||||
sys.path.insert(0, os.path.abspath("../../"))
|
||||
|
||||
# -- Project information -----------------------------------------------------
|
||||
|
||||
project = "ProtoTorch"
|
||||
copyright = "2021, Jensun Ravichandran"
|
||||
author = "Jensun Ravichandran"
|
||||
|
||||
# The full version, including alpha/beta/rc tags
|
||||
#
|
||||
release = "0.7.6"
|
||||
|
||||
# -- General configuration ---------------------------------------------------
|
||||
|
||||
# If your documentation needs a minimal Sphinx version, state it here.
|
||||
#
|
||||
needs_sphinx = "1.6"
|
||||
|
||||
# Add any Sphinx extension module names here, as strings. They can be
|
||||
# extensions coming with Sphinx (named "sphinx.ext.*") or your custom
|
||||
# ones.
|
||||
extensions = [
|
||||
"recommonmark",
|
||||
"sphinx.ext.autodoc",
|
||||
"sphinx.ext.autosummary",
|
||||
"sphinx.ext.doctest",
|
||||
"sphinx.ext.intersphinx",
|
||||
"sphinx.ext.todo",
|
||||
"sphinx.ext.coverage",
|
||||
"sphinx.ext.napoleon",
|
||||
"sphinx.ext.viewcode",
|
||||
"sphinx_rtd_theme",
|
||||
"sphinxcontrib.katex",
|
||||
'sphinx_autodoc_typehints',
|
||||
]
|
||||
|
||||
# katex_prerender = True
|
||||
katex_prerender = False
|
||||
|
||||
napoleon_use_ivar = True
|
||||
|
||||
# Add any paths that contain templates here, relative to this directory.
|
||||
templates_path = ["_templates"]
|
||||
|
||||
# The suffix(es) of source filenames.
|
||||
# You can specify multiple suffix as a list of string:
|
||||
#
|
||||
source_suffix = [".rst", ".md"]
|
||||
|
||||
# The master toctree document.
|
||||
master_doc = "index"
|
||||
|
||||
# List of patterns, relative to source directory, that match files and
|
||||
# directories to ignore when looking for source files.
|
||||
# This pattern also affects html_static_path and html_extra_path.
|
||||
exclude_patterns = []
|
||||
|
||||
# The name of the Pygments (syntax highlighting) style to use. Choose from:
|
||||
# ["default", "emacs", "friendly", "colorful", "autumn", "murphy", "manni",
|
||||
# "monokai", "perldoc", "pastie", "borland", "trac", "native", "fruity", "bw",
|
||||
# "vim", "vs", "tango", "rrt", "xcode", "igor", "paraiso-light", "paraiso-dark",
|
||||
# "lovelace", "algol", "algol_nu", "arduino", "rainbo w_dash", "abap",
|
||||
# "solarized-dark", "solarized-light", "sas", "stata", "stata-light",
|
||||
# "stata-dark", "inkpot"]
|
||||
pygments_style = "monokai"
|
||||
|
||||
# If true, `todo` and `todoList` produce output, else they produce nothing.
|
||||
todo_include_todos = True
|
||||
|
||||
# Disable docstring inheritance
|
||||
autodoc_inherit_docstrings = False
|
||||
|
||||
# -- Options for HTML output -------------------------------------------------
|
||||
|
||||
# The theme to use for HTML and HTML Help pages. See the documentation for
|
||||
# a list of builtin themes.
|
||||
# https://sphinx-themes.org/
|
||||
html_theme = "sphinx_rtd_theme"
|
||||
|
||||
html_logo = "_static/img/horizontal-lockup.png"
|
||||
|
||||
html_theme_options = {
|
||||
"logo_only": True,
|
||||
"display_version": True,
|
||||
"prev_next_buttons_location": "bottom",
|
||||
"style_external_links": False,
|
||||
"style_nav_header_background": "#ffffff",
|
||||
# Toc options
|
||||
"collapse_navigation": True,
|
||||
"sticky_navigation": True,
|
||||
"navigation_depth": 4,
|
||||
"includehidden": True,
|
||||
"titles_only": False,
|
||||
}
|
||||
|
||||
# Add any paths that contain custom static files (such as style sheets) here,
|
||||
# relative to this directory. They are copied after the builtin static files,
|
||||
# so a file named "default.css" will overwrite the builtin "default.css".
|
||||
html_static_path = ["_static"]
|
||||
|
||||
html_css_files = [
|
||||
"https://cdn.jsdelivr.net/npm/katex@0.11.1/dist/katex.min.css",
|
||||
]
|
||||
|
||||
# -- Options for HTMLHelp output ------------------------------------------
|
||||
|
||||
# Output file base name for HTML help builder.
|
||||
htmlhelp_basename = "prototorchdoc"
|
||||
|
||||
# -- Options for LaTeX output ---------------------------------------------
|
||||
|
||||
latex_elements = {
|
||||
# The paper size ("letterpaper" or "a4paper").
|
||||
#
|
||||
# "papersize": "letterpaper",
|
||||
# The font size ("10pt", "11pt" or "12pt").
|
||||
#
|
||||
# "pointsize": "10pt",
|
||||
# Additional stuff for the LaTeX preamble.
|
||||
#
|
||||
# "preamble": "",
|
||||
# Latex figure (float) alignment
|
||||
#
|
||||
# "figure_align": "htbp",
|
||||
}
|
||||
|
||||
# Grouping the document tree into LaTeX files. List of tuples
|
||||
# (source start file, target name, title,
|
||||
# author, documentclass [howto, manual, or own class]).
|
||||
latex_documents = [
|
||||
(
|
||||
master_doc,
|
||||
"prototorch.tex",
|
||||
"ProtoTorch Documentation",
|
||||
"Jensun Ravichandran",
|
||||
"manual",
|
||||
),
|
||||
]
|
||||
|
||||
# -- Options for manual page output ---------------------------------------
|
||||
|
||||
# One entry per manual page. List of tuples
|
||||
# (source start file, name, description, authors, manual section).
|
||||
man_pages = [(master_doc, "ProtoTorch", "ProtoTorch Documentation", [author],
|
||||
1)]
|
||||
|
||||
# -- Options for Texinfo output -------------------------------------------
|
||||
|
||||
# Grouping the document tree into Texinfo files. List of tuples
|
||||
# (source start file, target name, title, author,
|
||||
# dir menu entry, description, category)
|
||||
texinfo_documents = [
|
||||
(
|
||||
master_doc,
|
||||
"prototorch",
|
||||
"ProtoTorch Documentation",
|
||||
author,
|
||||
"prototorch",
|
||||
"Prototype-based machine learning in PyTorch.",
|
||||
"Miscellaneous",
|
||||
),
|
||||
]
|
||||
|
||||
# Example configuration for intersphinx: refer to the Python standard library.
|
||||
intersphinx_mapping = {
|
||||
"python": ("https://docs.python.org/", None),
|
||||
"numpy": ("https://docs.scipy.org/doc/numpy/", None),
|
||||
"torch": ('https://pytorch.org/docs/stable/', None),
|
||||
"pytorch_lightning":
|
||||
("https://pytorch-lightning.readthedocs.io/en/stable/", None),
|
||||
}
|
||||
|
||||
# -- Options for Epub output ----------------------------------------------
|
||||
# https://www.sphinx-doc.org/en/master/usage/configuration.html#options-for-epub-output
|
||||
|
||||
epub_cover = ()
|
||||
version = release
|
@ -1,22 +0,0 @@
|
||||
.. ProtoTorch documentation master file
|
||||
You can adapt this file completely to your liking, but it should at least
|
||||
contain the root `toctree` directive.
|
||||
|
||||
About ProtoTorch
|
||||
================
|
||||
|
||||
.. toctree::
|
||||
:hidden:
|
||||
:maxdepth: 3
|
||||
:caption: Contents:
|
||||
|
||||
self
|
||||
api
|
||||
|
||||
ProtoTorch is a PyTorch-based Python toolbox for bleeding-edge
|
||||
research in prototype-based machine learning algorithms.
|
||||
|
||||
Indices
|
||||
=======
|
||||
* :ref:`genindex`
|
||||
* :ref:`modindex`
|
@ -1,100 +0,0 @@
|
||||
"""ProtoTorch CBC example using 2D Iris data."""
|
||||
|
||||
import logging
|
||||
|
||||
import torch
|
||||
from matplotlib import pyplot as plt
|
||||
|
||||
import prototorch as pt
|
||||
|
||||
|
||||
class CBC(torch.nn.Module):
|
||||
|
||||
def __init__(self, data, **kwargs):
|
||||
super().__init__(**kwargs)
|
||||
self.components_layer = pt.components.ReasoningComponents(
|
||||
distribution=[2, 1, 2],
|
||||
components_initializer=pt.initializers.SSCI(data, noise=0.1),
|
||||
reasonings_initializer=pt.initializers.PPRI(components_first=True),
|
||||
)
|
||||
|
||||
def forward(self, x):
|
||||
components, reasonings = self.components_layer()
|
||||
sims = pt.similarities.euclidean_similarity(x, components)
|
||||
probs = pt.competitions.cbcc(sims, reasonings)
|
||||
return probs
|
||||
|
||||
|
||||
class VisCBC2D():
|
||||
|
||||
def __init__(self, model, data):
|
||||
self.model = model
|
||||
self.x_train, self.y_train = pt.utils.parse_data_arg(data)
|
||||
self.title = "Components Visualization"
|
||||
self.fig = plt.figure(self.title)
|
||||
self.border = 0.1
|
||||
self.resolution = 100
|
||||
self.cmap = "viridis"
|
||||
|
||||
def on_train_epoch_end(self):
|
||||
x_train, y_train = self.x_train, self.y_train
|
||||
_components = self.model.components_layer._components.detach()
|
||||
ax = self.fig.gca()
|
||||
ax.cla()
|
||||
ax.set_title(self.title)
|
||||
ax.axis("off")
|
||||
ax.scatter(
|
||||
x_train[:, 0],
|
||||
x_train[:, 1],
|
||||
c=y_train,
|
||||
cmap=self.cmap,
|
||||
edgecolor="k",
|
||||
marker="o",
|
||||
s=30,
|
||||
)
|
||||
ax.scatter(
|
||||
_components[:, 0],
|
||||
_components[:, 1],
|
||||
c="w",
|
||||
cmap=self.cmap,
|
||||
edgecolor="k",
|
||||
marker="D",
|
||||
s=50,
|
||||
)
|
||||
x = torch.vstack((x_train, _components))
|
||||
mesh_input, xx, yy = pt.utils.mesh2d(x, self.border, self.resolution)
|
||||
with torch.no_grad():
|
||||
y_pred = self.model(
|
||||
torch.Tensor(mesh_input).type_as(_components)).argmax(1)
|
||||
y_pred = y_pred.cpu().reshape(xx.shape)
|
||||
ax.contourf(xx, yy, y_pred, cmap=self.cmap, alpha=0.35)
|
||||
plt.pause(0.2)
|
||||
|
||||
|
||||
if __name__ == "__main__":
|
||||
train_ds = pt.datasets.Iris(dims=[0, 2])
|
||||
|
||||
train_loader = torch.utils.data.DataLoader(train_ds, batch_size=32)
|
||||
|
||||
model = CBC(train_ds)
|
||||
|
||||
optimizer = torch.optim.Adam(model.parameters(), lr=0.01)
|
||||
criterion = pt.losses.MarginLoss(margin=0.1)
|
||||
vis = VisCBC2D(model, train_ds)
|
||||
|
||||
for epoch in range(200):
|
||||
correct = 0.0
|
||||
for x, y in train_loader:
|
||||
y_oh = torch.eye(3)[y]
|
||||
y_pred = model(x)
|
||||
loss = criterion(y_pred, y_oh).mean(0)
|
||||
|
||||
optimizer.zero_grad()
|
||||
loss.backward()
|
||||
optimizer.step()
|
||||
|
||||
correct += (y_pred.argmax(1) == y).float().sum(0)
|
||||
|
||||
acc = 100 * correct / len(train_ds)
|
||||
logging.info(f"Epoch: {epoch} Accuracy: {acc:05.02f}%")
|
||||
vis.on_train_epoch_end()
|
103
examples/glvq_iris.py
Normal file
103
examples/glvq_iris.py
Normal file
@ -0,0 +1,103 @@
|
||||
"""ProtoTorch GLVQ example using 2D Iris data"""
|
||||
|
||||
import numpy as np
|
||||
import torch
|
||||
from matplotlib import pyplot as plt
|
||||
from sklearn.datasets import load_iris
|
||||
from sklearn.preprocessing import StandardScaler
|
||||
|
||||
from prototorch.functions.distances import euclidean_distance
|
||||
from prototorch.modules.losses import GLVQLoss
|
||||
from prototorch.modules.prototypes import AddPrototypes1D
|
||||
|
||||
# Prepare and preprocess the data
|
||||
scaler = StandardScaler()
|
||||
x_train, y_train = load_iris(True)
|
||||
x_train = x_train[:, [0, 2]]
|
||||
scaler.fit(x_train)
|
||||
x_train = scaler.transform(x_train)
|
||||
|
||||
|
||||
# Define the GLVQ model
|
||||
class Model(torch.nn.Module):
|
||||
def __init__(self, **kwargs):
|
||||
super().__init__()
|
||||
self.p1 = AddPrototypes1D(input_dim=2,
|
||||
prototypes_per_class=1,
|
||||
nclasses=3,
|
||||
prototype_initializer='zeros')
|
||||
|
||||
def forward(self, x):
|
||||
protos = self.p1.prototypes
|
||||
plabels = self.p1.prototype_labels
|
||||
dis = euclidean_distance(x, protos)
|
||||
return dis, plabels
|
||||
|
||||
|
||||
# Build the GLVQ model
|
||||
model = Model()
|
||||
|
||||
# Optimize using SGD optimizer from `torch.optim`
|
||||
optimizer = torch.optim.SGD(model.parameters(), lr=0.01)
|
||||
criterion = GLVQLoss(squashing='sigmoid_beta', beta=10)
|
||||
|
||||
# Training loop
|
||||
fig = plt.figure('Prototype Visualization')
|
||||
for epoch in range(70):
|
||||
# Compute loss.
|
||||
distances, plabels = model(torch.tensor(x_train))
|
||||
loss = criterion([distances, plabels], torch.tensor(y_train))
|
||||
print(f'Epoch: {epoch + 1:03d} Loss: {loss.item():02.02f}')
|
||||
|
||||
# Take a gradient descent step
|
||||
optimizer.zero_grad()
|
||||
loss.backward()
|
||||
optimizer.step()
|
||||
|
||||
# Get the prototypes form the model
|
||||
protos = model.p1.prototypes.data.numpy()
|
||||
|
||||
# Visualize the data and the prototypes
|
||||
ax = fig.gca()
|
||||
ax.cla()
|
||||
cmap = 'viridis'
|
||||
ax.scatter(x_train[:, 0], x_train[:, 1], c=y_train, edgecolor='k')
|
||||
ax.scatter(protos[:, 0],
|
||||
protos[:, 1],
|
||||
c=plabels,
|
||||
cmap=cmap,
|
||||
edgecolor='k',
|
||||
marker='D',
|
||||
s=50)
|
||||
|
||||
# Paint decision regions
|
||||
border = 1
|
||||
resolution = 50
|
||||
x = np.vstack((x_train, protos))
|
||||
x_min, x_max = x[:, 0].min(), x[:, 0].max()
|
||||
y_min, y_max = x[:, 1].min(), x[:, 1].max()
|
||||
x_min, x_max = x_min - border, x_max + border
|
||||
y_min, y_max = y_min - border, y_max + border
|
||||
try:
|
||||
xx, yy = np.meshgrid(np.arange(x_min, x_max, 1.0 / resolution),
|
||||
np.arange(y_min, y_max, 1.0 / resolution))
|
||||
except ValueError as ve:
|
||||
print(ve)
|
||||
raise ValueError(f'x_min: {x_min}, x_max: {x_max}. '
|
||||
f'x_min - x_max is {x_max - x_min}.')
|
||||
except MemoryError as me:
|
||||
print(me)
|
||||
raise ValueError('Too many points. ' 'Try reducing the resolution.')
|
||||
mesh_input = np.c_[xx.ravel(), yy.ravel()]
|
||||
|
||||
torch_input = torch.from_numpy(mesh_input)
|
||||
d = model(torch_input)[0]
|
||||
y_pred = np.argmin(d.detach().numpy(), axis=1)
|
||||
y_pred = y_pred.reshape(xx.shape)
|
||||
|
||||
# Plot voronoi regions
|
||||
ax.contourf(xx, yy, y_pred, cmap=cmap, alpha=0.35)
|
||||
|
||||
ax.set_xlim(left=x_min + 0, right=x_max - 0)
|
||||
ax.set_ylim(bottom=y_min + 0, top=y_max - 0)
|
||||
plt.pause(0.1)
|
@ -1,76 +0,0 @@
|
||||
"""ProtoTorch GMLVQ example using Iris data."""
|
||||
|
||||
import torch
|
||||
|
||||
import prototorch as pt
|
||||
|
||||
|
||||
class GMLVQ(torch.nn.Module):
|
||||
"""
|
||||
Implementation of Generalized Matrix Learning Vector Quantization.
|
||||
"""
|
||||
|
||||
def __init__(self, data, **kwargs):
|
||||
super().__init__(**kwargs)
|
||||
|
||||
self.components_layer = pt.components.LabeledComponents(
|
||||
distribution=[1, 1, 1],
|
||||
components_initializer=pt.initializers.SMCI(data, noise=0.1),
|
||||
)
|
||||
|
||||
self.backbone = pt.transforms.Omega(
|
||||
len(data[0][0]),
|
||||
len(data[0][0]),
|
||||
pt.initializers.RandomLinearTransformInitializer(),
|
||||
)
|
||||
|
||||
def forward(self, data):
|
||||
"""
|
||||
Forward function that returns a tuple of dissimilarities and label information.
|
||||
Feed into GLVQLoss to get a complete GMLVQ model.
|
||||
"""
|
||||
components, label = self.components_layer()
|
||||
|
||||
latent_x = self.backbone(data)
|
||||
latent_components = self.backbone(components)
|
||||
|
||||
distance = pt.distances.squared_euclidean_distance(
|
||||
latent_x, latent_components)
|
||||
|
||||
return distance, label
|
||||
|
||||
def predict(self, data):
|
||||
"""
|
||||
The GMLVQ has a modified prediction step, where a competition layer is applied.
|
||||
"""
|
||||
components, label = self.components_layer()
|
||||
distance = pt.distances.squared_euclidean_distance(data, components)
|
||||
winning_label = pt.competitions.wtac(distance, label)
|
||||
return winning_label
|
||||
|
||||
|
||||
if __name__ == "__main__":
|
||||
train_ds = pt.datasets.Iris()
|
||||
|
||||
train_loader = torch.utils.data.DataLoader(train_ds, batch_size=32)
|
||||
|
||||
model = GMLVQ(train_ds)
|
||||
optimizer = torch.optim.Adam(model.parameters(), lr=0.05)
|
||||
criterion = pt.losses.GLVQLoss()
|
||||
|
||||
for epoch in range(200):
|
||||
correct = 0.0
|
||||
for x, y in train_loader:
|
||||
d, labels = model(x)
|
||||
loss = criterion(d, y, labels).mean(0)
|
||||
|
||||
optimizer.zero_grad()
|
||||
loss.backward()
|
||||
optimizer.step()
|
||||
|
||||
with torch.no_grad():
|
||||
y_pred = model.predict(x)
|
||||
correct += (y_pred == y).float().sum(0)
|
||||
|
||||
acc = 100 * correct / len(train_ds)
|
||||
print(f"Epoch: {epoch} Accuracy: {acc:05.02f}%")
|
@ -1,56 +0,0 @@
|
||||
"""This example script shows the usage of the new components architecture.
|
||||
|
||||
Serialization/deserialization also works as expected.
|
||||
|
||||
"""
|
||||
|
||||
import torch
|
||||
|
||||
import prototorch as pt
|
||||
|
||||
ds = pt.datasets.Iris()
|
||||
|
||||
unsupervised = pt.components.Components(
|
||||
6,
|
||||
initializer=pt.initializers.ZCI(2),
|
||||
)
|
||||
print(unsupervised())
|
||||
|
||||
prototypes = pt.components.LabeledComponents(
|
||||
(3, 2),
|
||||
components_initializer=pt.initializers.SSCI(ds),
|
||||
)
|
||||
print(prototypes())
|
||||
|
||||
components = pt.components.ReasoningComponents(
|
||||
(3, 2),
|
||||
components_initializer=pt.initializers.SSCI(ds),
|
||||
reasonings_initializer=pt.initializers.PPRI(),
|
||||
)
|
||||
print(prototypes())
|
||||
|
||||
# Test Serialization
|
||||
import io
|
||||
|
||||
save = io.BytesIO()
|
||||
torch.save(unsupervised, save)
|
||||
save.seek(0)
|
||||
serialized_unsupervised = torch.load(save)
|
||||
|
||||
assert torch.all(unsupervised.components == serialized_unsupervised.components)
|
||||
|
||||
save = io.BytesIO()
|
||||
torch.save(prototypes, save)
|
||||
save.seek(0)
|
||||
serialized_prototypes = torch.load(save)
|
||||
|
||||
assert torch.all(prototypes.components == serialized_prototypes.components)
|
||||
assert torch.all(prototypes.labels == serialized_prototypes.labels)
|
||||
|
||||
save = io.BytesIO()
|
||||
torch.save(components, save)
|
||||
save.seek(0)
|
||||
serialized_components = torch.load(save)
|
||||
|
||||
assert torch.all(components.components == serialized_components.components)
|
||||
assert torch.all(components.reasonings == serialized_components.reasonings)
|
@ -1,61 +1 @@
|
||||
"""ProtoTorch package"""
|
||||
|
||||
import pkgutil
|
||||
|
||||
import pkg_resources
|
||||
|
||||
from . import datasets # noqa: F401
|
||||
from . import nn # noqa: F401
|
||||
from . import utils # noqa: F401
|
||||
from .core import competitions # noqa: F401
|
||||
from .core import components # noqa: F401
|
||||
from .core import distances # noqa: F401
|
||||
from .core import initializers # noqa: F401
|
||||
from .core import losses # noqa: F401
|
||||
from .core import pooling # noqa: F401
|
||||
from .core import similarities # noqa: F401
|
||||
from .core import transforms # noqa: F401
|
||||
|
||||
# Core Setup
|
||||
__version__ = "0.7.6"
|
||||
|
||||
__all_core__ = [
|
||||
"competitions",
|
||||
"components",
|
||||
"core",
|
||||
"datasets",
|
||||
"distances",
|
||||
"initializers",
|
||||
"losses",
|
||||
"nn",
|
||||
"pooling",
|
||||
"similarities",
|
||||
"transforms",
|
||||
"utils",
|
||||
]
|
||||
|
||||
# Plugin Loader
|
||||
__path__ = pkgutil.extend_path(__path__, __name__)
|
||||
|
||||
|
||||
def discover_plugins():
|
||||
return {
|
||||
entry_point.name: entry_point.load()
|
||||
for entry_point in pkg_resources.iter_entry_points(
|
||||
"prototorch.plugins")
|
||||
}
|
||||
|
||||
|
||||
discovered_plugins = discover_plugins()
|
||||
locals().update(discovered_plugins)
|
||||
|
||||
# Generate combines __version__ and __all__
|
||||
version_plugins = "\n".join([
|
||||
"- " + name + ": v" + plugin.__version__
|
||||
for name, plugin in discovered_plugins.items()
|
||||
])
|
||||
if version_plugins != "":
|
||||
version_plugins = "\nPlugins: \n" + version_plugins
|
||||
|
||||
version = "core: v" + __version__ + version_plugins
|
||||
__all__ = __all_core__ + list(discovered_plugins.keys())
|
||||
__version__ = '0.1.0-dev0'
|
||||
|
@ -1,10 +0,0 @@
|
||||
"""ProtoTorch core"""
|
||||
|
||||
from .competitions import *
|
||||
from .components import *
|
||||
from .distances import *
|
||||
from .initializers import *
|
||||
from .losses import *
|
||||
from .pooling import *
|
||||
from .similarities import *
|
||||
from .transforms import *
|
@ -1,93 +0,0 @@
|
||||
"""ProtoTorch competitions"""
|
||||
|
||||
import torch
|
||||
|
||||
|
||||
def wtac(distances: torch.Tensor, labels: torch.LongTensor):
|
||||
"""Winner-Takes-All-Competition.
|
||||
|
||||
Returns the labels corresponding to the winners.
|
||||
|
||||
"""
|
||||
winning_indices = torch.min(distances, dim=1).indices
|
||||
winning_labels = labels[winning_indices].squeeze()
|
||||
return winning_labels
|
||||
|
||||
|
||||
def knnc(distances: torch.Tensor, labels: torch.LongTensor, k: int = 1):
|
||||
"""K-Nearest-Neighbors-Competition.
|
||||
|
||||
Returns the labels corresponding to the winners.
|
||||
|
||||
"""
|
||||
winning_indices = torch.topk(-distances, k=k, dim=1).indices
|
||||
winning_labels = torch.mode(labels[winning_indices], dim=1).values
|
||||
return winning_labels
|
||||
|
||||
|
||||
def cbcc(detections: torch.Tensor, reasonings: torch.Tensor):
|
||||
"""Classification-By-Components Competition.
|
||||
|
||||
Returns probability distributions over the classes.
|
||||
|
||||
`detections` must be of shape [batch_size, num_components].
|
||||
`reasonings` must be of shape [num_components, num_classes, 2].
|
||||
|
||||
"""
|
||||
A, B = reasonings.permute(2, 1, 0).clamp(0, 1)
|
||||
pk = A
|
||||
nk = (1 - A) * B
|
||||
numerator = (detections @ (pk - nk).T) + nk.sum(1)
|
||||
probs = numerator / ((pk + nk).sum(1) + 1e-8)
|
||||
return probs
|
||||
|
||||
|
||||
class WTAC(torch.nn.Module):
|
||||
"""Winner-Takes-All-Competition Layer.
|
||||
|
||||
Thin wrapper over the `wtac` function.
|
||||
|
||||
"""
|
||||
|
||||
def forward(self, distances, labels): # pylint: disable=no-self-use
|
||||
return wtac(distances, labels)
|
||||
|
||||
|
||||
class LTAC(torch.nn.Module):
|
||||
"""Loser-Takes-All-Competition Layer.
|
||||
|
||||
Thin wrapper over the `wtac` function.
|
||||
|
||||
"""
|
||||
|
||||
def forward(self, probs, labels): # pylint: disable=no-self-use
|
||||
return wtac(-1.0 * probs, labels)
|
||||
|
||||
|
||||
class KNNC(torch.nn.Module):
|
||||
"""K-Nearest-Neighbors-Competition.
|
||||
|
||||
Thin wrapper over the `knnc` function.
|
||||
|
||||
"""
|
||||
|
||||
def __init__(self, k=1, **kwargs):
|
||||
super().__init__(**kwargs)
|
||||
self.k = k
|
||||
|
||||
def forward(self, distances, labels):
|
||||
return knnc(distances, labels, k=self.k)
|
||||
|
||||
def extra_repr(self):
|
||||
return f"k: {self.k}"
|
||||
|
||||
|
||||
class CBCC(torch.nn.Module):
|
||||
"""Classification-By-Components Competition.
|
||||
|
||||
Thin wrapper over the `cbcc` function.
|
||||
|
||||
"""
|
||||
|
||||
def forward(self, detections, reasonings): # pylint: disable=no-self-use
|
||||
return cbcc(detections, reasonings)
|
@ -1,380 +0,0 @@
|
||||
"""ProtoTorch components"""
|
||||
|
||||
import inspect
|
||||
from typing import Union
|
||||
|
||||
import torch
|
||||
from torch.nn.parameter import Parameter
|
||||
|
||||
from prototorch.utils import parse_distribution
|
||||
|
||||
from .initializers import (
|
||||
AbstractClassAwareCompInitializer,
|
||||
AbstractComponentsInitializer,
|
||||
AbstractLabelsInitializer,
|
||||
AbstractReasoningsInitializer,
|
||||
LabelsInitializer,
|
||||
PurePositiveReasoningsInitializer,
|
||||
RandomReasoningsInitializer,
|
||||
)
|
||||
|
||||
|
||||
def validate_initializer(initializer, instanceof):
|
||||
"""Check if the initializer is valid."""
|
||||
if not isinstance(initializer, instanceof):
|
||||
emsg = f"`initializer` has to be an instance " \
|
||||
f"of some subtype of {instanceof}. " \
|
||||
f"You have provided: {initializer} instead. "
|
||||
helpmsg = ""
|
||||
if inspect.isclass(initializer):
|
||||
helpmsg = f"Perhaps you meant to say, {initializer.__name__}() " \
|
||||
f"with the brackets instead of just {initializer.__name__}?"
|
||||
raise TypeError(emsg + helpmsg)
|
||||
return True
|
||||
|
||||
|
||||
def gencat(ins, attr, init, *iargs, **ikwargs):
|
||||
"""Generate new items and concatenate with existing items."""
|
||||
new_items = init.generate(*iargs, **ikwargs)
|
||||
if hasattr(ins, attr):
|
||||
items = torch.cat([getattr(ins, attr), new_items])
|
||||
else:
|
||||
items = new_items
|
||||
return items, new_items
|
||||
|
||||
|
||||
def removeind(ins, attr, indices):
|
||||
"""Remove items at specified indices."""
|
||||
mask = torch.ones(len(ins), dtype=torch.bool)
|
||||
mask[indices] = False
|
||||
items = getattr(ins, attr)[mask]
|
||||
return items, mask
|
||||
|
||||
|
||||
def get_cikwargs(init, distribution):
|
||||
"""Return appropriate key-word arguments for a component initializer."""
|
||||
if isinstance(init, AbstractClassAwareCompInitializer):
|
||||
cikwargs = dict(distribution=distribution)
|
||||
else:
|
||||
distribution = parse_distribution(distribution)
|
||||
num_components = sum(distribution.values())
|
||||
cikwargs = dict(num_components=num_components)
|
||||
return cikwargs
|
||||
|
||||
|
||||
class AbstractComponents(torch.nn.Module):
|
||||
"""Abstract class for all components modules."""
|
||||
|
||||
@property
|
||||
def num_components(self):
|
||||
"""Current number of components."""
|
||||
return len(self._components)
|
||||
|
||||
@property
|
||||
def components(self):
|
||||
"""Detached Tensor containing the components."""
|
||||
return self._components.detach().cpu()
|
||||
|
||||
def _register_components(self, components):
|
||||
self.register_parameter("_components", Parameter(components))
|
||||
|
||||
def extra_repr(self):
|
||||
return f"components: (shape: {tuple(self._components.shape)})"
|
||||
|
||||
def __len__(self):
|
||||
return self.num_components
|
||||
|
||||
|
||||
class Components(AbstractComponents):
|
||||
"""A set of adaptable Tensors."""
|
||||
|
||||
def __init__(self, num_components: int,
|
||||
initializer: AbstractComponentsInitializer):
|
||||
super().__init__()
|
||||
self.add_components(num_components, initializer)
|
||||
|
||||
def add_components(self, num_components: int,
|
||||
initializer: AbstractComponentsInitializer):
|
||||
"""Generate and add new components."""
|
||||
assert validate_initializer(initializer, AbstractComponentsInitializer)
|
||||
_components, new_components = gencat(self, "_components", initializer,
|
||||
num_components)
|
||||
self._register_components(_components)
|
||||
return new_components
|
||||
|
||||
def remove_components(self, indices):
|
||||
"""Remove components at specified indices."""
|
||||
_components, mask = removeind(self, "_components", indices)
|
||||
self._register_components(_components)
|
||||
return mask
|
||||
|
||||
def forward(self):
|
||||
"""Simply return the components parameter Tensor."""
|
||||
return self._components
|
||||
|
||||
|
||||
class AbstractLabels(torch.nn.Module):
|
||||
"""Abstract class for all labels modules."""
|
||||
|
||||
@property
|
||||
def labels(self):
|
||||
return self._labels.cpu()
|
||||
|
||||
@property
|
||||
def num_labels(self):
|
||||
return len(self._labels)
|
||||
|
||||
@property
|
||||
def unique_labels(self):
|
||||
return torch.unique(self._labels)
|
||||
|
||||
@property
|
||||
def num_unique(self):
|
||||
return len(self.unique_labels)
|
||||
|
||||
@property
|
||||
def distribution(self):
|
||||
unique, counts = torch.unique(self._labels,
|
||||
sorted=True,
|
||||
return_counts=True)
|
||||
return dict(zip(unique.tolist(), counts.tolist()))
|
||||
|
||||
def _register_labels(self, labels):
|
||||
self.register_buffer("_labels", labels)
|
||||
|
||||
def extra_repr(self):
|
||||
r = f"num_labels: {self.num_labels}, num_unique: {self.num_unique}"
|
||||
if len(self.distribution) < 11: # avoid lengthy representations
|
||||
d = self.distribution
|
||||
unique, counts = list(d.keys()), list(d.values())
|
||||
r += f", unique: {unique}, counts: {counts}"
|
||||
return r
|
||||
|
||||
def __len__(self):
|
||||
return self.num_labels
|
||||
|
||||
|
||||
class Labels(AbstractLabels):
|
||||
"""A set of standalone labels."""
|
||||
|
||||
def __init__(self,
|
||||
distribution: Union[dict, list, tuple],
|
||||
initializer: AbstractLabelsInitializer = LabelsInitializer()):
|
||||
super().__init__()
|
||||
self.add_labels(distribution, initializer)
|
||||
|
||||
def add_labels(
|
||||
self,
|
||||
distribution: Union[dict, tuple, list],
|
||||
initializer: AbstractLabelsInitializer = LabelsInitializer()):
|
||||
"""Generate and add new labels."""
|
||||
assert validate_initializer(initializer, AbstractLabelsInitializer)
|
||||
_labels, new_labels = gencat(self, "_labels", initializer,
|
||||
distribution)
|
||||
self._register_labels(_labels)
|
||||
return new_labels
|
||||
|
||||
def remove_labels(self, indices):
|
||||
"""Remove labels at specified indices."""
|
||||
_labels, mask = removeind(self, "_labels", indices)
|
||||
self._register_labels(_labels)
|
||||
return mask
|
||||
|
||||
def forward(self):
|
||||
"""Simply return the labels."""
|
||||
return self._labels
|
||||
|
||||
|
||||
class LabeledComponents(AbstractComponents):
|
||||
"""A set of adaptable components and corresponding unadaptable labels."""
|
||||
|
||||
def __init__(
|
||||
self,
|
||||
distribution: Union[dict, list, tuple],
|
||||
components_initializer: AbstractComponentsInitializer,
|
||||
labels_initializer: AbstractLabelsInitializer = LabelsInitializer()):
|
||||
super().__init__()
|
||||
self.add_components(distribution, components_initializer,
|
||||
labels_initializer)
|
||||
|
||||
@property
|
||||
def distribution(self):
|
||||
unique, counts = torch.unique(self._labels,
|
||||
sorted=True,
|
||||
return_counts=True)
|
||||
return dict(zip(unique.tolist(), counts.tolist()))
|
||||
|
||||
@property
|
||||
def num_classes(self):
|
||||
return len(self.distribution.keys())
|
||||
|
||||
@property
|
||||
def labels(self):
|
||||
"""Tensor containing the component labels."""
|
||||
return self._labels.cpu()
|
||||
|
||||
def _register_labels(self, labels):
|
||||
self.register_buffer("_labels", labels)
|
||||
|
||||
def add_components(
|
||||
self,
|
||||
distribution,
|
||||
components_initializer,
|
||||
labels_initializer: AbstractLabelsInitializer = LabelsInitializer()):
|
||||
"""Generate and add new components and labels."""
|
||||
assert validate_initializer(components_initializer,
|
||||
AbstractComponentsInitializer)
|
||||
assert validate_initializer(labels_initializer,
|
||||
AbstractLabelsInitializer)
|
||||
cikwargs = get_cikwargs(components_initializer, distribution)
|
||||
_components, new_components = gencat(self, "_components",
|
||||
components_initializer,
|
||||
**cikwargs)
|
||||
_labels, new_labels = gencat(self, "_labels", labels_initializer,
|
||||
distribution)
|
||||
self._register_components(_components)
|
||||
self._register_labels(_labels)
|
||||
return new_components, new_labels
|
||||
|
||||
def remove_components(self, indices):
|
||||
"""Remove components and labels at specified indices."""
|
||||
_components, mask = removeind(self, "_components", indices)
|
||||
_labels, mask = removeind(self, "_labels", indices)
|
||||
self._register_components(_components)
|
||||
self._register_labels(_labels)
|
||||
return mask
|
||||
|
||||
def forward(self):
|
||||
"""Simply return the components parameter Tensor and labels."""
|
||||
return self._components, self._labels
|
||||
|
||||
|
||||
class Reasonings(torch.nn.Module):
|
||||
"""A set of standalone reasoning matrices.
|
||||
|
||||
The `reasonings` tensor is of shape [num_components, num_classes, 2].
|
||||
|
||||
"""
|
||||
|
||||
def __init__(
|
||||
self,
|
||||
distribution: Union[dict, list, tuple],
|
||||
initializer:
|
||||
AbstractReasoningsInitializer = RandomReasoningsInitializer(),
|
||||
):
|
||||
super().__init__()
|
||||
self.add_reasonings(distribution, initializer)
|
||||
|
||||
@property
|
||||
def num_classes(self):
|
||||
return self._reasonings.shape[1]
|
||||
|
||||
@property
|
||||
def reasonings(self):
|
||||
"""Tensor containing the reasoning matrices."""
|
||||
return self._reasonings.detach().cpu()
|
||||
|
||||
def _register_reasonings(self, reasonings):
|
||||
self.register_buffer("_reasonings", reasonings)
|
||||
|
||||
def add_reasonings(
|
||||
self,
|
||||
distribution: Union[dict, list, tuple],
|
||||
initializer:
|
||||
AbstractReasoningsInitializer = RandomReasoningsInitializer()):
|
||||
"""Generate and add new reasonings."""
|
||||
assert validate_initializer(initializer, AbstractReasoningsInitializer)
|
||||
_reasonings, new_reasonings = gencat(self, "_reasonings", initializer,
|
||||
distribution)
|
||||
self._register_reasonings(_reasonings)
|
||||
return new_reasonings
|
||||
|
||||
def remove_reasonings(self, indices):
|
||||
"""Remove reasonings at specified indices."""
|
||||
_reasonings, mask = removeind(self, "_reasonings", indices)
|
||||
self._register_reasonings(_reasonings)
|
||||
return mask
|
||||
|
||||
def forward(self):
|
||||
"""Simply return the reasonings."""
|
||||
return self._reasonings
|
||||
|
||||
|
||||
class ReasoningComponents(AbstractComponents):
|
||||
r"""A set of components and a corresponding adapatable reasoning matrices.
|
||||
|
||||
Every component has its own reasoning matrix.
|
||||
|
||||
A reasoning matrix is an Nx2 matrix, where N is the number of classes. The
|
||||
first element is called positive reasoning :math:`p`, the second negative
|
||||
reasoning :math:`n`. A components can reason in favour (positive) of a
|
||||
class, against (negative) a class or not at all (neutral).
|
||||
|
||||
It holds that :math:`0 \leq n \leq 1`, :math:`0 \leq p \leq 1` and :math:`0
|
||||
\leq n+p \leq 1`. Therefore :math:`n` and :math:`p` are two elements of a
|
||||
three element probability distribution.
|
||||
|
||||
"""
|
||||
|
||||
def __init__(
|
||||
self,
|
||||
distribution: Union[dict, list, tuple],
|
||||
components_initializer: AbstractComponentsInitializer,
|
||||
reasonings_initializer:
|
||||
AbstractReasoningsInitializer = PurePositiveReasoningsInitializer()):
|
||||
super().__init__()
|
||||
self.add_components(distribution, components_initializer,
|
||||
reasonings_initializer)
|
||||
|
||||
@property
|
||||
def num_classes(self):
|
||||
return self._reasonings.shape[1]
|
||||
|
||||
@property
|
||||
def reasonings(self):
|
||||
"""Tensor containing the reasoning matrices."""
|
||||
return self._reasonings.detach().cpu()
|
||||
|
||||
@property
|
||||
def reasoning_matrices(self):
|
||||
"""Reasoning matrices for each class."""
|
||||
with torch.no_grad():
|
||||
A, B = self._reasonings.permute(2, 1, 0).clamp(0, 1)
|
||||
pk = A
|
||||
nk = (1 - pk) * B
|
||||
ik = 1 - pk - nk
|
||||
matrices = torch.stack([pk, nk, ik], dim=-1).permute(1, 2, 0)
|
||||
return matrices.cpu()
|
||||
|
||||
def _register_reasonings(self, reasonings):
|
||||
self.register_parameter("_reasonings", Parameter(reasonings))
|
||||
|
||||
def add_components(self, distribution, components_initializer,
|
||||
reasonings_initializer: AbstractReasoningsInitializer):
|
||||
"""Generate and add new components and reasonings."""
|
||||
assert validate_initializer(components_initializer,
|
||||
AbstractComponentsInitializer)
|
||||
assert validate_initializer(reasonings_initializer,
|
||||
AbstractReasoningsInitializer)
|
||||
cikwargs = get_cikwargs(components_initializer, distribution)
|
||||
_components, new_components = gencat(self, "_components",
|
||||
components_initializer,
|
||||
**cikwargs)
|
||||
_reasonings, new_reasonings = gencat(self, "_reasonings",
|
||||
reasonings_initializer,
|
||||
distribution)
|
||||
self._register_components(_components)
|
||||
self._register_reasonings(_reasonings)
|
||||
return new_components, new_reasonings
|
||||
|
||||
def remove_components(self, indices):
|
||||
"""Remove components and reasonings at specified indices."""
|
||||
_components, mask = removeind(self, "_components", indices)
|
||||
_reasonings, mask = removeind(self, "_reasonings", indices)
|
||||
self._register_components(_components)
|
||||
self._register_reasonings(_reasonings)
|
||||
return mask
|
||||
|
||||
def forward(self):
|
||||
"""Simply return the components and reasonings."""
|
||||
return self._components, self._reasonings
|
@ -1,95 +0,0 @@
|
||||
"""ProtoTorch distances"""
|
||||
|
||||
import torch
|
||||
|
||||
|
||||
def squared_euclidean_distance(x, y):
|
||||
r"""Compute the squared Euclidean distance between :math:`\bm x` and :math:`\bm y`.
|
||||
|
||||
Compute :math:`{\langle \bm x - \bm y \rangle}_2`
|
||||
|
||||
**Alias:**
|
||||
``prototorch.functions.distances.sed``
|
||||
"""
|
||||
x, y = (arr.view(arr.size(0), -1) for arr in (x, y))
|
||||
expanded_x = x.unsqueeze(dim=1)
|
||||
batchwise_difference = y - expanded_x
|
||||
differences_raised = torch.pow(batchwise_difference, 2)
|
||||
distances = torch.sum(differences_raised, axis=2)
|
||||
return distances
|
||||
|
||||
|
||||
def euclidean_distance(x, y):
|
||||
r"""Compute the Euclidean distance between :math:`x` and :math:`y`.
|
||||
|
||||
Compute :math:`\sqrt{{\langle \bm x - \bm y \rangle}_2}`
|
||||
|
||||
:returns: Distance Tensor of shape :math:`X \times Y`
|
||||
:rtype: `torch.tensor`
|
||||
"""
|
||||
x, y = (arr.view(arr.size(0), -1) for arr in (x, y))
|
||||
distances_raised = squared_euclidean_distance(x, y)
|
||||
distances = torch.sqrt(distances_raised)
|
||||
return distances
|
||||
|
||||
|
||||
def euclidean_distance_v2(x, y):
|
||||
x, y = (arr.view(arr.size(0), -1) for arr in (x, y))
|
||||
diff = y - x.unsqueeze(1)
|
||||
pairwise_distances = (diff @ diff.permute((0, 2, 1))).sqrt()
|
||||
# Passing `dim1=-2` and `dim2=-1` to `diagonal()` takes the
|
||||
# batch diagonal. See:
|
||||
# https://pytorch.org/docs/stable/generated/torch.diagonal.html
|
||||
distances = torch.diagonal(pairwise_distances, dim1=-2, dim2=-1)
|
||||
return distances
|
||||
|
||||
|
||||
def lpnorm_distance(x, y, p):
|
||||
r"""Calculate the lp-norm between :math:`\bm x` and :math:`\bm y`.
|
||||
Also known as Minkowski distance.
|
||||
|
||||
Compute :math:`{\| \bm x - \bm y \|}_p`.
|
||||
|
||||
Calls ``torch.cdist``
|
||||
|
||||
:param p: p parameter of the lp norm
|
||||
"""
|
||||
x, y = (arr.view(arr.size(0), -1) for arr in (x, y))
|
||||
distances = torch.cdist(x, y, p=p)
|
||||
return distances
|
||||
|
||||
|
||||
def omega_distance(x, y, omega):
|
||||
r"""Omega distance.
|
||||
|
||||
Compute :math:`{\| \Omega \bm x - \Omega \bm y \|}_p`
|
||||
|
||||
:param `torch.tensor` omega: Two dimensional matrix
|
||||
"""
|
||||
x, y = (arr.view(arr.size(0), -1) for arr in (x, y))
|
||||
projected_x = x @ omega
|
||||
projected_y = y @ omega
|
||||
distances = squared_euclidean_distance(projected_x, projected_y)
|
||||
return distances
|
||||
|
||||
|
||||
def lomega_distance(x, y, omegas):
|
||||
r"""Localized Omega distance.
|
||||
|
||||
Compute :math:`{\| \Omega_k \bm x - \Omega_k \bm y_k \|}_p`
|
||||
|
||||
:param `torch.tensor` omegas: Three dimensional matrix
|
||||
"""
|
||||
x, y = (arr.view(arr.size(0), -1) for arr in (x, y))
|
||||
projected_x = x @ omegas
|
||||
projected_y = torch.diagonal(y @ omegas).T
|
||||
expanded_y = torch.unsqueeze(projected_y, dim=1)
|
||||
batchwise_difference = expanded_y - projected_x
|
||||
differences_squared = batchwise_difference**2
|
||||
distances = torch.sum(differences_squared, dim=2)
|
||||
distances = distances.permute(1, 0)
|
||||
return distances
|
||||
|
||||
|
||||
# Aliases
|
||||
sed = squared_euclidean_distance
|
@ -1,555 +0,0 @@
|
||||
"""ProtoTorch code initializers"""
|
||||
|
||||
import warnings
|
||||
from abc import ABC, abstractmethod
|
||||
from collections.abc import Iterable
|
||||
from typing import (
|
||||
Callable,
|
||||
Type,
|
||||
Union,
|
||||
)
|
||||
|
||||
import torch
|
||||
|
||||
from prototorch.utils import parse_data_arg, parse_distribution
|
||||
|
||||
|
||||
# Components
|
||||
class AbstractComponentsInitializer(ABC):
|
||||
"""Abstract class for all components initializers."""
|
||||
...
|
||||
|
||||
|
||||
class LiteralCompInitializer(AbstractComponentsInitializer):
|
||||
"""'Generate' the provided components.
|
||||
|
||||
Use this to 'generate' pre-initialized components elsewhere.
|
||||
|
||||
"""
|
||||
|
||||
def __init__(self, components):
|
||||
self.components = components
|
||||
|
||||
def generate(self, num_components: int = 0):
|
||||
"""Ignore `num_components` and simply return `self.components`."""
|
||||
provided_num_components = len(self.components)
|
||||
if provided_num_components != num_components:
|
||||
wmsg = f"The number of components ({provided_num_components}) " \
|
||||
f"provided to {self.__class__.__name__} " \
|
||||
f"does not match the expected number ({num_components})."
|
||||
warnings.warn(wmsg)
|
||||
if not isinstance(self.components, torch.Tensor):
|
||||
wmsg = f"Converting components to {torch.Tensor}..."
|
||||
warnings.warn(wmsg)
|
||||
self.components = torch.Tensor(self.components)
|
||||
return self.components
|
||||
|
||||
|
||||
class ShapeAwareCompInitializer(AbstractComponentsInitializer):
|
||||
"""Abstract class for all dimension-aware components initializers."""
|
||||
|
||||
def __init__(self, shape: Union[Iterable, int]):
|
||||
if isinstance(shape, Iterable):
|
||||
self.component_shape = tuple(shape)
|
||||
else:
|
||||
self.component_shape = (shape, )
|
||||
|
||||
@abstractmethod
|
||||
def generate(self, num_components: int):
|
||||
...
|
||||
|
||||
|
||||
class ZerosCompInitializer(ShapeAwareCompInitializer):
|
||||
"""Generate zeros corresponding to the components shape."""
|
||||
|
||||
def generate(self, num_components: int):
|
||||
components = torch.zeros((num_components, ) + self.component_shape)
|
||||
return components
|
||||
|
||||
|
||||
class OnesCompInitializer(ShapeAwareCompInitializer):
|
||||
"""Generate ones corresponding to the components shape."""
|
||||
|
||||
def generate(self, num_components: int):
|
||||
components = torch.ones((num_components, ) + self.component_shape)
|
||||
return components
|
||||
|
||||
|
||||
class FillValueCompInitializer(OnesCompInitializer):
|
||||
"""Generate components with the provided `fill_value`."""
|
||||
|
||||
def __init__(self, shape, fill_value: float = 1.0):
|
||||
super().__init__(shape)
|
||||
self.fill_value = fill_value
|
||||
|
||||
def generate(self, num_components: int):
|
||||
ones = super().generate(num_components)
|
||||
components = ones.fill_(self.fill_value)
|
||||
return components
|
||||
|
||||
|
||||
class UniformCompInitializer(OnesCompInitializer):
|
||||
"""Generate components by sampling from a continuous uniform distribution."""
|
||||
|
||||
def __init__(self, shape, minimum=0.0, maximum=1.0, scale=1.0):
|
||||
super().__init__(shape)
|
||||
self.minimum = minimum
|
||||
self.maximum = maximum
|
||||
self.scale = scale
|
||||
|
||||
def generate(self, num_components: int):
|
||||
ones = super().generate(num_components)
|
||||
components = self.scale * ones.uniform_(self.minimum, self.maximum)
|
||||
return components
|
||||
|
||||
|
||||
class RandomNormalCompInitializer(OnesCompInitializer):
|
||||
"""Generate components by sampling from a standard normal distribution."""
|
||||
|
||||
def __init__(self, shape, shift=0.0, scale=1.0):
|
||||
super().__init__(shape)
|
||||
self.shift = shift
|
||||
self.scale = scale
|
||||
|
||||
def generate(self, num_components: int):
|
||||
ones = super().generate(num_components)
|
||||
components = self.scale * (torch.randn_like(ones) + self.shift)
|
||||
return components
|
||||
|
||||
|
||||
class AbstractDataAwareCompInitializer(AbstractComponentsInitializer):
|
||||
"""Abstract class for all data-aware components initializers.
|
||||
|
||||
Components generated by data-aware components initializers inherit the shape
|
||||
of the provided data.
|
||||
|
||||
`data` has to be a torch tensor.
|
||||
|
||||
"""
|
||||
|
||||
def __init__(self,
|
||||
data: torch.Tensor,
|
||||
noise: float = 0.0,
|
||||
transform: Callable = torch.nn.Identity()):
|
||||
self.data = data
|
||||
self.noise = noise
|
||||
self.transform = transform
|
||||
|
||||
def generate_end_hook(self, samples):
|
||||
drift = torch.rand_like(samples) * self.noise
|
||||
components = self.transform(samples + drift)
|
||||
return components
|
||||
|
||||
@abstractmethod
|
||||
def generate(self, num_components: int):
|
||||
...
|
||||
return self.generate_end_hook(...)
|
||||
|
||||
def __del__(self):
|
||||
del self.data
|
||||
|
||||
|
||||
class DataAwareCompInitializer(AbstractDataAwareCompInitializer):
|
||||
"""'Generate' the components from the provided data."""
|
||||
|
||||
def generate(self, num_components: int = 0):
|
||||
"""Ignore `num_components` and simply return transformed `self.data`."""
|
||||
components = self.generate_end_hook(self.data)
|
||||
return components
|
||||
|
||||
|
||||
class SelectionCompInitializer(AbstractDataAwareCompInitializer):
|
||||
"""Generate components by uniformly sampling from the provided data."""
|
||||
|
||||
def generate(self, num_components: int):
|
||||
indices = torch.LongTensor(num_components).random_(0, len(self.data))
|
||||
samples = self.data[indices]
|
||||
components = self.generate_end_hook(samples)
|
||||
return components
|
||||
|
||||
|
||||
class MeanCompInitializer(AbstractDataAwareCompInitializer):
|
||||
"""Generate components by computing the mean of the provided data."""
|
||||
|
||||
def generate(self, num_components: int):
|
||||
mean = self.data.mean(dim=0)
|
||||
repeat_dim = [num_components] + [1] * len(mean.shape)
|
||||
samples = mean.repeat(repeat_dim)
|
||||
components = self.generate_end_hook(samples)
|
||||
return components
|
||||
|
||||
|
||||
class AbstractClassAwareCompInitializer(AbstractComponentsInitializer):
|
||||
"""Abstract class for all class-aware components initializers.
|
||||
|
||||
Components generated by class-aware components initializers inherit the shape
|
||||
of the provided data.
|
||||
|
||||
`data` could be a torch Dataset or DataLoader or a list/tuple of data and
|
||||
target tensors.
|
||||
|
||||
"""
|
||||
|
||||
def __init__(self,
|
||||
data,
|
||||
noise: float = 0.0,
|
||||
transform: Callable = torch.nn.Identity()):
|
||||
self.data, self.targets = parse_data_arg(data)
|
||||
self.noise = noise
|
||||
self.transform = transform
|
||||
self.clabels = torch.unique(self.targets).int().tolist()
|
||||
self.num_classes = len(self.clabels)
|
||||
|
||||
def generate_end_hook(self, samples):
|
||||
drift = torch.rand_like(samples) * self.noise
|
||||
components = self.transform(samples + drift)
|
||||
return components
|
||||
|
||||
@abstractmethod
|
||||
def generate(self, distribution: Union[dict, list, tuple]):
|
||||
...
|
||||
return self.generate_end_hook(...)
|
||||
|
||||
def __del__(self):
|
||||
del self.data
|
||||
del self.targets
|
||||
|
||||
|
||||
class ClassAwareCompInitializer(AbstractClassAwareCompInitializer):
|
||||
"""'Generate' components from provided data and requested distribution."""
|
||||
|
||||
def generate(self, distribution: Union[dict, list, tuple]):
|
||||
"""Ignore `distribution` and simply return transformed `self.data`."""
|
||||
components = self.generate_end_hook(self.data)
|
||||
return components
|
||||
|
||||
|
||||
class AbstractStratifiedCompInitializer(AbstractClassAwareCompInitializer):
|
||||
"""Abstract class for all stratified components initializers."""
|
||||
|
||||
@property
|
||||
@abstractmethod
|
||||
def subinit_type(self) -> Type[AbstractDataAwareCompInitializer]:
|
||||
...
|
||||
|
||||
def generate(self, distribution: Union[dict, list, tuple]):
|
||||
distribution = parse_distribution(distribution)
|
||||
components = torch.tensor([])
|
||||
for k, v in distribution.items():
|
||||
stratified_data = self.data[self.targets == k]
|
||||
if len(stratified_data) == 0:
|
||||
raise ValueError(f"No data available for class {k}.")
|
||||
initializer = self.subinit_type(
|
||||
stratified_data,
|
||||
noise=self.noise,
|
||||
transform=self.transform,
|
||||
)
|
||||
samples = initializer.generate(num_components=v)
|
||||
components = torch.cat([components, samples])
|
||||
return components
|
||||
|
||||
|
||||
class StratifiedSelectionCompInitializer(AbstractStratifiedCompInitializer):
|
||||
"""Generate components using stratified sampling from the provided data."""
|
||||
|
||||
@property
|
||||
def subinit_type(self):
|
||||
return SelectionCompInitializer
|
||||
|
||||
|
||||
class StratifiedMeanCompInitializer(AbstractStratifiedCompInitializer):
|
||||
"""Generate components at stratified means of the provided data."""
|
||||
|
||||
@property
|
||||
def subinit_type(self):
|
||||
return MeanCompInitializer
|
||||
|
||||
|
||||
# Labels
|
||||
class AbstractLabelsInitializer(ABC):
|
||||
"""Abstract class for all labels initializers."""
|
||||
|
||||
@abstractmethod
|
||||
def generate(self, distribution: Union[dict, list, tuple]):
|
||||
...
|
||||
|
||||
|
||||
class LiteralLabelsInitializer(AbstractLabelsInitializer):
|
||||
"""'Generate' the provided labels.
|
||||
|
||||
Use this to 'generate' pre-initialized labels elsewhere.
|
||||
|
||||
"""
|
||||
|
||||
def __init__(self, labels):
|
||||
self.labels = labels
|
||||
|
||||
def generate(self, distribution: Union[dict, list, tuple]):
|
||||
"""Ignore `distribution` and simply return `self.labels`.
|
||||
|
||||
Convert to long tensor, if necessary.
|
||||
"""
|
||||
labels = self.labels
|
||||
if not isinstance(labels, torch.LongTensor):
|
||||
wmsg = f"Converting labels to {torch.LongTensor}..."
|
||||
warnings.warn(wmsg)
|
||||
labels = torch.LongTensor(labels)
|
||||
return labels
|
||||
|
||||
|
||||
class DataAwareLabelsInitializer(AbstractLabelsInitializer):
|
||||
"""'Generate' the labels from a torch Dataset."""
|
||||
|
||||
def __init__(self, data):
|
||||
self.data, self.targets = parse_data_arg(data)
|
||||
|
||||
def generate(self, distribution: Union[dict, list, tuple]):
|
||||
"""Ignore `num_components` and simply return `self.targets`."""
|
||||
return self.targets
|
||||
|
||||
|
||||
class LabelsInitializer(AbstractLabelsInitializer):
|
||||
"""Generate labels from `distribution`."""
|
||||
|
||||
def generate(self, distribution: Union[dict, list, tuple]):
|
||||
distribution = parse_distribution(distribution)
|
||||
labels_list = []
|
||||
for k, v in distribution.items():
|
||||
labels_list.extend([k] * v)
|
||||
labels = torch.LongTensor(labels_list)
|
||||
return labels
|
||||
|
||||
|
||||
class OneHotLabelsInitializer(LabelsInitializer):
|
||||
"""Generate one-hot-encoded labels from `distribution`."""
|
||||
|
||||
def generate(self, distribution: Union[dict, list, tuple]):
|
||||
distribution = parse_distribution(distribution)
|
||||
num_classes = len(distribution.keys())
|
||||
# this breaks if class labels are not [0,...,nclasses]
|
||||
labels = torch.eye(num_classes)[super().generate(distribution)]
|
||||
return labels
|
||||
|
||||
|
||||
# Reasonings
|
||||
def compute_distribution_shape(distribution):
|
||||
distribution = parse_distribution(distribution)
|
||||
num_components = sum(distribution.values())
|
||||
num_classes = len(distribution.keys())
|
||||
return (num_components, num_classes, 2)
|
||||
|
||||
|
||||
class AbstractReasoningsInitializer(ABC):
|
||||
"""Abstract class for all reasonings initializers."""
|
||||
|
||||
def __init__(self, components_first: bool = True):
|
||||
self.components_first = components_first
|
||||
|
||||
def generate_end_hook(self, reasonings):
|
||||
if not self.components_first:
|
||||
reasonings = reasonings.permute(2, 1, 0)
|
||||
return reasonings
|
||||
|
||||
@abstractmethod
|
||||
def generate(self, distribution: Union[dict, list, tuple]):
|
||||
...
|
||||
return self.generate_end_hook(...)
|
||||
|
||||
|
||||
class LiteralReasoningsInitializer(AbstractReasoningsInitializer):
|
||||
"""'Generate' the provided reasonings.
|
||||
|
||||
Use this to 'generate' pre-initialized reasonings elsewhere.
|
||||
|
||||
"""
|
||||
|
||||
def __init__(self, reasonings, **kwargs):
|
||||
super().__init__(**kwargs)
|
||||
self.reasonings = reasonings
|
||||
|
||||
def generate(self, distribution: Union[dict, list, tuple]):
|
||||
"""Ignore `distributuion` and simply return self.reasonings."""
|
||||
reasonings = self.reasonings
|
||||
if not isinstance(reasonings, torch.Tensor):
|
||||
wmsg = f"Converting reasonings to {torch.Tensor}..."
|
||||
warnings.warn(wmsg)
|
||||
reasonings = torch.Tensor(reasonings)
|
||||
reasonings = self.generate_end_hook(reasonings)
|
||||
return reasonings
|
||||
|
||||
|
||||
class ZerosReasoningsInitializer(AbstractReasoningsInitializer):
|
||||
"""Reasonings are all initialized with zeros."""
|
||||
|
||||
def generate(self, distribution: Union[dict, list, tuple]):
|
||||
shape = compute_distribution_shape(distribution)
|
||||
reasonings = torch.zeros(*shape)
|
||||
reasonings = self.generate_end_hook(reasonings)
|
||||
return reasonings
|
||||
|
||||
|
||||
class OnesReasoningsInitializer(AbstractReasoningsInitializer):
|
||||
"""Reasonings are all initialized with ones."""
|
||||
|
||||
def generate(self, distribution: Union[dict, list, tuple]):
|
||||
shape = compute_distribution_shape(distribution)
|
||||
reasonings = torch.ones(*shape)
|
||||
reasonings = self.generate_end_hook(reasonings)
|
||||
return reasonings
|
||||
|
||||
|
||||
class RandomReasoningsInitializer(AbstractReasoningsInitializer):
|
||||
"""Reasonings are randomly initialized."""
|
||||
|
||||
def __init__(self, minimum=0.4, maximum=0.6, **kwargs):
|
||||
super().__init__(**kwargs)
|
||||
self.minimum = minimum
|
||||
self.maximum = maximum
|
||||
|
||||
def generate(self, distribution: Union[dict, list, tuple]):
|
||||
shape = compute_distribution_shape(distribution)
|
||||
reasonings = torch.ones(*shape).uniform_(self.minimum, self.maximum)
|
||||
reasonings = self.generate_end_hook(reasonings)
|
||||
return reasonings
|
||||
|
||||
|
||||
class PurePositiveReasoningsInitializer(AbstractReasoningsInitializer):
|
||||
"""Each component reasons positively for exactly one class."""
|
||||
|
||||
def generate(self, distribution: Union[dict, list, tuple]):
|
||||
num_components, num_classes, _ = compute_distribution_shape(
|
||||
distribution)
|
||||
A = OneHotLabelsInitializer().generate(distribution)
|
||||
B = torch.zeros(num_components, num_classes)
|
||||
reasonings = torch.stack([A, B], dim=-1)
|
||||
reasonings = self.generate_end_hook(reasonings)
|
||||
return reasonings
|
||||
|
||||
|
||||
# Transforms
|
||||
class AbstractTransformInitializer(ABC):
|
||||
"""Abstract class for all transform initializers."""
|
||||
...
|
||||
|
||||
|
||||
class AbstractLinearTransformInitializer(AbstractTransformInitializer):
|
||||
"""Abstract class for all linear transform initializers."""
|
||||
|
||||
def __init__(self, out_dim_first: bool = False):
|
||||
self.out_dim_first = out_dim_first
|
||||
|
||||
def generate_end_hook(self, weights):
|
||||
if self.out_dim_first:
|
||||
weights = weights.permute(1, 0)
|
||||
return weights
|
||||
|
||||
@abstractmethod
|
||||
def generate(self, in_dim: int, out_dim: int):
|
||||
...
|
||||
return self.generate_end_hook(...)
|
||||
|
||||
|
||||
class ZerosLinearTransformInitializer(AbstractLinearTransformInitializer):
|
||||
"""Initialize a matrix with zeros."""
|
||||
|
||||
def generate(self, in_dim: int, out_dim: int):
|
||||
weights = torch.zeros(in_dim, out_dim)
|
||||
return self.generate_end_hook(weights)
|
||||
|
||||
|
||||
class OnesLinearTransformInitializer(AbstractLinearTransformInitializer):
|
||||
"""Initialize a matrix with ones."""
|
||||
|
||||
def generate(self, in_dim: int, out_dim: int):
|
||||
weights = torch.ones(in_dim, out_dim)
|
||||
return self.generate_end_hook(weights)
|
||||
|
||||
|
||||
class RandomLinearTransformInitializer(AbstractLinearTransformInitializer):
|
||||
"""Initialize a matrix with random values."""
|
||||
|
||||
def generate(self, in_dim: int, out_dim: int):
|
||||
weights = torch.rand(in_dim, out_dim)
|
||||
return self.generate_end_hook(weights)
|
||||
|
||||
|
||||
class EyeLinearTransformInitializer(AbstractLinearTransformInitializer):
|
||||
"""Initialize a matrix with the largest possible identity matrix."""
|
||||
|
||||
def generate(self, in_dim: int, out_dim: int):
|
||||
weights = torch.zeros(in_dim, out_dim)
|
||||
I = torch.eye(min(in_dim, out_dim))
|
||||
weights[:I.shape[0], :I.shape[1]] = I
|
||||
return self.generate_end_hook(weights)
|
||||
|
||||
|
||||
class AbstractDataAwareLTInitializer(AbstractLinearTransformInitializer):
|
||||
"""Abstract class for all data-aware linear transform initializers."""
|
||||
|
||||
def __init__(self,
|
||||
data: torch.Tensor,
|
||||
noise: float = 0.0,
|
||||
transform: Callable = torch.nn.Identity(),
|
||||
out_dim_first: bool = False):
|
||||
super().__init__(out_dim_first)
|
||||
self.data = data
|
||||
self.noise = noise
|
||||
self.transform = transform
|
||||
|
||||
def generate_end_hook(self, weights: torch.Tensor):
|
||||
drift = torch.rand_like(weights) * self.noise
|
||||
weights = self.transform(weights + drift)
|
||||
if self.out_dim_first:
|
||||
weights = weights.permute(1, 0)
|
||||
return weights
|
||||
|
||||
|
||||
class PCALinearTransformInitializer(AbstractDataAwareLTInitializer):
|
||||
"""Initialize a matrix with Eigenvectors from the data."""
|
||||
|
||||
def generate(self, in_dim: int, out_dim: int):
|
||||
_, _, weights = torch.pca_lowrank(self.data, q=out_dim)
|
||||
return self.generate_end_hook(weights)
|
||||
|
||||
|
||||
class LiteralLinearTransformInitializer(AbstractDataAwareLTInitializer):
|
||||
"""'Generate' the provided weights."""
|
||||
|
||||
def generate(self, in_dim: int, out_dim: int):
|
||||
return self.generate_end_hook(self.data)
|
||||
|
||||
|
||||
# Aliases - Components
|
||||
CACI = ClassAwareCompInitializer
|
||||
DACI = DataAwareCompInitializer
|
||||
FVCI = FillValueCompInitializer
|
||||
LCI = LiteralCompInitializer
|
||||
MCI = MeanCompInitializer
|
||||
OCI = OnesCompInitializer
|
||||
RNCI = RandomNormalCompInitializer
|
||||
SCI = SelectionCompInitializer
|
||||
SMCI = StratifiedMeanCompInitializer
|
||||
SSCI = StratifiedSelectionCompInitializer
|
||||
UCI = UniformCompInitializer
|
||||
ZCI = ZerosCompInitializer
|
||||
|
||||
# Aliases - Labels
|
||||
DLI = DataAwareLabelsInitializer
|
||||
LI = LabelsInitializer
|
||||
LLI = LiteralLabelsInitializer
|
||||
OHLI = OneHotLabelsInitializer
|
||||
|
||||
# Aliases - Reasonings
|
||||
LRI = LiteralReasoningsInitializer
|
||||
ORI = OnesReasoningsInitializer
|
||||
PPRI = PurePositiveReasoningsInitializer
|
||||
RRI = RandomReasoningsInitializer
|
||||
ZRI = ZerosReasoningsInitializer
|
||||
|
||||
# Aliases - Transforms
|
||||
ELTI = Eye = EyeLinearTransformInitializer
|
||||
OLTI = OnesLinearTransformInitializer
|
||||
RLTI = RandomLinearTransformInitializer
|
||||
ZLTI = ZerosLinearTransformInitializer
|
||||
PCALTI = PCALinearTransformInitializer
|
||||
LLTI = LiteralLinearTransformInitializer
|
@ -1,184 +0,0 @@
|
||||
"""ProtoTorch losses"""
|
||||
|
||||
import torch
|
||||
|
||||
from prototorch.nn.activations import get_activation
|
||||
|
||||
|
||||
# Helpers
|
||||
def _get_matcher(targets, labels):
|
||||
"""Returns a boolean tensor."""
|
||||
matcher = torch.eq(targets.unsqueeze(dim=1), labels)
|
||||
if labels.ndim == 2:
|
||||
# if the labels are one-hot vectors
|
||||
num_classes = targets.size()[1]
|
||||
matcher = torch.eq(torch.sum(matcher, dim=-1), num_classes)
|
||||
return matcher
|
||||
|
||||
|
||||
def _get_dp_dm(distances, targets, plabels, with_indices=False):
|
||||
"""Returns the d+ and d- values for a batch of distances."""
|
||||
matcher = _get_matcher(targets, plabels)
|
||||
not_matcher = torch.bitwise_not(matcher)
|
||||
|
||||
inf = torch.full_like(distances, fill_value=float("inf"))
|
||||
d_matching = torch.where(matcher, distances, inf)
|
||||
d_unmatching = torch.where(not_matcher, distances, inf)
|
||||
dp = torch.min(d_matching, dim=-1, keepdim=True)
|
||||
dm = torch.min(d_unmatching, dim=-1, keepdim=True)
|
||||
if with_indices:
|
||||
return dp, dm
|
||||
return dp.values, dm.values
|
||||
|
||||
|
||||
# GLVQ
|
||||
def glvq_loss(distances, target_labels, prototype_labels):
|
||||
"""GLVQ loss function with support for one-hot labels."""
|
||||
dp, dm = _get_dp_dm(distances, target_labels, prototype_labels)
|
||||
mu = (dp - dm) / (dp + dm)
|
||||
return mu
|
||||
|
||||
|
||||
def lvq1_loss(distances, target_labels, prototype_labels):
|
||||
"""LVQ1 loss function with support for one-hot labels.
|
||||
|
||||
See Section 4 [Sado&Yamada]
|
||||
https://papers.nips.cc/paper/1995/file/9c3b1830513cc3b8fc4b76635d32e692-Paper.pdf
|
||||
"""
|
||||
dp, dm = _get_dp_dm(distances, target_labels, prototype_labels)
|
||||
mu = dp
|
||||
mu[dp > dm] = -dm[dp > dm]
|
||||
return mu
|
||||
|
||||
|
||||
def lvq21_loss(distances, target_labels, prototype_labels):
|
||||
"""LVQ2.1 loss function with support for one-hot labels.
|
||||
|
||||
See Section 4 [Sado&Yamada]
|
||||
https://papers.nips.cc/paper/1995/file/9c3b1830513cc3b8fc4b76635d32e692-Paper.pdf
|
||||
"""
|
||||
dp, dm = _get_dp_dm(distances, target_labels, prototype_labels)
|
||||
mu = dp - dm
|
||||
|
||||
return mu
|
||||
|
||||
|
||||
# Probabilistic
|
||||
def _get_class_probabilities(probabilities, targets, prototype_labels):
|
||||
# Create Label Mapping
|
||||
uniques = prototype_labels.unique(sorted=True).tolist()
|
||||
key_val = {key: val for key, val in zip(uniques, range(len(uniques)))}
|
||||
|
||||
target_indices = torch.LongTensor(list(map(key_val.get, targets.tolist())))
|
||||
|
||||
whole = probabilities.sum(dim=1)
|
||||
correct = probabilities[torch.arange(len(probabilities)), target_indices]
|
||||
wrong = whole - correct
|
||||
|
||||
return whole, correct, wrong
|
||||
|
||||
|
||||
def nllr_loss(probabilities, targets, prototype_labels):
|
||||
"""Compute the Negative Log-Likelihood Ratio loss."""
|
||||
_, correct, wrong = _get_class_probabilities(probabilities, targets,
|
||||
prototype_labels)
|
||||
|
||||
likelihood = correct / wrong
|
||||
log_likelihood = torch.log(likelihood)
|
||||
return -1.0 * log_likelihood
|
||||
|
||||
|
||||
def rslvq_loss(probabilities, targets, prototype_labels):
|
||||
"""Compute the Robust Soft Learning Vector Quantization (RSLVQ) loss."""
|
||||
whole, correct, _ = _get_class_probabilities(probabilities, targets,
|
||||
prototype_labels)
|
||||
|
||||
likelihood = correct / whole
|
||||
log_likelihood = torch.log(likelihood)
|
||||
return -1.0 * log_likelihood
|
||||
|
||||
|
||||
def margin_loss(y_pred, y_true, margin=0.3):
|
||||
"""Compute the margin loss."""
|
||||
dp = torch.sum(y_true * y_pred, dim=-1)
|
||||
dm = torch.max(y_pred - y_true, dim=-1).values
|
||||
return torch.nn.functional.relu(dm - dp + margin)
|
||||
|
||||
|
||||
class GLVQLoss(torch.nn.Module):
|
||||
|
||||
def __init__(self,
|
||||
margin=0.0,
|
||||
transfer_fn="identity",
|
||||
beta=10,
|
||||
add_dp=False,
|
||||
**kwargs):
|
||||
super().__init__(**kwargs)
|
||||
self.margin = margin
|
||||
self.transfer_fn = get_activation(transfer_fn)
|
||||
self.beta = torch.tensor(beta)
|
||||
self.add_dp = add_dp
|
||||
|
||||
def forward(self, outputs, targets, plabels):
|
||||
# mu = glvq_loss(outputs, targets, plabels)
|
||||
dp, dm = _get_dp_dm(outputs, targets, plabels)
|
||||
mu = (dp - dm) / (dp + dm)
|
||||
if self.add_dp:
|
||||
mu = mu + dp
|
||||
batch_loss = self.transfer_fn(mu + self.margin, beta=self.beta)
|
||||
return batch_loss.sum()
|
||||
|
||||
|
||||
class MarginLoss(torch.nn.modules.loss._Loss):
|
||||
|
||||
def __init__(self,
|
||||
margin=0.3,
|
||||
size_average=None,
|
||||
reduce=None,
|
||||
reduction="mean"):
|
||||
super().__init__(size_average, reduce, reduction)
|
||||
self.margin = margin
|
||||
|
||||
def forward(self, y_pred, y_true):
|
||||
return margin_loss(y_pred, y_true, self.margin)
|
||||
|
||||
|
||||
class NeuralGasEnergy(torch.nn.Module):
|
||||
|
||||
def __init__(self, lm, **kwargs):
|
||||
super().__init__(**kwargs)
|
||||
self.lm = lm
|
||||
|
||||
def forward(self, d):
|
||||
order = torch.argsort(d, dim=1)
|
||||
ranks = torch.argsort(order, dim=1)
|
||||
cost = torch.sum(self._nghood_fn(ranks, self.lm) * d)
|
||||
|
||||
return cost, order
|
||||
|
||||
def extra_repr(self):
|
||||
return f"lambda: {self.lm}"
|
||||
|
||||
@staticmethod
|
||||
def _nghood_fn(rankings, lm):
|
||||
return torch.exp(-rankings / lm)
|
||||
|
||||
|
||||
class GrowingNeuralGasEnergy(NeuralGasEnergy):
|
||||
|
||||
def __init__(self, topology_layer, **kwargs):
|
||||
super().__init__(**kwargs)
|
||||
self.topology_layer = topology_layer
|
||||
|
||||
@staticmethod
|
||||
def _nghood_fn(rankings, topology):
|
||||
winner = rankings[:, 0]
|
||||
|
||||
weights = torch.zeros_like(rankings, dtype=torch.float)
|
||||
weights[torch.arange(rankings.shape[0]), winner] = 1.0
|
||||
|
||||
neighbours = topology.get_neighbours(winner)
|
||||
|
||||
weights[neighbours] = 0.1
|
||||
|
||||
return weights
|
@ -1,108 +0,0 @@
|
||||
"""ProtoTorch pooling"""
|
||||
|
||||
from typing import Callable
|
||||
|
||||
import torch
|
||||
|
||||
|
||||
def stratify_with(values: torch.Tensor,
|
||||
labels: torch.LongTensor,
|
||||
fn: Callable,
|
||||
fill_value: float = 0.0) -> (torch.Tensor):
|
||||
"""Apply an arbitrary stratification strategy on the columns on `values`.
|
||||
|
||||
The outputs correspond to sorted labels.
|
||||
"""
|
||||
clabels = torch.unique(labels, dim=0, sorted=True)
|
||||
num_classes = clabels.size()[0]
|
||||
if values.size()[1] == num_classes:
|
||||
# skip if stratification is trivial
|
||||
return values
|
||||
batch_size = values.size()[0]
|
||||
winning_values = torch.zeros(num_classes, batch_size, device=labels.device)
|
||||
filler = torch.full_like(values.T, fill_value=fill_value)
|
||||
for i, cl in enumerate(clabels):
|
||||
matcher = torch.eq(labels.unsqueeze(dim=1), cl)
|
||||
if labels.ndim == 2:
|
||||
# if the labels are one-hot vectors
|
||||
matcher = torch.eq(torch.sum(matcher, dim=-1), num_classes)
|
||||
cdists = torch.where(matcher, values.T, filler).T
|
||||
winning_values[i] = fn(cdists)
|
||||
if labels.ndim == 2:
|
||||
# Transpose to return with `batch_size` first and
|
||||
# reverse the columns to fix the ordering of the classes
|
||||
return torch.flip(winning_values.T, dims=(1, ))
|
||||
|
||||
return winning_values.T # return with `batch_size` first
|
||||
|
||||
|
||||
def stratified_sum_pooling(values: torch.Tensor,
|
||||
labels: torch.LongTensor) -> (torch.Tensor):
|
||||
"""Group-wise sum."""
|
||||
winning_values = stratify_with(
|
||||
values,
|
||||
labels,
|
||||
fn=lambda x: torch.sum(x, dim=1, keepdim=True).squeeze(),
|
||||
fill_value=0.0)
|
||||
return winning_values
|
||||
|
||||
|
||||
def stratified_min_pooling(values: torch.Tensor,
|
||||
labels: torch.LongTensor) -> (torch.Tensor):
|
||||
"""Group-wise minimum."""
|
||||
winning_values = stratify_with(
|
||||
values,
|
||||
labels,
|
||||
fn=lambda x: torch.min(x, dim=1, keepdim=True).values.squeeze(),
|
||||
fill_value=float("inf"))
|
||||
return winning_values
|
||||
|
||||
|
||||
def stratified_max_pooling(values: torch.Tensor,
|
||||
labels: torch.LongTensor) -> (torch.Tensor):
|
||||
"""Group-wise maximum."""
|
||||
winning_values = stratify_with(
|
||||
values,
|
||||
labels,
|
||||
fn=lambda x: torch.max(x, dim=1, keepdim=True).values.squeeze(),
|
||||
fill_value=-1.0 * float("inf"))
|
||||
return winning_values
|
||||
|
||||
|
||||
def stratified_prod_pooling(values: torch.Tensor,
|
||||
labels: torch.LongTensor) -> (torch.Tensor):
|
||||
"""Group-wise maximum."""
|
||||
winning_values = stratify_with(
|
||||
values,
|
||||
labels,
|
||||
fn=lambda x: torch.prod(x, dim=1, keepdim=True).squeeze(),
|
||||
fill_value=1.0)
|
||||
return winning_values
|
||||
|
||||
|
||||
class StratifiedSumPooling(torch.nn.Module):
|
||||
"""Thin wrapper over the `stratified_sum_pooling` function."""
|
||||
|
||||
def forward(self, values, labels): # pylint: disable=no-self-use
|
||||
return stratified_sum_pooling(values, labels)
|
||||
|
||||
|
||||
class StratifiedProdPooling(torch.nn.Module):
|
||||
"""Thin wrapper over the `stratified_prod_pooling` function."""
|
||||
|
||||
def forward(self, values, labels): # pylint: disable=no-self-use
|
||||
return stratified_prod_pooling(values, labels)
|
||||
|
||||
|
||||
class StratifiedMinPooling(torch.nn.Module):
|
||||
"""Thin wrapper over the `stratified_min_pooling` function."""
|
||||
|
||||
def forward(self, values, labels): # pylint: disable=no-self-use
|
||||
return stratified_min_pooling(values, labels)
|
||||
|
||||
|
||||
class StratifiedMaxPooling(torch.nn.Module):
|
||||
"""Thin wrapper over the `stratified_max_pooling` function."""
|
||||
|
||||
def forward(self, values, labels): # pylint: disable=no-self-use
|
||||
return stratified_max_pooling(values, labels)
|
@ -1,31 +0,0 @@
|
||||
"""ProtoTorch similarities."""
|
||||
|
||||
import torch
|
||||
|
||||
from .distances import euclidean_distance
|
||||
|
||||
|
||||
def gaussian(x, variance=1.0):
|
||||
return torch.exp(-(x * x) / (2 * variance))
|
||||
|
||||
|
||||
def euclidean_similarity(x, y, variance=1.0):
|
||||
distances = euclidean_distance(x, y)
|
||||
similarities = gaussian(distances, variance)
|
||||
return similarities
|
||||
|
||||
|
||||
def cosine_similarity(x, y):
|
||||
"""Compute the cosine similarity between :math:`x` and :math:`y`.
|
||||
|
||||
Expected dimension of x is 2.
|
||||
Expected dimension of y is 2.
|
||||
"""
|
||||
x, y = (arr.view(arr.size(0), -1) for arr in (x, y))
|
||||
norm_x = x.pow(2).sum(1).sqrt()
|
||||
norm_y = y.pow(2).sum(1).sqrt()
|
||||
norm_mat = norm_x.unsqueeze(-1) @ norm_y.unsqueeze(-1).T
|
||||
epsilon = torch.finfo(norm_mat.dtype).eps
|
||||
norm_mat.clamp_(min=epsilon)
|
||||
similarities = (x @ y.T) / norm_mat
|
||||
return similarities
|
@ -1,47 +0,0 @@
|
||||
"""ProtoTorch transforms"""
|
||||
|
||||
import torch
|
||||
from torch.nn.parameter import Parameter
|
||||
|
||||
from .initializers import (
|
||||
AbstractLinearTransformInitializer,
|
||||
EyeLinearTransformInitializer,
|
||||
)
|
||||
|
||||
|
||||
class LinearTransform(torch.nn.Module):
|
||||
|
||||
def __init__(
|
||||
self,
|
||||
in_dim: int,
|
||||
out_dim: int,
|
||||
initializer:
|
||||
AbstractLinearTransformInitializer = EyeLinearTransformInitializer()):
|
||||
super().__init__()
|
||||
self.set_weights(in_dim, out_dim, initializer)
|
||||
|
||||
@property
|
||||
def weights(self):
|
||||
return self._weights.detach().cpu()
|
||||
|
||||
def _register_weights(self, weights):
|
||||
self.register_parameter("_weights", Parameter(weights))
|
||||
|
||||
def set_weights(
|
||||
self,
|
||||
in_dim: int,
|
||||
out_dim: int,
|
||||
initializer:
|
||||
AbstractLinearTransformInitializer = EyeLinearTransformInitializer()):
|
||||
weights = initializer.generate(in_dim, out_dim)
|
||||
self._register_weights(weights)
|
||||
|
||||
def forward(self, x):
|
||||
return x @ self._weights
|
||||
|
||||
def extra_repr(self):
|
||||
return f"weights: (shape: {tuple(self._weights.shape)})"
|
||||
|
||||
|
||||
# Aliases
|
||||
Omega = LinearTransform
|
@ -1,13 +0,0 @@
|
||||
"""ProtoTorch datasets"""
|
||||
|
||||
from .abstract import CSVDataset, NumpyDataset
|
||||
from .sklearn import (
|
||||
Blobs,
|
||||
Circles,
|
||||
Iris,
|
||||
Moons,
|
||||
Random,
|
||||
)
|
||||
from .spiral import Spiral
|
||||
from .tecator import Tecator
|
||||
from .xor import XOR
|
@ -1,115 +0,0 @@
|
||||
"""ProtoTorch abstract dataset classes
|
||||
|
||||
Based on `torchvision.VisionDataset` and `torchvision.MNIST`.
|
||||
|
||||
For the original code, see:
|
||||
https://github.com/pytorch/vision/blob/master/torchvision/datasets/vision.py
|
||||
https://github.com/pytorch/vision/blob/master/torchvision/datasets/mnist.py
|
||||
|
||||
"""
|
||||
|
||||
import os
|
||||
|
||||
import numpy as np
|
||||
import torch
|
||||
|
||||
|
||||
class Dataset(torch.utils.data.Dataset):
|
||||
"""Abstract dataset class to be inherited."""
|
||||
|
||||
_repr_indent = 2
|
||||
|
||||
def __init__(self, root):
|
||||
if isinstance(root, str):
|
||||
root = os.path.expanduser(root)
|
||||
self.root = root
|
||||
|
||||
def __getitem__(self, index):
|
||||
raise NotImplementedError
|
||||
|
||||
def __len__(self):
|
||||
raise NotImplementedError
|
||||
|
||||
|
||||
class ProtoDataset(Dataset):
|
||||
"""Abstract dataset class to be inherited."""
|
||||
|
||||
training_file = "training.pt"
|
||||
test_file = "test.pt"
|
||||
|
||||
def __init__(self, root="", train=True, download=True, verbose=True):
|
||||
super().__init__(root)
|
||||
self.train = train # training set or test set
|
||||
self.verbose = verbose
|
||||
|
||||
if download:
|
||||
self._download()
|
||||
|
||||
if not self._check_exists():
|
||||
raise RuntimeError("Dataset not found. "
|
||||
"You can use download=True to download it")
|
||||
|
||||
data_file = self.training_file if self.train else self.test_file
|
||||
|
||||
self.data, self.targets = torch.load(
|
||||
os.path.join(self.processed_folder, data_file))
|
||||
|
||||
@property
|
||||
def raw_folder(self):
|
||||
return os.path.join(self.root, self.__class__.__name__, "raw")
|
||||
|
||||
@property
|
||||
def processed_folder(self):
|
||||
return os.path.join(self.root, self.__class__.__name__, "processed")
|
||||
|
||||
@property
|
||||
def class_to_idx(self):
|
||||
return {_class: i for i, _class in enumerate(self.classes)}
|
||||
|
||||
def _check_exists(self):
|
||||
return os.path.exists(
|
||||
os.path.join(
|
||||
self.processed_folder, self.training_file)) and os.path.exists(
|
||||
os.path.join(self.processed_folder, self.test_file))
|
||||
|
||||
def __repr__(self):
|
||||
head = "Dataset " + self.__class__.__name__
|
||||
body = ["Number of datapoints: {}".format(self.__len__())]
|
||||
if self.root is not None:
|
||||
body.append("Root location: {}".format(self.root))
|
||||
body += self.extra_repr().splitlines()
|
||||
lines = [head] + [" " * self._repr_indent + line for line in body]
|
||||
return "\n".join(lines)
|
||||
|
||||
def extra_repr(self):
|
||||
return f"Split: {'Train' if self.train is True else 'Test'}"
|
||||
|
||||
def __len__(self):
|
||||
return len(self.data)
|
||||
|
||||
def _download(self):
|
||||
raise NotImplementedError
|
||||
|
||||
|
||||
class NumpyDataset(torch.utils.data.TensorDataset):
|
||||
"""Create a PyTorch TensorDataset from NumPy arrays."""
|
||||
|
||||
def __init__(self, data, targets):
|
||||
self.data = torch.Tensor(data)
|
||||
self.targets = torch.LongTensor(targets)
|
||||
tensors = [self.data, self.targets]
|
||||
super().__init__(*tensors)
|
||||
|
||||
|
||||
class CSVDataset(NumpyDataset):
|
||||
"""Create a Dataset from a CSV file."""
|
||||
|
||||
def __init__(self, filepath, target_col=-1, delimiter=',', skip_header=0):
|
||||
raw = np.genfromtxt(
|
||||
filepath,
|
||||
delimiter=delimiter,
|
||||
skip_header=skip_header,
|
||||
)
|
||||
data = np.delete(raw, 1, target_col)
|
||||
targets = raw[:, target_col]
|
||||
super().__init__(data, targets)
|
@ -1,165 +0,0 @@
|
||||
"""Thin wrappers for a few scikit-learn datasets.
|
||||
|
||||
URL:
|
||||
https://scikit-learn.org/stable/modules/classes.html#module-sklearn.datasets
|
||||
|
||||
"""
|
||||
|
||||
from __future__ import annotations
|
||||
|
||||
import warnings
|
||||
from typing import Sequence
|
||||
|
||||
from sklearn.datasets import (
|
||||
load_iris,
|
||||
make_blobs,
|
||||
make_circles,
|
||||
make_classification,
|
||||
make_moons,
|
||||
)
|
||||
|
||||
from prototorch.datasets.abstract import NumpyDataset
|
||||
|
||||
|
||||
class Iris(NumpyDataset):
|
||||
"""Iris Dataset by Ronald Fisher introduced in 1936.
|
||||
|
||||
The dataset contains four measurements from flowers of three species of iris.
|
||||
|
||||
.. list-table:: Iris
|
||||
:header-rows: 1
|
||||
|
||||
* - dimensions
|
||||
- classes
|
||||
- training size
|
||||
- validation size
|
||||
- test size
|
||||
* - 4
|
||||
- 3
|
||||
- 150
|
||||
- 0
|
||||
- 0
|
||||
|
||||
:param dims: select a subset of dimensions
|
||||
"""
|
||||
|
||||
def __init__(self, dims: Sequence[int] | None = None):
|
||||
x, y = load_iris(return_X_y=True)
|
||||
if dims is not None:
|
||||
x = x[:, dims]
|
||||
super().__init__(x, y)
|
||||
|
||||
|
||||
class Blobs(NumpyDataset):
|
||||
"""Generate isotropic Gaussian blobs for clustering.
|
||||
|
||||
Read more at
|
||||
https://scikit-learn.org/stable/datasets/sample_generators.html#sample-generators.
|
||||
|
||||
"""
|
||||
|
||||
def __init__(
|
||||
self,
|
||||
num_samples: int = 300,
|
||||
num_features: int = 2,
|
||||
seed: None | int = 0,
|
||||
):
|
||||
x, y = make_blobs(
|
||||
num_samples,
|
||||
num_features,
|
||||
centers=None,
|
||||
random_state=seed,
|
||||
shuffle=False,
|
||||
)
|
||||
super().__init__(x, y)
|
||||
|
||||
|
||||
class Random(NumpyDataset):
|
||||
"""Generate a random n-class classification problem.
|
||||
|
||||
Read more at
|
||||
https://scikit-learn.org/stable/modules/generated/sklearn.datasets.make_classification.html.
|
||||
|
||||
Note: n_classes * n_clusters_per_class <= 2**n_informative must satisfy.
|
||||
"""
|
||||
|
||||
def __init__(
|
||||
self,
|
||||
num_samples: int = 300,
|
||||
num_features: int = 2,
|
||||
num_classes: int = 2,
|
||||
num_clusters: int = 2,
|
||||
num_informative: None | int = None,
|
||||
separation: float = 1.0,
|
||||
seed: None | int = 0,
|
||||
):
|
||||
if not num_informative:
|
||||
import math
|
||||
num_informative = math.ceil(math.log2(num_classes * num_clusters))
|
||||
if num_features < num_informative:
|
||||
warnings.warn("Generating more features than requested.")
|
||||
num_features = num_informative
|
||||
x, y = make_classification(
|
||||
num_samples,
|
||||
num_features,
|
||||
n_informative=num_informative,
|
||||
n_redundant=0,
|
||||
n_classes=num_classes,
|
||||
n_clusters_per_class=num_clusters,
|
||||
class_sep=separation,
|
||||
random_state=seed,
|
||||
shuffle=False,
|
||||
)
|
||||
super().__init__(x, y)
|
||||
|
||||
|
||||
class Circles(NumpyDataset):
|
||||
"""Make a large circle containing a smaller circle in 2D.
|
||||
|
||||
A simple toy dataset to visualize clustering and classification algorithms.
|
||||
|
||||
Read more at
|
||||
https://scikit-learn.org/stable/modules/generated/sklearn.datasets.make_circles.html
|
||||
|
||||
"""
|
||||
|
||||
def __init__(
|
||||
self,
|
||||
num_samples: int = 300,
|
||||
noise: float = 0.3,
|
||||
factor: float = 0.8,
|
||||
seed: None | int = 0,
|
||||
):
|
||||
x, y = make_circles(
|
||||
num_samples,
|
||||
noise=noise,
|
||||
factor=factor,
|
||||
random_state=seed,
|
||||
shuffle=False,
|
||||
)
|
||||
super().__init__(x, y)
|
||||
|
||||
|
||||
class Moons(NumpyDataset):
|
||||
"""Make two interleaving half circles.
|
||||
|
||||
A simple toy dataset to visualize clustering and classification algorithms.
|
||||
|
||||
Read more at
|
||||
https://scikit-learn.org/stable/modules/generated/sklearn.datasets.make_moons.html
|
||||
|
||||
"""
|
||||
|
||||
def __init__(
|
||||
self,
|
||||
num_samples: int = 300,
|
||||
noise: float = 0.3,
|
||||
seed: None | int = 0,
|
||||
):
|
||||
x, y = make_moons(
|
||||
num_samples,
|
||||
noise=noise,
|
||||
random_state=seed,
|
||||
shuffle=False,
|
||||
)
|
||||
super().__init__(x, y)
|
@ -1,59 +0,0 @@
|
||||
"""Spiral dataset for binary classification."""
|
||||
|
||||
import numpy as np
|
||||
import torch
|
||||
|
||||
|
||||
def make_spiral(num_samples=500, noise=0.3):
|
||||
"""Generates the Spiral Dataset.
|
||||
|
||||
For use in Prototorch use `prototorch.datasets.Spiral` instead.
|
||||
"""
|
||||
|
||||
def get_samples(n, delta_t):
|
||||
points = []
|
||||
for i in range(n):
|
||||
r = i / num_samples * 5
|
||||
t = 1.75 * i / n * 2 * np.pi + delta_t
|
||||
x = r * np.sin(t) + np.random.rand(1) * noise
|
||||
y = r * np.cos(t) + np.random.rand(1) * noise
|
||||
points.append([x, y])
|
||||
return points
|
||||
|
||||
n = num_samples // 2
|
||||
positive = get_samples(n=n, delta_t=0)
|
||||
negative = get_samples(n=n, delta_t=np.pi)
|
||||
x = np.concatenate(
|
||||
[np.array(positive).reshape(n, -1),
|
||||
np.array(negative).reshape(n, -1)],
|
||||
axis=0)
|
||||
y = np.concatenate([np.zeros(n), np.ones(n)])
|
||||
return x, y
|
||||
|
||||
|
||||
class Spiral(torch.utils.data.TensorDataset):
|
||||
"""Spiral dataset for binary classification.
|
||||
|
||||
This datasets consists of two spirals of two different classes.
|
||||
|
||||
.. list-table:: Spiral
|
||||
:header-rows: 1
|
||||
|
||||
* - dimensions
|
||||
- classes
|
||||
- training size
|
||||
- validation size
|
||||
- test size
|
||||
* - 2
|
||||
- 2
|
||||
- num_samples
|
||||
- 0
|
||||
- 0
|
||||
|
||||
:param num_samples: number of random samples
|
||||
:param noise: noise added to the spirals
|
||||
"""
|
||||
|
||||
def __init__(self, num_samples: int = 500, noise: float = 0.3):
|
||||
x, y = make_spiral(num_samples, noise)
|
||||
super().__init__(torch.Tensor(x), torch.LongTensor(y))
|
@ -1,118 +0,0 @@
|
||||
"""Tecator dataset for classification.
|
||||
|
||||
URL:
|
||||
http://lib.stat.cmu.edu/datasets/tecator
|
||||
|
||||
LICENCE / TERMS / COPYRIGHT:
|
||||
This is the Tecator data set: The task is to predict the fat content
|
||||
of a meat sample on the basis of its near infrared absorbance spectrum.
|
||||
-------------------------------------------------------------------------
|
||||
1. Statement of permission from Tecator (the original data source)
|
||||
|
||||
These data are recorded on a Tecator Infratec Food and Feed Analyzer
|
||||
working in the wavelength range 850 - 1050 nm by the Near Infrared
|
||||
Transmission (NIT) principle. Each sample contains finely chopped pure
|
||||
meat with different moisture, fat and protein contents.
|
||||
|
||||
If results from these data are used in a publication we want you to
|
||||
mention the instrument and company name (Tecator) in the publication.
|
||||
In addition, please send a preprint of your article to
|
||||
|
||||
Karin Thente, Tecator AB,
|
||||
Box 70, S-263 21 Hoganas, Sweden
|
||||
|
||||
The data are available in the public domain with no responsability from
|
||||
the original data source. The data can be redistributed as long as this
|
||||
permission note is attached.
|
||||
|
||||
For more information about the instrument - call Perstorp Analytical's
|
||||
representative in your area.
|
||||
|
||||
Description:
|
||||
For each meat sample the data consists of a 100 channel spectrum of
|
||||
absorbances and the contents of moisture (water), fat and protein.
|
||||
The absorbance is -log10 of the transmittance
|
||||
measured by the spectrometer. The three contents, measured in percent,
|
||||
are determined by analytic chemistry.
|
||||
"""
|
||||
|
||||
import logging
|
||||
import os
|
||||
|
||||
import numpy as np
|
||||
import torch
|
||||
from torchvision.datasets.utils import download_file_from_google_drive
|
||||
|
||||
from prototorch.datasets.abstract import ProtoDataset
|
||||
|
||||
|
||||
class Tecator(ProtoDataset):
|
||||
"""
|
||||
`Tecator Dataset <http://lib.stat.cmu.edu/datasets/tecator>`__ for classification.
|
||||
|
||||
The dataset contains wavelength measurements of meat.
|
||||
|
||||
.. list-table:: Tecator
|
||||
:header-rows: 1
|
||||
|
||||
* - dimensions
|
||||
- classes
|
||||
- training size
|
||||
- validation size
|
||||
- test size
|
||||
* - 100
|
||||
- 2
|
||||
- 129
|
||||
- 43
|
||||
- 43
|
||||
"""
|
||||
|
||||
_resources = [
|
||||
("1P9WIYnyxFPh6f1vqAbnKfK8oYmUgyV83",
|
||||
"ba5607c580d0f91bb27dc29d13c2f8df"),
|
||||
] # (google_storage_id, md5hash)
|
||||
classes = ["0 - low_fat", "1 - high_fat"]
|
||||
|
||||
def __getitem__(self, index):
|
||||
img, target = self.data[index], int(self.targets[index])
|
||||
return img, target
|
||||
|
||||
def _download(self):
|
||||
"""Download the data if it doesn't exist in already."""
|
||||
if self._check_exists():
|
||||
return
|
||||
|
||||
logging.debug("Making directories...")
|
||||
os.makedirs(self.raw_folder, exist_ok=True)
|
||||
os.makedirs(self.processed_folder, exist_ok=True)
|
||||
|
||||
logging.debug("Downloading...")
|
||||
for fileid, md5 in self._resources:
|
||||
filename = "tecator.npz"
|
||||
download_file_from_google_drive(fileid,
|
||||
root=self.raw_folder,
|
||||
filename=filename,
|
||||
md5=md5)
|
||||
|
||||
logging.debug("Processing...")
|
||||
with np.load(os.path.join(self.raw_folder, "tecator.npz"),
|
||||
allow_pickle=False) as f:
|
||||
x_train, y_train = f["x_train"], f["y_train"]
|
||||
x_test, y_test = f["x_test"], f["y_test"]
|
||||
training_set = [
|
||||
torch.Tensor(x_train),
|
||||
torch.LongTensor(y_train),
|
||||
]
|
||||
test_set = [
|
||||
torch.Tensor(x_test),
|
||||
torch.LongTensor(y_test),
|
||||
]
|
||||
|
||||
with open(os.path.join(self.processed_folder, self.training_file),
|
||||
"wb") as f:
|
||||
torch.save(training_set, f)
|
||||
with open(os.path.join(self.processed_folder, self.test_file),
|
||||
"wb") as f:
|
||||
torch.save(test_set, f)
|
||||
|
||||
logging.debug("Done!")
|
@ -1,19 +0,0 @@
|
||||
"""Exclusive-or (XOR) dataset for binary classification."""
|
||||
|
||||
import torch
|
||||
|
||||
|
||||
def make_xor(num_samples=500):
|
||||
x = torch.rand(num_samples, 2)
|
||||
y = torch.zeros(num_samples)
|
||||
y[torch.logical_and(x[:, 0] > 0.5, x[:, 1] < 0.5)] = 1
|
||||
y[torch.logical_and(x[:, 1] > 0.5, x[:, 0] < 0.5)] = 1
|
||||
return x, y
|
||||
|
||||
|
||||
class XOR(torch.utils.data.TensorDataset):
|
||||
"""Exclusive-or (XOR) dataset for binary classification."""
|
||||
|
||||
def __init__(self, num_samples: int = 500):
|
||||
x, y = make_xor(num_samples)
|
||||
super().__init__(x, y)
|
48
prototorch/functions/activations.py
Normal file
48
prototorch/functions/activations.py
Normal file
@ -0,0 +1,48 @@
|
||||
"""ProtoTorch activation functions."""
|
||||
|
||||
import torch
|
||||
|
||||
ACTIVATIONS = dict()
|
||||
|
||||
|
||||
def register_activation(func):
|
||||
ACTIVATIONS[func.__name__] = func
|
||||
return func
|
||||
|
||||
|
||||
@register_activation
|
||||
def identity(input, **kwargs):
|
||||
""":math:`f(x) = x`"""
|
||||
return input
|
||||
|
||||
|
||||
@register_activation
|
||||
def sigmoid_beta(input, beta=10):
|
||||
""":math:`f(x) = \\frac{1}{1 + e^{-\\beta x}}`
|
||||
|
||||
Keyword Arguments:
|
||||
beta (float): Parameter :math:`\\beta`
|
||||
"""
|
||||
out = torch.reciprocal(1.0 + torch.exp(-beta * input))
|
||||
return out
|
||||
|
||||
|
||||
@register_activation
|
||||
def swish_beta(input, beta=10):
|
||||
""":math:`f(x) = \\frac{x}{1 + e^{-\\beta x}}`
|
||||
|
||||
Keyword Arguments:
|
||||
beta (float): Parameter :math:`\\beta`
|
||||
"""
|
||||
out = input * sigmoid_beta(input, beta=beta)
|
||||
return out
|
||||
|
||||
|
||||
def get_activation(funcname):
|
||||
if callable(funcname):
|
||||
return funcname
|
||||
else:
|
||||
if funcname in ACTIVATIONS:
|
||||
return ACTIVATIONS.get(funcname)
|
||||
else:
|
||||
raise NameError(f'Activation {funcname} was not found.')
|
15
prototorch/functions/competitions.py
Normal file
15
prototorch/functions/competitions.py
Normal file
@ -0,0 +1,15 @@
|
||||
"""ProtoTorch competition functions."""
|
||||
|
||||
import torch
|
||||
|
||||
|
||||
def wtac(distances, labels):
|
||||
winning_indices = torch.min(distances, dim=1).indices
|
||||
winning_labels = labels[winning_indices].squeeze()
|
||||
return winning_labels
|
||||
|
||||
|
||||
def knnc(distances, labels, k):
|
||||
winning_indices = torch.topk(-distances, k=k, dim=1).indices
|
||||
winning_labels = labels[winning_indices].squeeze()
|
||||
return winning_labels
|
78
prototorch/functions/distances.py
Normal file
78
prototorch/functions/distances.py
Normal file
@ -0,0 +1,78 @@
|
||||
"""ProtoTorch distance functions."""
|
||||
|
||||
import torch
|
||||
|
||||
|
||||
def squared_euclidean_distance(x, y):
|
||||
"""Compute the squared Euclidean distance between :math:`x` and :math:`y`.
|
||||
|
||||
Expected dimension of x is 2.
|
||||
Expected dimension of y is 2.
|
||||
"""
|
||||
expanded_x = x.unsqueeze(dim=1)
|
||||
batchwise_difference = y - expanded_x
|
||||
differences_raised = torch.pow(batchwise_difference, 2)
|
||||
distances = torch.sum(differences_raised, axis=2)
|
||||
return distances
|
||||
|
||||
|
||||
def euclidean_distance(x, y):
|
||||
"""Compute the Euclidean distance between :math:`x` and :math:`y`.
|
||||
|
||||
Expected dimension of x is 2.
|
||||
Expected dimension of y is 2.
|
||||
"""
|
||||
distances_raised = squared_euclidean_distance(x, y)
|
||||
distances = torch.sqrt(distances_raised)
|
||||
return distances
|
||||
|
||||
|
||||
def lpnorm_distance(x, y, p):
|
||||
"""Compute :math:`{\\langle x, y \\rangle}_p`.
|
||||
|
||||
Expected dimension of x is 2.
|
||||
Expected dimension of y is 2.
|
||||
"""
|
||||
# # DEPRECATED in favor of torch.cdist
|
||||
# expanded_x = x.unsqueeze(dim=1)
|
||||
# batchwise_difference = y - expanded_x
|
||||
# differences_raised = torch.pow(batchwise_difference, p)
|
||||
# distances_raised = torch.sum(differences_raised, axis=2)
|
||||
# distances = torch.pow(distances_raised, 1.0 / p)
|
||||
# return distances
|
||||
distances = torch.cdist(x, y, p=p)
|
||||
return distances
|
||||
|
||||
|
||||
def omega_distance(x, y, omega):
|
||||
"""Omega distance.
|
||||
|
||||
Compute :math:`{\\langle \\Omega x, \\Omega y \\rangle}_p`
|
||||
|
||||
Expected dimension of x is 2.
|
||||
Expected dimension of y is 2.
|
||||
Expected dimension of omega is 2.
|
||||
"""
|
||||
projected_x = x @ omega
|
||||
projected_y = y @ omega
|
||||
distances = squared_euclidean_distance(projected_x, projected_y)
|
||||
return distances
|
||||
|
||||
|
||||
def lomega_distance(x, y, omegas):
|
||||
"""Localized Omega distance.
|
||||
|
||||
Compute :math:`{\\langle \\Omega_k x, \\Omega_k y_k \\rangle}_p`
|
||||
|
||||
Expected dimension of x is 2.
|
||||
Expected dimension of y is 2.
|
||||
Expected dimension of omegas is 3.
|
||||
"""
|
||||
projected_x = x @ omegas
|
||||
projected_y = torch.diagonal(y @ omegas).T
|
||||
expanded_y = torch.unsqueeze(projected_y, dim=1)
|
||||
batchwise_difference = expanded_y - projected_x
|
||||
differences_squared = batchwise_difference**2
|
||||
distances = torch.sum(differences_squared, dim=2)
|
||||
distances = distances.permute(1, 0)
|
||||
return distances
|
93
prototorch/functions/initializers.py
Normal file
93
prototorch/functions/initializers.py
Normal file
@ -0,0 +1,93 @@
|
||||
"""ProtoTorch initialization functions."""
|
||||
|
||||
from itertools import chain
|
||||
|
||||
import torch
|
||||
|
||||
INITIALIZERS = dict()
|
||||
|
||||
|
||||
def register_initializer(func):
|
||||
INITIALIZERS[func.__name__] = func
|
||||
return func
|
||||
|
||||
|
||||
def labels_from(distribution):
|
||||
"""Takes a distribution tensor and returns a labels tensor."""
|
||||
nclasses = distribution.shape[0]
|
||||
llist = [[i] * n for i, n in zip(range(nclasses), distribution)]
|
||||
# labels = [l for cl in llist for l in cl] # flatten the list of lists
|
||||
labels = list(chain(*llist)) # flatten using itertools.chain
|
||||
return torch.tensor(labels, requires_grad=False)
|
||||
|
||||
|
||||
@register_initializer
|
||||
def ones(x_train, y_train, prototype_distribution):
|
||||
nprotos = torch.sum(prototype_distribution)
|
||||
protos = torch.ones(nprotos, *x_train.shape[1:])
|
||||
plabels = labels_from(prototype_distribution)
|
||||
return protos, plabels
|
||||
|
||||
|
||||
@register_initializer
|
||||
def zeros(x_train, y_train, prototype_distribution):
|
||||
nprotos = torch.sum(prototype_distribution)
|
||||
protos = torch.zeros(nprotos, *x_train.shape[1:])
|
||||
plabels = labels_from(prototype_distribution)
|
||||
return protos, plabels
|
||||
|
||||
|
||||
@register_initializer
|
||||
def rand(x_train, y_train, prototype_distribution):
|
||||
nprotos = torch.sum(prototype_distribution)
|
||||
protos = torch.rand(nprotos, *x_train.shape[1:])
|
||||
plabels = labels_from(prototype_distribution)
|
||||
return protos, plabels
|
||||
|
||||
|
||||
@register_initializer
|
||||
def randn(x_train, y_train, prototype_distribution):
|
||||
nprotos = torch.sum(prototype_distribution)
|
||||
protos = torch.randn(nprotos, *x_train.shape[1:])
|
||||
plabels = labels_from(prototype_distribution)
|
||||
return protos, plabels
|
||||
|
||||
|
||||
@register_initializer
|
||||
def stratified_mean(x_train, y_train, prototype_distribution):
|
||||
nprotos = torch.sum(prototype_distribution)
|
||||
pdim = x_train.shape[1]
|
||||
protos = torch.empty(nprotos, pdim)
|
||||
plabels = labels_from(prototype_distribution)
|
||||
for i, l in enumerate(plabels):
|
||||
xl = x_train[y_train == l]
|
||||
mean_xl = torch.mean(xl, dim=0)
|
||||
protos[i] = mean_xl
|
||||
return protos, plabels
|
||||
|
||||
|
||||
@register_initializer
|
||||
def stratified_random(x_train, y_train, prototype_distribution):
|
||||
gen = torch.manual_seed(torch.initial_seed())
|
||||
nprotos = torch.sum(prototype_distribution)
|
||||
pdim = x_train.shape[1]
|
||||
protos = torch.empty(nprotos, pdim)
|
||||
plabels = labels_from(prototype_distribution)
|
||||
for i, l in enumerate(plabels):
|
||||
xl = x_train[y_train == l]
|
||||
rand_index = torch.zeros(1).long().random_(0,
|
||||
xl.shape[1] - 1,
|
||||
generator=gen)
|
||||
random_xl = xl[rand_index]
|
||||
protos[i] = random_xl
|
||||
return protos, plabels
|
||||
|
||||
|
||||
def get_initializer(funcname):
|
||||
if callable(funcname):
|
||||
return funcname
|
||||
else:
|
||||
if funcname in INITIALIZERS:
|
||||
return INITIALIZERS.get(funcname)
|
||||
else:
|
||||
raise NameError(f'Initializer {funcname} was not found.')
|
25
prototorch/functions/losses.py
Normal file
25
prototorch/functions/losses.py
Normal file
@ -0,0 +1,25 @@
|
||||
"""ProtoTorch loss functions."""
|
||||
|
||||
import torch
|
||||
|
||||
|
||||
def glvq_loss(distances, target_labels, prototype_labels):
|
||||
"""GLVQ loss function with support for one-hot labels."""
|
||||
matcher = torch.eq(target_labels.unsqueeze(dim=1), prototype_labels)
|
||||
if prototype_labels.ndim == 2:
|
||||
# if the labels are one-hot vectors
|
||||
nclasses = target_labels.size()[1]
|
||||
matcher = torch.eq(torch.sum(matcher, dim=-1), nclasses)
|
||||
not_matcher = torch.bitwise_not(matcher)
|
||||
|
||||
dplus_criterion = distances * matcher > 0.0
|
||||
dminus_criterion = distances * not_matcher > 0.0
|
||||
|
||||
inf = torch.full_like(distances, fill_value=float('inf'))
|
||||
distances_to_wpluses = torch.where(dplus_criterion, distances, inf)
|
||||
distances_to_wminuses = torch.where(dminus_criterion, distances, inf)
|
||||
dpluses = torch.min(distances_to_wpluses, dim=1, keepdim=True).values
|
||||
dminuses = torch.min(distances_to_wminuses, dim=1, keepdim=True).values
|
||||
|
||||
mu = (dpluses - dminuses) / (dpluses + dminuses)
|
||||
return mu
|
0
prototorch/modules/__init__.py
Normal file
0
prototorch/modules/__init__.py
Normal file
21
prototorch/modules/losses.py
Normal file
21
prototorch/modules/losses.py
Normal file
@ -0,0 +1,21 @@
|
||||
"""ProtoTorch losses."""
|
||||
|
||||
import torch
|
||||
|
||||
from prototorch.functions.activations import get_activation
|
||||
from prototorch.functions.losses import glvq_loss
|
||||
|
||||
|
||||
class GLVQLoss(torch.nn.Module):
|
||||
"""GLVQ Loss."""
|
||||
def __init__(self, margin=0.0, squashing='identity', beta=10, **kwargs):
|
||||
super().__init__(**kwargs)
|
||||
self.margin = margin
|
||||
self.squashing = get_activation(squashing)
|
||||
self.beta = beta
|
||||
|
||||
def forward(self, outputs, targets):
|
||||
distances, plabels = outputs
|
||||
mu = glvq_loss(distances, targets, plabels)
|
||||
batch_loss = self.squashing(mu + self.margin, beta=self.beta)
|
||||
return torch.sum(batch_loss, dim=0)
|
57
prototorch/modules/prototypes.py
Normal file
57
prototorch/modules/prototypes.py
Normal file
@ -0,0 +1,57 @@
|
||||
"""ProtoTorch prototype modules."""
|
||||
|
||||
import torch
|
||||
|
||||
from prototorch.functions.initializers import get_initializer
|
||||
|
||||
|
||||
class AddPrototypes1D(torch.nn.Module):
|
||||
def __init__(self,
|
||||
prototypes_per_class=1,
|
||||
prototype_distribution=None,
|
||||
prototype_initializer='ones',
|
||||
data=None,
|
||||
**kwargs):
|
||||
|
||||
if data is None:
|
||||
if 'input_dim' not in kwargs:
|
||||
raise NameError('`input_dim` required if '
|
||||
'no `data` is provided.')
|
||||
if prototype_distribution is not None:
|
||||
nclasses = sum(prototype_distribution)
|
||||
else:
|
||||
if 'nclasses' not in kwargs:
|
||||
raise NameError('`prototype_distribution` required if '
|
||||
'both `data` and `nclasses` are not '
|
||||
'provided.')
|
||||
nclasses = kwargs.pop('nclasses')
|
||||
input_dim = kwargs.pop('input_dim')
|
||||
# input_shape = (input_dim, )
|
||||
x_train = torch.rand(nclasses, input_dim)
|
||||
y_train = torch.arange(nclasses)
|
||||
|
||||
else:
|
||||
x_train, y_train = data
|
||||
x_train = torch.as_tensor(x_train)
|
||||
y_train = torch.as_tensor(y_train)
|
||||
|
||||
super().__init__(**kwargs)
|
||||
self.prototypes_per_class = prototypes_per_class
|
||||
with torch.no_grad():
|
||||
if not prototype_distribution:
|
||||
num_classes = torch.unique(y_train).shape[0]
|
||||
self.prototype_distribution = torch.tensor(
|
||||
[self.prototypes_per_class] * num_classes)
|
||||
else:
|
||||
self.prototype_distribution = torch.tensor(
|
||||
prototype_distribution)
|
||||
self.prototype_initializer = get_initializer(prototype_initializer)
|
||||
prototypes, prototype_labels = self.prototype_initializer(
|
||||
x_train,
|
||||
y_train,
|
||||
prototype_distribution=self.prototype_distribution)
|
||||
self.prototypes = torch.nn.Parameter(prototypes)
|
||||
self.prototype_labels = prototype_labels
|
||||
|
||||
def forward(self):
|
||||
return self.prototypes, self.prototype_labels
|
@ -1,4 +0,0 @@
|
||||
"""ProtoTorch Neural Network Module"""
|
||||
|
||||
from .activations import *
|
||||
from .wrappers import *
|
@ -1,66 +0,0 @@
|
||||
"""ProtoTorch activations"""
|
||||
|
||||
import torch
|
||||
|
||||
ACTIVATIONS = dict()
|
||||
|
||||
|
||||
def register_activation(fn):
|
||||
"""Add the activation function to the registry."""
|
||||
name = fn.__name__
|
||||
ACTIVATIONS[name] = fn
|
||||
return fn
|
||||
|
||||
|
||||
@register_activation
|
||||
def identity(x, beta=0.0):
|
||||
"""Identity activation function.
|
||||
|
||||
Definition:
|
||||
:math:`f(x) = x`
|
||||
|
||||
Keyword Arguments:
|
||||
beta (`float`): Ignored.
|
||||
"""
|
||||
return x
|
||||
|
||||
|
||||
@register_activation
|
||||
def sigmoid_beta(x, beta=10.0):
|
||||
r"""Sigmoid activation function with scaling.
|
||||
|
||||
Definition:
|
||||
:math:`f(x) = \frac{1}{1 + e^{-\beta x}}`
|
||||
|
||||
Keyword Arguments:
|
||||
beta (`float`): Scaling parameter :math:`\beta`
|
||||
"""
|
||||
out = 1.0 / (1.0 + torch.exp(-1.0 * beta * x))
|
||||
return out
|
||||
|
||||
|
||||
@register_activation
|
||||
def swish_beta(x, beta=10.0):
|
||||
r"""Swish activation function with scaling.
|
||||
|
||||
Definition:
|
||||
:math:`f(x) = \frac{x}{1 + e^{-\beta x}}`
|
||||
|
||||
Keyword Arguments:
|
||||
beta (`float`): Scaling parameter :math:`\beta`
|
||||
"""
|
||||
out = x * sigmoid_beta(x, beta=beta)
|
||||
return out
|
||||
|
||||
|
||||
def get_activation(funcname):
|
||||
"""Deserialize the activation function."""
|
||||
if callable(funcname):
|
||||
return funcname
|
||||
elif funcname in ACTIVATIONS:
|
||||
return ACTIVATIONS.get(funcname)
|
||||
else:
|
||||
emsg = f"Unable to find matching function for `{funcname}` " \
|
||||
f"in `prototorch.nn.activations`. "
|
||||
helpmsg = f"Possible values are {list(ACTIVATIONS.keys())}."
|
||||
raise NameError(emsg + helpmsg)
|
@ -1,38 +0,0 @@
|
||||
"""ProtoTorch wrappers."""
|
||||
|
||||
import torch
|
||||
|
||||
|
||||
class LambdaLayer(torch.nn.Module):
|
||||
|
||||
def __init__(self, fn, name=None):
|
||||
super().__init__()
|
||||
self.fn = fn
|
||||
self.name = name or fn.__name__ # lambda fns get <lambda>
|
||||
|
||||
def forward(self, *args, **kwargs):
|
||||
return self.fn(*args, **kwargs)
|
||||
|
||||
def extra_repr(self):
|
||||
return self.name
|
||||
|
||||
|
||||
class LossLayer(torch.nn.modules.loss._Loss):
|
||||
|
||||
def __init__(self,
|
||||
fn,
|
||||
name=None,
|
||||
size_average=None,
|
||||
reduce=None,
|
||||
reduction: str = "mean") -> None:
|
||||
super().__init__(size_average=size_average,
|
||||
reduce=reduce,
|
||||
reduction=reduction)
|
||||
self.fn = fn
|
||||
self.name = name or fn.__name__ # lambda fns get <lambda>
|
||||
|
||||
def forward(self, *args, **kwargs):
|
||||
return self.fn(*args, **kwargs)
|
||||
|
||||
def extra_repr(self):
|
||||
return self.name
|
@ -1,13 +0,0 @@
|
||||
"""ProtoTorch utils module"""
|
||||
|
||||
from .colors import (
|
||||
get_colors,
|
||||
get_legend_handles,
|
||||
hex_to_rgb,
|
||||
rgb_to_hex,
|
||||
)
|
||||
from .utils import (
|
||||
mesh2d,
|
||||
parse_data_arg,
|
||||
parse_distribution,
|
||||
)
|
@ -1,60 +0,0 @@
|
||||
"""ProtoTorch color utilities"""
|
||||
|
||||
import matplotlib.lines as mlines
|
||||
import torch
|
||||
from matplotlib import cm
|
||||
from matplotlib.colors import (
|
||||
Normalize,
|
||||
to_hex,
|
||||
to_rgb,
|
||||
)
|
||||
|
||||
|
||||
def hex_to_rgb(hex_values):
|
||||
for v in hex_values:
|
||||
v = v.lstrip('#')
|
||||
lv = len(v)
|
||||
c = [int(v[i:i + lv // 3], 16) for i in range(0, lv, lv // 3)]
|
||||
yield c
|
||||
|
||||
|
||||
def rgb_to_hex(rgb_values):
|
||||
for v in rgb_values:
|
||||
c = "%02x%02x%02x" % tuple(v)
|
||||
yield c
|
||||
|
||||
|
||||
def get_colors(vmax, vmin=0, cmap="viridis"):
|
||||
cmap = cm.get_cmap(cmap)
|
||||
colornorm = Normalize(vmin=vmin, vmax=vmax)
|
||||
colors = dict()
|
||||
for c in range(vmin, vmax + 1):
|
||||
colors[c] = to_hex(cmap(colornorm(c)))
|
||||
return colors
|
||||
|
||||
|
||||
def get_legend_handles(colors, labels, marker="dots", zero_indexed=False):
|
||||
handles = list()
|
||||
for color, label in zip(colors.values(), labels):
|
||||
if marker == "dots":
|
||||
handle = mlines.Line2D(
|
||||
xdata=[],
|
||||
ydata=[],
|
||||
label=label,
|
||||
color="white",
|
||||
markerfacecolor=color,
|
||||
marker="o",
|
||||
markersize=10,
|
||||
markeredgecolor="k",
|
||||
)
|
||||
else:
|
||||
handle = mlines.Line2D(
|
||||
xdata=[],
|
||||
ydata=[],
|
||||
label=label,
|
||||
color=color,
|
||||
marker="",
|
||||
markersize=15,
|
||||
)
|
||||
handles.append(handle)
|
||||
return handles
|
@ -1,136 +0,0 @@
|
||||
"""ProtoTorch utilities"""
|
||||
|
||||
import warnings
|
||||
from typing import (
|
||||
Dict,
|
||||
Iterable,
|
||||
List,
|
||||
Optional,
|
||||
Union,
|
||||
)
|
||||
|
||||
import numpy as np
|
||||
import torch
|
||||
from torch.utils.data import DataLoader, Dataset
|
||||
|
||||
|
||||
def generate_mesh(
|
||||
minima: torch.TensorType,
|
||||
maxima: torch.TensorType,
|
||||
border: float = 1.0,
|
||||
resolution: int = 100,
|
||||
device: Optional[torch.device] = None,
|
||||
):
|
||||
# Apply Border
|
||||
ptp = maxima - minima
|
||||
shift = border * ptp
|
||||
minima -= shift
|
||||
maxima += shift
|
||||
|
||||
# Generate Mesh
|
||||
minima = minima.to(device).unsqueeze(1)
|
||||
maxima = maxima.to(device).unsqueeze(1)
|
||||
|
||||
factors = torch.linspace(0, 1, resolution, device=device)
|
||||
marginals = factors * maxima + ((1 - factors) * minima)
|
||||
|
||||
single_dimensions = torch.meshgrid(*marginals)
|
||||
mesh_input = torch.stack([dim.ravel() for dim in single_dimensions], dim=1)
|
||||
|
||||
return mesh_input, single_dimensions
|
||||
|
||||
|
||||
def mesh2d(x=None, border: float = 1.0, resolution: int = 100):
|
||||
if x is not None:
|
||||
x_shift = border * np.ptp(x[:, 0])
|
||||
y_shift = border * np.ptp(x[:, 1])
|
||||
x_min, x_max = x[:, 0].min() - x_shift, x[:, 0].max() + x_shift
|
||||
y_min, y_max = x[:, 1].min() - y_shift, x[:, 1].max() + y_shift
|
||||
else:
|
||||
x_min, x_max = -border, border
|
||||
y_min, y_max = -border, border
|
||||
xx, yy = np.meshgrid(np.linspace(x_min, x_max, resolution),
|
||||
np.linspace(y_min, y_max, resolution))
|
||||
mesh = np.c_[xx.ravel(), yy.ravel()]
|
||||
return mesh, xx, yy
|
||||
|
||||
|
||||
def distribution_from_list(list_dist: List[int],
|
||||
clabels: Optional[Iterable[int]] = None):
|
||||
clabels = clabels or list(range(len(list_dist)))
|
||||
distribution = dict(zip(clabels, list_dist))
|
||||
return distribution
|
||||
|
||||
|
||||
def parse_distribution(
|
||||
user_distribution,
|
||||
clabels: Optional[Iterable[int]] = None) -> Dict[int, int]:
|
||||
"""Parse user-provided distribution.
|
||||
|
||||
Return a dictionary with integer keys that represent the class labels and
|
||||
values that denote the number of components/prototypes with that class
|
||||
label.
|
||||
|
||||
The argument `user_distribution` could be any one of a number of allowed
|
||||
formats. If it is a Python list, it is assumed that there are as many
|
||||
entries in this list as there are classes, and the value at each index of
|
||||
this list describes the number of prototypes for that particular class. So,
|
||||
[1, 1, 1] implies that we have three classes with one prototype per class.
|
||||
If it is a Python tuple, a shorthand of (num_classes, prototypes_per_class)
|
||||
is assumed. If it is a Python dictionary, the key-value pairs describe the
|
||||
class label and the number of prototypes for that class respectively. So,
|
||||
{0: 2, 1: 2, 2: 2} implies that we have three classes with labels {1, 2,
|
||||
3}, each equipped with two prototypes. If however, the dictionary contains
|
||||
the keys "num_classes" and "per_class", they are parsed to use their values
|
||||
as one might expect.
|
||||
|
||||
"""
|
||||
if isinstance(user_distribution, dict):
|
||||
if "num_classes" in user_distribution.keys():
|
||||
num_classes = int(user_distribution["num_classes"])
|
||||
per_class = int(user_distribution["per_class"])
|
||||
return distribution_from_list([per_class] * num_classes, clabels)
|
||||
else:
|
||||
return user_distribution
|
||||
elif isinstance(user_distribution, tuple):
|
||||
assert len(user_distribution) == 2
|
||||
num_classes, per_class = user_distribution
|
||||
num_classes, per_class = int(num_classes), int(per_class)
|
||||
return distribution_from_list([per_class] * num_classes, clabels)
|
||||
elif isinstance(user_distribution, list):
|
||||
return distribution_from_list(user_distribution, clabels)
|
||||
else:
|
||||
msg = f"`distribution` was not understood." \
|
||||
f"You have provided: {user_distribution}."
|
||||
raise ValueError(msg)
|
||||
|
||||
|
||||
def parse_data_arg(data_arg: Union[Dataset, DataLoader, list, tuple]):
|
||||
"""Return data and target as torch tensors."""
|
||||
if isinstance(data_arg, Dataset):
|
||||
if hasattr(data_arg, "__len__"):
|
||||
ds_size = len(data_arg) # type: ignore
|
||||
loader = DataLoader(data_arg, batch_size=ds_size)
|
||||
data, targets = next(iter(loader))
|
||||
else:
|
||||
emsg = f"Dataset {data_arg} is not sized (`__len__` unimplemented)."
|
||||
raise TypeError(emsg)
|
||||
|
||||
elif isinstance(data_arg, DataLoader):
|
||||
data = torch.tensor([])
|
||||
targets = torch.tensor([])
|
||||
for x, y in data_arg:
|
||||
data = torch.cat([data, x])
|
||||
targets = torch.cat([targets, y])
|
||||
else:
|
||||
assert len(data_arg) == 2
|
||||
data, targets = data_arg
|
||||
if not isinstance(data, torch.Tensor):
|
||||
wmsg = f"Converting data to {torch.Tensor}..."
|
||||
warnings.warn(wmsg)
|
||||
data = torch.Tensor(data)
|
||||
if not isinstance(targets, torch.LongTensor):
|
||||
wmsg = f"Converting targets to {torch.LongTensor}..."
|
||||
warnings.warn(wmsg)
|
||||
targets = torch.LongTensor(targets)
|
||||
return data, targets
|
16
setup.cfg
16
setup.cfg
@ -1,16 +0,0 @@
|
||||
[pylint]
|
||||
disable =
|
||||
too-many-arguments,
|
||||
too-few-public-methods,
|
||||
fixme,
|
||||
|
||||
|
||||
[pycodestyle]
|
||||
max-line-length = 79
|
||||
|
||||
[isort]
|
||||
multi_line_output = 3
|
||||
include_trailing_comma = True
|
||||
force_grid_wrap = 3
|
||||
use_parentheses = True
|
||||
line_length = 79
|
120
setup.py
120
setup.py
@ -1,95 +1,49 @@
|
||||
"""
|
||||
"""Install ProtoTorch."""
|
||||
|
||||
######
|
||||
# # ##### #### ##### #### ##### #### ##### #### # #
|
||||
# # # # # # # # # # # # # # # # # #
|
||||
###### # # # # # # # # # # # # # ######
|
||||
# ##### # # # # # # # # ##### # # #
|
||||
# # # # # # # # # # # # # # # # #
|
||||
# # # #### # #### # #### # # #### # #
|
||||
from setuptools import setup
|
||||
from setuptools import find_packages
|
||||
|
||||
ProtoTorch Core Package
|
||||
"""
|
||||
from setuptools import find_packages, setup
|
||||
PROJECT_URL = 'https://github.com/si-cim/prototorch'
|
||||
DOWNLOAD_URL = 'https://github.com/si-cim/prototorch.git'
|
||||
|
||||
PROJECT_URL = "https://github.com/si-cim/prototorch"
|
||||
DOWNLOAD_URL = "https://github.com/si-cim/prototorch.git"
|
||||
|
||||
with open("README.md", encoding="utf-8") as fh:
|
||||
with open('README.md', 'r') as fh:
|
||||
long_description = fh.read()
|
||||
|
||||
INSTALL_REQUIRES = [
|
||||
"torch>=2.0.0",
|
||||
"torchvision",
|
||||
"numpy",
|
||||
"scikit-learn",
|
||||
"matplotlib",
|
||||
]
|
||||
DATASETS = [
|
||||
"requests",
|
||||
"tqdm",
|
||||
]
|
||||
DEV = [
|
||||
"bump2version",
|
||||
"pre-commit",
|
||||
]
|
||||
DOCS = [
|
||||
"recommonmark",
|
||||
"sphinx",
|
||||
"sphinx_rtd_theme",
|
||||
"sphinxcontrib-katex",
|
||||
"sphinx-autodoc-typehints",
|
||||
]
|
||||
EXAMPLES = [
|
||||
"torchinfo",
|
||||
]
|
||||
TESTS = [
|
||||
"flake8",
|
||||
"pytest",
|
||||
]
|
||||
ALL = DATASETS + DEV + DOCS + EXAMPLES + TESTS
|
||||
|
||||
setup(
|
||||
name="prototorch",
|
||||
version="0.7.6",
|
||||
description="Highly extensible, GPU-supported "
|
||||
"Learning Vector Quantization (LVQ) toolbox "
|
||||
"built using PyTorch and its nn API.",
|
||||
setup(name='prototorch',
|
||||
version='0.1.0-dev0',
|
||||
description='Highly extensible, GPU-supported '
|
||||
'Learning Vector Quantization (LVQ) toolbox '
|
||||
'built using PyTorch and its nn API.',
|
||||
long_description=long_description,
|
||||
long_description_content_type="text/markdown",
|
||||
author="Jensun Ravichandran",
|
||||
author_email="jjensun@gmail.com",
|
||||
long_description_content_type='text/markdown',
|
||||
author='Jensun Ravichandran',
|
||||
author_email='jjensun@gmail.com',
|
||||
url=PROJECT_URL,
|
||||
download_url=DOWNLOAD_URL,
|
||||
license="MIT",
|
||||
python_requires=">=3.8",
|
||||
install_requires=INSTALL_REQUIRES,
|
||||
license='MIT',
|
||||
install_requires=[
|
||||
'torch>=1.3.1',
|
||||
'torchvision>=0.5.0',
|
||||
'numpy>=1.9.1',
|
||||
],
|
||||
extras_require={
|
||||
"datasets": DATASETS,
|
||||
"dev": DEV,
|
||||
"docs": DOCS,
|
||||
"examples": EXAMPLES,
|
||||
"tests": TESTS,
|
||||
"all": ALL,
|
||||
'examples': [
|
||||
'sklearn',
|
||||
'matplotlib',
|
||||
],
|
||||
'tests': ['pytest'],
|
||||
},
|
||||
classifiers=[
|
||||
"Environment :: Console",
|
||||
"Natural Language :: English",
|
||||
"Development Status :: 4 - Beta",
|
||||
"Intended Audience :: Developers",
|
||||
"Intended Audience :: Education",
|
||||
"Intended Audience :: Science/Research",
|
||||
"Topic :: Scientific/Engineering :: Artificial Intelligence",
|
||||
"Topic :: Software Development :: Libraries",
|
||||
"Topic :: Software Development :: Libraries :: Python Modules",
|
||||
"License :: OSI Approved :: MIT License",
|
||||
"Operating System :: OS Independent",
|
||||
"Programming Language :: Python :: 3",
|
||||
"Programming Language :: Python :: 3.8",
|
||||
"Programming Language :: Python :: 3.9",
|
||||
"Programming Language :: Python :: 3.10",
|
||||
"Programming Language :: Python :: 3.11",
|
||||
'Development Status :: 2 - Pre-Alpha', 'Environment :: Console',
|
||||
'Intended Audience :: Developers', 'Intended Audience :: Education',
|
||||
'Intended Audience :: Science/Research',
|
||||
'License :: OSI Approved :: MIT License',
|
||||
'Programming Language :: Python :: 3.6',
|
||||
'Programming Language :: Python :: 3.7',
|
||||
'Programming Language :: Python :: 3.8',
|
||||
'Operating System :: OS Independent',
|
||||
'Topic :: Scientific/Engineering :: Artificial Intelligence',
|
||||
'Topic :: Software Development :: Libraries',
|
||||
'Topic :: Software Development :: Libraries :: Python Modules'
|
||||
],
|
||||
packages=find_packages(),
|
||||
zip_safe=False,
|
||||
)
|
||||
packages=find_packages())
|
||||
|
@ -1,777 +0,0 @@
|
||||
"""ProtoTorch core test suite"""
|
||||
|
||||
import unittest
|
||||
|
||||
import numpy as np
|
||||
import pytest
|
||||
import torch
|
||||
|
||||
import prototorch as pt
|
||||
from prototorch.utils import parse_distribution
|
||||
|
||||
|
||||
# Utils
|
||||
def test_parse_distribution_dict_0():
|
||||
distribution = {"num_classes": 1, "per_class": 0}
|
||||
distribution = parse_distribution(distribution)
|
||||
assert distribution == {0: 0}
|
||||
|
||||
|
||||
def test_parse_distribution_dict_1():
|
||||
distribution = dict(num_classes=3, per_class=2)
|
||||
distribution = parse_distribution(distribution)
|
||||
assert distribution == {0: 2, 1: 2, 2: 2}
|
||||
|
||||
|
||||
def test_parse_distribution_dict_2():
|
||||
distribution = {0: 1, 2: 2, -1: 3}
|
||||
distribution = parse_distribution(distribution)
|
||||
assert distribution == {0: 1, 2: 2, -1: 3}
|
||||
|
||||
|
||||
def test_parse_distribution_tuple():
|
||||
distribution = (2, 3)
|
||||
distribution = parse_distribution(distribution)
|
||||
assert distribution == {0: 3, 1: 3}
|
||||
|
||||
|
||||
def test_parse_distribution_list():
|
||||
distribution = [1, 1, 0, 2]
|
||||
distribution = parse_distribution(distribution)
|
||||
assert distribution == {0: 1, 1: 1, 2: 0, 3: 2}
|
||||
|
||||
|
||||
def test_parse_distribution_custom_labels():
|
||||
distribution = [1, 1, 0, 2]
|
||||
clabels = [1, 2, 5, 3]
|
||||
distribution = parse_distribution(distribution, clabels)
|
||||
assert distribution == {1: 1, 2: 1, 5: 0, 3: 2}
|
||||
|
||||
|
||||
# Components initializers
|
||||
def test_literal_comp_generate():
|
||||
protos = torch.rand(4, 3, 5, 5)
|
||||
c = pt.initializers.LiteralCompInitializer(protos)
|
||||
components = c.generate([])
|
||||
assert torch.allclose(components, protos)
|
||||
|
||||
|
||||
def test_literal_comp_generate_from_list():
|
||||
protos = [[0, 1], [2, 3], [4, 5]]
|
||||
c = pt.initializers.LiteralCompInitializer(protos)
|
||||
with pytest.warns(UserWarning):
|
||||
components = c.generate([])
|
||||
assert torch.allclose(components, torch.Tensor(protos))
|
||||
|
||||
|
||||
def test_shape_aware_raises_error():
|
||||
with pytest.raises(TypeError):
|
||||
_ = pt.initializers.ShapeAwareCompInitializer(shape=(2, ))
|
||||
|
||||
|
||||
def test_data_aware_comp_generate():
|
||||
protos = torch.rand(4, 3, 5, 5)
|
||||
c = pt.initializers.DataAwareCompInitializer(protos)
|
||||
components = c.generate(num_components="IgnoreMe!")
|
||||
assert torch.allclose(components, protos)
|
||||
|
||||
|
||||
def test_class_aware_comp_generate():
|
||||
protos = torch.rand(4, 2, 3, 5, 5)
|
||||
plabels = torch.tensor([0, 0, 1, 1]).long()
|
||||
c = pt.initializers.ClassAwareCompInitializer([protos, plabels])
|
||||
components = c.generate(distribution=[])
|
||||
assert torch.allclose(components, protos)
|
||||
|
||||
|
||||
def test_zeros_comp_generate():
|
||||
shape = (3, 5, 5)
|
||||
c = pt.initializers.ZerosCompInitializer(shape)
|
||||
components = c.generate(num_components=4)
|
||||
assert torch.allclose(components, torch.zeros(4, 3, 5, 5))
|
||||
|
||||
|
||||
def test_ones_comp_generate():
|
||||
c = pt.initializers.OnesCompInitializer(2)
|
||||
components = c.generate(num_components=3)
|
||||
assert torch.allclose(components, torch.ones(3, 2))
|
||||
|
||||
|
||||
def test_fill_value_comp_generate():
|
||||
c = pt.initializers.FillValueCompInitializer(2, 0.0)
|
||||
components = c.generate(num_components=3)
|
||||
assert torch.allclose(components, torch.zeros(3, 2))
|
||||
|
||||
|
||||
def test_uniform_comp_generate_min_max_bound():
|
||||
c = pt.initializers.UniformCompInitializer(2, -1.0, 1.0)
|
||||
components = c.generate(num_components=1024)
|
||||
assert components.min() >= -1.0
|
||||
assert components.max() <= 1.0
|
||||
|
||||
|
||||
def test_random_comp_generate_mean():
|
||||
c = pt.initializers.RandomNormalCompInitializer(2, -1.0)
|
||||
components = c.generate(num_components=1024)
|
||||
assert torch.allclose(components.mean(),
|
||||
torch.tensor(-1.0),
|
||||
rtol=1e-05,
|
||||
atol=1e-01)
|
||||
|
||||
|
||||
def test_comp_generate_0_components():
|
||||
c = pt.initializers.ZerosCompInitializer(2)
|
||||
_ = c.generate(num_components=0)
|
||||
|
||||
|
||||
def test_stratified_mean_comp_generate():
|
||||
# yapf: disable
|
||||
x = torch.Tensor(
|
||||
[[0, -1, -2],
|
||||
[10, 11, 12],
|
||||
[0, 0, 0],
|
||||
[2, 2, 2]])
|
||||
y = torch.LongTensor([0, 0, 1, 1])
|
||||
desired = torch.Tensor(
|
||||
[[5.0, 5.0, 5.0],
|
||||
[1.0, 1.0, 1.0]])
|
||||
# yapf: enable
|
||||
c = pt.initializers.StratifiedMeanCompInitializer(data=[x, y])
|
||||
actual = c.generate([1, 1])
|
||||
assert torch.allclose(actual, desired)
|
||||
|
||||
|
||||
def test_stratified_selection_comp_generate():
|
||||
# yapf: disable
|
||||
x = torch.Tensor(
|
||||
[[0, 0, 0],
|
||||
[1, 1, 1],
|
||||
[0, 0, 0],
|
||||
[1, 1, 1]])
|
||||
y = torch.LongTensor([0, 1, 0, 1])
|
||||
desired = torch.Tensor(
|
||||
[[0, 0, 0],
|
||||
[1, 1, 1]])
|
||||
# yapf: enable
|
||||
c = pt.initializers.StratifiedSelectionCompInitializer(data=[x, y])
|
||||
actual = c.generate([1, 1])
|
||||
assert torch.allclose(actual, desired)
|
||||
|
||||
|
||||
# Labels initializers
|
||||
def test_literal_labels_init():
|
||||
l = pt.initializers.LiteralLabelsInitializer([0, 0, 1, 2])
|
||||
with pytest.warns(UserWarning):
|
||||
labels = l.generate([])
|
||||
assert torch.allclose(labels, torch.LongTensor([0, 0, 1, 2]))
|
||||
|
||||
|
||||
def test_labels_init_from_list():
|
||||
l = pt.initializers.LabelsInitializer()
|
||||
components = l.generate(distribution=[1, 1, 1])
|
||||
assert torch.allclose(components, torch.LongTensor([0, 1, 2]))
|
||||
|
||||
|
||||
def test_labels_init_from_tuple_legal():
|
||||
l = pt.initializers.LabelsInitializer()
|
||||
components = l.generate(distribution=(3, 1))
|
||||
assert torch.allclose(components, torch.LongTensor([0, 1, 2]))
|
||||
|
||||
|
||||
def test_labels_init_from_tuple_illegal():
|
||||
l = pt.initializers.LabelsInitializer()
|
||||
with pytest.raises(AssertionError):
|
||||
_ = l.generate(distribution=(1, 1, 1))
|
||||
|
||||
|
||||
def test_data_aware_labels_init():
|
||||
data, targets = [0, 1, 2, 3], [0, 0, 1, 1]
|
||||
ds = pt.datasets.NumpyDataset(data, targets)
|
||||
l = pt.initializers.DataAwareLabelsInitializer(ds)
|
||||
labels = l.generate([])
|
||||
assert torch.allclose(labels, torch.LongTensor(targets))
|
||||
|
||||
|
||||
# Reasonings initializers
|
||||
def test_literal_reasonings_init():
|
||||
r = pt.initializers.LiteralReasoningsInitializer([0, 0, 1, 2])
|
||||
with pytest.warns(UserWarning):
|
||||
reasonings = r.generate([])
|
||||
assert torch.allclose(reasonings, torch.Tensor([0, 0, 1, 2]))
|
||||
|
||||
|
||||
def test_random_reasonings_init():
|
||||
r = pt.initializers.RandomReasoningsInitializer(0.2, 0.8)
|
||||
reasonings = r.generate(distribution=[0, 1])
|
||||
assert torch.numel(reasonings) == 1 * 2 * 2
|
||||
assert reasonings.min() >= 0.2
|
||||
assert reasonings.max() <= 0.8
|
||||
|
||||
|
||||
def test_zeros_reasonings_init():
|
||||
r = pt.initializers.ZerosReasoningsInitializer()
|
||||
reasonings = r.generate(distribution=[0, 1])
|
||||
assert torch.allclose(reasonings, torch.zeros(1, 2, 2))
|
||||
|
||||
|
||||
def test_ones_reasonings_init():
|
||||
r = pt.initializers.ZerosReasoningsInitializer()
|
||||
reasonings = r.generate(distribution=[1, 2, 3])
|
||||
assert torch.allclose(reasonings, torch.zeros(6, 3, 2))
|
||||
|
||||
|
||||
def test_pure_positive_reasonings_init_one_per_class():
|
||||
r = pt.initializers.PurePositiveReasoningsInitializer(
|
||||
components_first=False)
|
||||
reasonings = r.generate(distribution=(4, 1))
|
||||
assert torch.allclose(reasonings[0], torch.eye(4))
|
||||
|
||||
|
||||
def test_pure_positive_reasonings_init_unrepresented_classes():
|
||||
r = pt.initializers.PurePositiveReasoningsInitializer()
|
||||
reasonings = r.generate(distribution=[9, 0, 0, 0])
|
||||
assert reasonings.shape[0] == 9
|
||||
assert reasonings.shape[1] == 4
|
||||
assert reasonings.shape[2] == 2
|
||||
|
||||
|
||||
def test_random_reasonings_init_channels_not_first():
|
||||
r = pt.initializers.RandomReasoningsInitializer(components_first=False)
|
||||
reasonings = r.generate(distribution=[0, 0, 0, 1])
|
||||
assert reasonings.shape[0] == 2
|
||||
assert reasonings.shape[1] == 4
|
||||
assert reasonings.shape[2] == 1
|
||||
|
||||
|
||||
# Transform initializers
|
||||
def test_eye_transform_init_square():
|
||||
t = pt.initializers.EyeLinearTransformInitializer()
|
||||
I = t.generate(3, 3)
|
||||
assert torch.allclose(I, torch.eye(3))
|
||||
|
||||
|
||||
def test_eye_transform_init_narrow():
|
||||
t = pt.initializers.EyeLinearTransformInitializer()
|
||||
actual = t.generate(3, 2)
|
||||
desired = torch.Tensor([[1, 0], [0, 1], [0, 0]])
|
||||
assert torch.allclose(actual, desired)
|
||||
|
||||
|
||||
def test_eye_transform_init_wide():
|
||||
t = pt.initializers.EyeLinearTransformInitializer()
|
||||
actual = t.generate(2, 3)
|
||||
desired = torch.Tensor([[1, 0, 0], [0, 1, 0]])
|
||||
assert torch.allclose(actual, desired)
|
||||
|
||||
|
||||
# Transforms
|
||||
def test_linear_transform_default_eye_init():
|
||||
l = pt.transforms.LinearTransform(2, 4)
|
||||
actual = l.weights
|
||||
desired = torch.Tensor([[1, 0, 0, 0], [0, 1, 0, 0]])
|
||||
assert torch.allclose(actual, desired)
|
||||
|
||||
|
||||
def test_linear_transform_forward():
|
||||
l = pt.transforms.LinearTransform(4, 2)
|
||||
actual_weights = l.weights
|
||||
desired_weights = torch.Tensor([[1, 0], [0, 1], [0, 0], [0, 0]])
|
||||
assert torch.allclose(actual_weights, desired_weights)
|
||||
actual_outputs = l(torch.Tensor([[1.1, 2.2, 3.3, 4.4], \
|
||||
[1.1, 2.2, 3.3, 4.4], \
|
||||
[5.5, 6.6, 7.7, 8.8]]))
|
||||
desired_outputs = torch.Tensor([[1.1, 2.2], [1.1, 2.2], [5.5, 6.6]])
|
||||
assert torch.allclose(actual_outputs, desired_outputs)
|
||||
|
||||
|
||||
def test_linear_transform_zeros_init():
|
||||
l = pt.transforms.LinearTransform(
|
||||
in_dim=2,
|
||||
out_dim=4,
|
||||
initializer=pt.initializers.ZerosLinearTransformInitializer(),
|
||||
)
|
||||
actual = l.weights
|
||||
desired = torch.zeros(2, 4)
|
||||
assert torch.allclose(actual, desired)
|
||||
|
||||
|
||||
def test_linear_transform_out_dim_first():
|
||||
l = pt.transforms.LinearTransform(
|
||||
in_dim=2,
|
||||
out_dim=4,
|
||||
initializer=pt.initializers.OLTI(out_dim_first=True),
|
||||
)
|
||||
assert l.weights.shape[0] == 4
|
||||
assert l.weights.shape[1] == 2
|
||||
|
||||
|
||||
# Components
|
||||
def test_components_no_initializer():
|
||||
with pytest.raises(TypeError):
|
||||
_ = pt.components.Components(3, None)
|
||||
|
||||
|
||||
def test_components_no_num_components():
|
||||
with pytest.raises(TypeError):
|
||||
_ = pt.components.Components(initializer=pt.initializers.OCI(2))
|
||||
|
||||
|
||||
def test_components_none_num_components():
|
||||
with pytest.raises(TypeError):
|
||||
_ = pt.components.Components(None, initializer=pt.initializers.OCI(2))
|
||||
|
||||
|
||||
def test_components_no_args():
|
||||
with pytest.raises(TypeError):
|
||||
_ = pt.components.Components()
|
||||
|
||||
|
||||
def test_components_zeros_init():
|
||||
c = pt.components.Components(3, pt.initializers.ZCI(2))
|
||||
assert torch.allclose(c.components, torch.zeros(3, 2))
|
||||
|
||||
|
||||
def test_labeled_components_dict_init():
|
||||
c = pt.components.LabeledComponents({0: 3}, pt.initializers.OCI(2))
|
||||
assert torch.allclose(c.components, torch.ones(3, 2))
|
||||
assert torch.allclose(c.labels, torch.zeros(3, dtype=torch.long))
|
||||
|
||||
|
||||
def test_labeled_components_list_init():
|
||||
c = pt.components.LabeledComponents([3], pt.initializers.OCI(2))
|
||||
assert torch.allclose(c.components, torch.ones(3, 2))
|
||||
assert torch.allclose(c.labels, torch.zeros(3, dtype=torch.long))
|
||||
|
||||
|
||||
def test_labeled_components_tuple_init():
|
||||
c = pt.components.LabeledComponents({0: 1, 1: 2}, pt.initializers.OCI(2))
|
||||
assert torch.allclose(c.components, torch.ones(3, 2))
|
||||
assert torch.allclose(c.labels, torch.LongTensor([0, 1, 1]))
|
||||
|
||||
|
||||
# Labels
|
||||
def test_standalone_labels_dict_init():
|
||||
l = pt.components.Labels({0: 3})
|
||||
assert torch.allclose(l.labels, torch.zeros(3, dtype=torch.long))
|
||||
|
||||
|
||||
def test_standalone_labels_list_init():
|
||||
l = pt.components.Labels([3])
|
||||
assert torch.allclose(l.labels, torch.zeros(3, dtype=torch.long))
|
||||
|
||||
|
||||
def test_standalone_labels_tuple_init():
|
||||
l = pt.components.Labels({0: 1, 1: 2})
|
||||
assert torch.allclose(l.labels, torch.LongTensor([0, 1, 1]))
|
||||
|
||||
|
||||
# Losses
|
||||
def test_glvq_loss_int_labels():
|
||||
d = torch.stack([torch.ones(100), torch.zeros(100)], dim=1)
|
||||
labels = torch.tensor([0, 1])
|
||||
targets = torch.ones(100)
|
||||
batch_loss = pt.losses.glvq_loss(distances=d,
|
||||
target_labels=targets,
|
||||
prototype_labels=labels)
|
||||
loss_value = torch.sum(batch_loss, dim=0)
|
||||
assert loss_value == -100
|
||||
|
||||
|
||||
def test_glvq_loss_one_hot_labels():
|
||||
d = torch.stack([torch.ones(100), torch.zeros(100)], dim=1)
|
||||
labels = torch.tensor([[0, 1], [1, 0]])
|
||||
wl = torch.tensor([1, 0])
|
||||
targets = torch.stack([wl for _ in range(100)], dim=0)
|
||||
batch_loss = pt.losses.glvq_loss(distances=d,
|
||||
target_labels=targets,
|
||||
prototype_labels=labels)
|
||||
loss_value = torch.sum(batch_loss, dim=0)
|
||||
assert loss_value == -100
|
||||
|
||||
|
||||
def test_glvq_loss_one_hot_unequal():
|
||||
dlist = [torch.ones(100), torch.zeros(100), torch.zeros(100)]
|
||||
d = torch.stack(dlist, dim=1)
|
||||
labels = torch.tensor([[0, 1], [1, 0], [1, 0]])
|
||||
wl = torch.tensor([1, 0])
|
||||
targets = torch.stack([wl for _ in range(100)], dim=0)
|
||||
batch_loss = pt.losses.glvq_loss(distances=d,
|
||||
target_labels=targets,
|
||||
prototype_labels=labels)
|
||||
loss_value = torch.sum(batch_loss, dim=0)
|
||||
assert loss_value == -100
|
||||
|
||||
|
||||
# Activations
|
||||
class TestActivations(unittest.TestCase):
|
||||
|
||||
def setUp(self):
|
||||
self.flist = ["identity", "sigmoid_beta", "swish_beta"]
|
||||
self.x = torch.randn(1024, 1)
|
||||
|
||||
def test_registry(self):
|
||||
self.assertIsNotNone(pt.nn.ACTIVATIONS)
|
||||
|
||||
def test_funcname_deserialization(self):
|
||||
for funcname in self.flist:
|
||||
f = pt.nn.get_activation(funcname)
|
||||
iscallable = callable(f)
|
||||
self.assertTrue(iscallable)
|
||||
|
||||
def test_callable_deserialization(self):
|
||||
|
||||
def dummy(x, **kwargs):
|
||||
return x
|
||||
|
||||
for f in [dummy, lambda x: x]:
|
||||
f = pt.nn.get_activation(f)
|
||||
iscallable = callable(f)
|
||||
self.assertTrue(iscallable)
|
||||
self.assertEqual(1, f(1))
|
||||
|
||||
def test_unknown_deserialization(self):
|
||||
for funcname in ["blubb", "foobar"]:
|
||||
with self.assertRaises(NameError):
|
||||
_ = pt.nn.get_activation(funcname)
|
||||
|
||||
def test_identity(self):
|
||||
actual = pt.nn.identity(self.x)
|
||||
desired = self.x
|
||||
mismatch = np.testing.assert_array_almost_equal(actual,
|
||||
desired,
|
||||
decimal=5)
|
||||
self.assertIsNone(mismatch)
|
||||
|
||||
def test_sigmoid_beta1(self):
|
||||
actual = pt.nn.sigmoid_beta(self.x, beta=1.0)
|
||||
desired = torch.sigmoid(self.x)
|
||||
mismatch = np.testing.assert_array_almost_equal(actual,
|
||||
desired,
|
||||
decimal=5)
|
||||
self.assertIsNone(mismatch)
|
||||
|
||||
def test_swish_beta1(self):
|
||||
actual = pt.nn.swish_beta(self.x, beta=1.0)
|
||||
desired = self.x * torch.sigmoid(self.x)
|
||||
mismatch = np.testing.assert_array_almost_equal(actual,
|
||||
desired,
|
||||
decimal=5)
|
||||
self.assertIsNone(mismatch)
|
||||
|
||||
def tearDown(self):
|
||||
del self.x
|
||||
|
||||
|
||||
# Competitions
|
||||
class TestCompetitions(unittest.TestCase):
|
||||
|
||||
def setUp(self):
|
||||
pass
|
||||
|
||||
def test_wtac(self):
|
||||
d = torch.tensor([[2.0, 3.0, 1.99, 3.01], [2.0, 3.0, 2.01, 3.0]])
|
||||
labels = torch.tensor([0, 1, 2, 3])
|
||||
competition_layer = pt.competitions.WTAC()
|
||||
actual = competition_layer(d, labels)
|
||||
desired = torch.tensor([2, 0])
|
||||
mismatch = np.testing.assert_array_almost_equal(actual,
|
||||
desired,
|
||||
decimal=5)
|
||||
self.assertIsNone(mismatch)
|
||||
|
||||
def test_wtac_unequal_dist(self):
|
||||
d = torch.tensor([[2.0, 3.0, 4.0], [2.0, 3.0, 1.0]])
|
||||
labels = torch.tensor([0, 1, 1])
|
||||
competition_layer = pt.competitions.WTAC()
|
||||
actual = competition_layer(d, labels)
|
||||
desired = torch.tensor([0, 1])
|
||||
mismatch = np.testing.assert_array_almost_equal(actual,
|
||||
desired,
|
||||
decimal=5)
|
||||
self.assertIsNone(mismatch)
|
||||
|
||||
def test_wtac_one_hot(self):
|
||||
d = torch.tensor([[1.99, 3.01], [3.0, 2.01]])
|
||||
labels = torch.tensor([[0, 1], [1, 0]])
|
||||
competition_layer = pt.competitions.WTAC()
|
||||
actual = competition_layer(d, labels)
|
||||
desired = torch.tensor([[0, 1], [1, 0]])
|
||||
mismatch = np.testing.assert_array_almost_equal(actual,
|
||||
desired,
|
||||
decimal=5)
|
||||
self.assertIsNone(mismatch)
|
||||
|
||||
def test_knnc_k1(self):
|
||||
d = torch.tensor([[2.0, 3.0, 1.99, 3.01], [2.0, 3.0, 2.01, 3.0]])
|
||||
labels = torch.tensor([0, 1, 2, 3])
|
||||
competition_layer = pt.competitions.KNNC(k=1)
|
||||
actual = competition_layer(d, labels)
|
||||
desired = torch.tensor([2, 0])
|
||||
mismatch = np.testing.assert_array_almost_equal(actual,
|
||||
desired,
|
||||
decimal=5)
|
||||
self.assertIsNone(mismatch)
|
||||
|
||||
def tearDown(self):
|
||||
pass
|
||||
|
||||
|
||||
# Pooling
|
||||
class TestPooling(unittest.TestCase):
|
||||
|
||||
def setUp(self):
|
||||
pass
|
||||
|
||||
def test_stratified_min(self):
|
||||
d = torch.tensor([[1.0, 0.0, 2.0, 3.0], [9.0, 8.0, 0, 1]])
|
||||
labels = torch.tensor([0, 0, 1, 2])
|
||||
pooling_layer = pt.pooling.StratifiedMinPooling()
|
||||
actual = pooling_layer(d, labels)
|
||||
desired = torch.tensor([[0.0, 2.0, 3.0], [8.0, 0.0, 1.0]])
|
||||
mismatch = np.testing.assert_array_almost_equal(actual,
|
||||
desired,
|
||||
decimal=5)
|
||||
self.assertIsNone(mismatch)
|
||||
|
||||
def test_stratified_min_one_hot(self):
|
||||
d = torch.tensor([[1.0, 0.0, 2.0, 3.0], [9.0, 8.0, 0, 1]])
|
||||
labels = torch.tensor([0, 0, 1, 2])
|
||||
labels = torch.eye(3)[labels]
|
||||
pooling_layer = pt.pooling.StratifiedMinPooling()
|
||||
actual = pooling_layer(d, labels)
|
||||
desired = torch.tensor([[0.0, 2.0, 3.0], [8.0, 0.0, 1.0]])
|
||||
mismatch = np.testing.assert_array_almost_equal(actual,
|
||||
desired,
|
||||
decimal=5)
|
||||
self.assertIsNone(mismatch)
|
||||
|
||||
def test_stratified_min_trivial(self):
|
||||
d = torch.tensor([[0.0, 2.0, 3.0], [8.0, 0, 1]])
|
||||
labels = torch.tensor([0, 1, 2])
|
||||
pooling_layer = pt.pooling.StratifiedMinPooling()
|
||||
actual = pooling_layer(d, labels)
|
||||
desired = torch.tensor([[0.0, 2.0, 3.0], [8.0, 0.0, 1.0]])
|
||||
mismatch = np.testing.assert_array_almost_equal(actual,
|
||||
desired,
|
||||
decimal=5)
|
||||
self.assertIsNone(mismatch)
|
||||
|
||||
def test_stratified_max(self):
|
||||
d = torch.tensor([[1.0, 0.0, 2.0, 3.0, 9.0], [9.0, 8.0, 0, 1, 7.0]])
|
||||
labels = torch.tensor([0, 0, 3, 2, 0])
|
||||
pooling_layer = pt.pooling.StratifiedMaxPooling()
|
||||
actual = pooling_layer(d, labels)
|
||||
desired = torch.tensor([[9.0, 3.0, 2.0], [9.0, 1.0, 0.0]])
|
||||
mismatch = np.testing.assert_array_almost_equal(actual,
|
||||
desired,
|
||||
decimal=5)
|
||||
self.assertIsNone(mismatch)
|
||||
|
||||
def test_stratified_max_one_hot(self):
|
||||
d = torch.tensor([[1.0, 0.0, 2.0, 3.0, 9.0], [9.0, 8.0, 0, 1, 7.0]])
|
||||
labels = torch.tensor([0, 0, 2, 1, 0])
|
||||
labels = torch.nn.functional.one_hot(labels, num_classes=3)
|
||||
pooling_layer = pt.pooling.StratifiedMaxPooling()
|
||||
actual = pooling_layer(d, labels)
|
||||
desired = torch.tensor([[9.0, 3.0, 2.0], [9.0, 1.0, 0.0]])
|
||||
mismatch = np.testing.assert_array_almost_equal(actual,
|
||||
desired,
|
||||
decimal=5)
|
||||
self.assertIsNone(mismatch)
|
||||
|
||||
def test_stratified_sum(self):
|
||||
d = torch.tensor([[1.0, 0.0, 2.0, 3.0], [9.0, 8.0, 0, 1]])
|
||||
labels = torch.LongTensor([0, 0, 1, 2])
|
||||
pooling_layer = pt.pooling.StratifiedSumPooling()
|
||||
actual = pooling_layer(d, labels)
|
||||
desired = torch.tensor([[1.0, 2.0, 3.0], [17.0, 0.0, 1.0]])
|
||||
mismatch = np.testing.assert_array_almost_equal(actual,
|
||||
desired,
|
||||
decimal=5)
|
||||
self.assertIsNone(mismatch)
|
||||
|
||||
def test_stratified_sum_one_hot(self):
|
||||
d = torch.tensor([[1.0, 0.0, 2.0, 3.0], [9.0, 8.0, 0, 1]])
|
||||
labels = torch.tensor([0, 0, 1, 2])
|
||||
labels = torch.eye(3)[labels]
|
||||
pooling_layer = pt.pooling.StratifiedSumPooling()
|
||||
actual = pooling_layer(d, labels)
|
||||
desired = torch.tensor([[1.0, 2.0, 3.0], [17.0, 0.0, 1.0]])
|
||||
mismatch = np.testing.assert_array_almost_equal(actual,
|
||||
desired,
|
||||
decimal=5)
|
||||
self.assertIsNone(mismatch)
|
||||
|
||||
def test_stratified_prod(self):
|
||||
d = torch.tensor([[1.0, 0.0, 2.0, 3.0, 9.0], [9.0, 8.0, 0, 1, 7.0]])
|
||||
labels = torch.tensor([0, 0, 3, 2, 0])
|
||||
pooling_layer = pt.pooling.StratifiedProdPooling()
|
||||
actual = pooling_layer(d, labels)
|
||||
desired = torch.tensor([[0.0, 3.0, 2.0], [504.0, 1.0, 0.0]])
|
||||
mismatch = np.testing.assert_array_almost_equal(actual,
|
||||
desired,
|
||||
decimal=5)
|
||||
self.assertIsNone(mismatch)
|
||||
|
||||
def tearDown(self):
|
||||
pass
|
||||
|
||||
|
||||
# Distances
|
||||
class TestDistances(unittest.TestCase):
|
||||
|
||||
def setUp(self):
|
||||
self.nx, self.mx = 32, 2048
|
||||
self.ny, self.my = 8, 2048
|
||||
self.x = torch.randn(self.nx, self.mx)
|
||||
self.y = torch.randn(self.ny, self.my)
|
||||
|
||||
def test_manhattan(self):
|
||||
actual = pt.distances.lpnorm_distance(self.x, self.y, p=1)
|
||||
desired = torch.empty(self.nx, self.ny)
|
||||
for i in range(self.nx):
|
||||
for j in range(self.ny):
|
||||
desired[i][j] = torch.nn.functional.pairwise_distance(
|
||||
self.x[i].reshape(1, -1),
|
||||
self.y[j].reshape(1, -1),
|
||||
p=1,
|
||||
keepdim=False,
|
||||
)
|
||||
mismatch = np.testing.assert_array_almost_equal(actual,
|
||||
desired,
|
||||
decimal=2)
|
||||
self.assertIsNone(mismatch)
|
||||
|
||||
def test_euclidean(self):
|
||||
actual = pt.distances.euclidean_distance(self.x, self.y)
|
||||
desired = torch.empty(self.nx, self.ny)
|
||||
for i in range(self.nx):
|
||||
for j in range(self.ny):
|
||||
desired[i][j] = torch.nn.functional.pairwise_distance(
|
||||
self.x[i].reshape(1, -1),
|
||||
self.y[j].reshape(1, -1),
|
||||
p=2,
|
||||
keepdim=False,
|
||||
)
|
||||
mismatch = np.testing.assert_array_almost_equal(actual,
|
||||
desired,
|
||||
decimal=3)
|
||||
self.assertIsNone(mismatch)
|
||||
|
||||
def test_squared_euclidean(self):
|
||||
actual = pt.distances.squared_euclidean_distance(self.x, self.y)
|
||||
desired = torch.empty(self.nx, self.ny)
|
||||
for i in range(self.nx):
|
||||
for j in range(self.ny):
|
||||
desired[i][j] = (torch.nn.functional.pairwise_distance(
|
||||
self.x[i].reshape(1, -1),
|
||||
self.y[j].reshape(1, -1),
|
||||
p=2,
|
||||
keepdim=False,
|
||||
)**2)
|
||||
mismatch = np.testing.assert_array_almost_equal(actual,
|
||||
desired,
|
||||
decimal=2)
|
||||
self.assertIsNone(mismatch)
|
||||
|
||||
def test_lpnorm_p0(self):
|
||||
actual = pt.distances.lpnorm_distance(self.x, self.y, p=0)
|
||||
desired = torch.empty(self.nx, self.ny)
|
||||
for i in range(self.nx):
|
||||
for j in range(self.ny):
|
||||
desired[i][j] = torch.nn.functional.pairwise_distance(
|
||||
self.x[i].reshape(1, -1),
|
||||
self.y[j].reshape(1, -1),
|
||||
p=0,
|
||||
keepdim=False,
|
||||
)
|
||||
mismatch = np.testing.assert_array_almost_equal(actual,
|
||||
desired,
|
||||
decimal=4)
|
||||
self.assertIsNone(mismatch)
|
||||
|
||||
def test_lpnorm_p2(self):
|
||||
actual = pt.distances.lpnorm_distance(self.x, self.y, p=2)
|
||||
desired = torch.empty(self.nx, self.ny)
|
||||
for i in range(self.nx):
|
||||
for j in range(self.ny):
|
||||
desired[i][j] = torch.nn.functional.pairwise_distance(
|
||||
self.x[i].reshape(1, -1),
|
||||
self.y[j].reshape(1, -1),
|
||||
p=2,
|
||||
keepdim=False,
|
||||
)
|
||||
mismatch = np.testing.assert_array_almost_equal(actual,
|
||||
desired,
|
||||
decimal=4)
|
||||
self.assertIsNone(mismatch)
|
||||
|
||||
def test_lpnorm_p3(self):
|
||||
actual = pt.distances.lpnorm_distance(self.x, self.y, p=3)
|
||||
desired = torch.empty(self.nx, self.ny)
|
||||
for i in range(self.nx):
|
||||
for j in range(self.ny):
|
||||
desired[i][j] = torch.nn.functional.pairwise_distance(
|
||||
self.x[i].reshape(1, -1),
|
||||
self.y[j].reshape(1, -1),
|
||||
p=3,
|
||||
keepdim=False,
|
||||
)
|
||||
mismatch = np.testing.assert_array_almost_equal(actual,
|
||||
desired,
|
||||
decimal=4)
|
||||
self.assertIsNone(mismatch)
|
||||
|
||||
def test_lpnorm_pinf(self):
|
||||
actual = pt.distances.lpnorm_distance(self.x, self.y, p=float("inf"))
|
||||
desired = torch.empty(self.nx, self.ny)
|
||||
for i in range(self.nx):
|
||||
for j in range(self.ny):
|
||||
desired[i][j] = torch.nn.functional.pairwise_distance(
|
||||
self.x[i].reshape(1, -1),
|
||||
self.y[j].reshape(1, -1),
|
||||
p=float("inf"),
|
||||
keepdim=False,
|
||||
)
|
||||
mismatch = np.testing.assert_array_almost_equal(actual,
|
||||
desired,
|
||||
decimal=4)
|
||||
self.assertIsNone(mismatch)
|
||||
|
||||
def test_omega_identity(self):
|
||||
omega = torch.eye(self.mx, self.my)
|
||||
actual = pt.distances.omega_distance(self.x, self.y, omega=omega)
|
||||
desired = torch.empty(self.nx, self.ny)
|
||||
for i in range(self.nx):
|
||||
for j in range(self.ny):
|
||||
desired[i][j] = (torch.nn.functional.pairwise_distance(
|
||||
self.x[i].reshape(1, -1),
|
||||
self.y[j].reshape(1, -1),
|
||||
p=2,
|
||||
keepdim=False,
|
||||
)**2)
|
||||
mismatch = np.testing.assert_array_almost_equal(actual,
|
||||
desired,
|
||||
decimal=2)
|
||||
self.assertIsNone(mismatch)
|
||||
|
||||
def test_lomega_identity(self):
|
||||
omega = torch.eye(self.mx, self.my)
|
||||
omegas = torch.stack([omega for _ in range(self.ny)], dim=0)
|
||||
actual = pt.distances.lomega_distance(self.x, self.y, omegas=omegas)
|
||||
desired = torch.empty(self.nx, self.ny)
|
||||
for i in range(self.nx):
|
||||
for j in range(self.ny):
|
||||
desired[i][j] = (torch.nn.functional.pairwise_distance(
|
||||
self.x[i].reshape(1, -1),
|
||||
self.y[j].reshape(1, -1),
|
||||
p=2,
|
||||
keepdim=False,
|
||||
)**2)
|
||||
mismatch = np.testing.assert_array_almost_equal(actual,
|
||||
desired,
|
||||
decimal=2)
|
||||
self.assertIsNone(mismatch)
|
||||
|
||||
def tearDown(self):
|
||||
del self.x, self.y
|
@ -1,186 +0,0 @@
|
||||
"""ProtoTorch datasets test suite"""
|
||||
|
||||
import os
|
||||
import unittest
|
||||
|
||||
import numpy as np
|
||||
import torch
|
||||
|
||||
import prototorch as pt
|
||||
from prototorch.datasets.abstract import Dataset, ProtoDataset
|
||||
|
||||
|
||||
class TestAbstract(unittest.TestCase):
|
||||
|
||||
def setUp(self):
|
||||
self.ds = Dataset("./artifacts")
|
||||
|
||||
def test_getitem(self):
|
||||
with self.assertRaises(NotImplementedError):
|
||||
_ = self.ds[0]
|
||||
|
||||
def test_len(self):
|
||||
with self.assertRaises(NotImplementedError):
|
||||
_ = len(self.ds)
|
||||
|
||||
def tearDown(self):
|
||||
del self.ds
|
||||
|
||||
|
||||
class TestProtoDataset(unittest.TestCase):
|
||||
|
||||
def test_download(self):
|
||||
with self.assertRaises(NotImplementedError):
|
||||
_ = ProtoDataset("./artifacts", download=True)
|
||||
|
||||
def test_exists(self):
|
||||
with self.assertRaises(RuntimeError):
|
||||
_ = ProtoDataset("./artifacts", download=False)
|
||||
|
||||
|
||||
class TestNumpyDataset(unittest.TestCase):
|
||||
|
||||
def test_list_init(self):
|
||||
ds = pt.datasets.NumpyDataset([1], [1])
|
||||
self.assertEqual(len(ds), 1)
|
||||
|
||||
def test_numpy_init(self):
|
||||
data = np.random.randn(3, 2)
|
||||
targets = np.array([0, 1, 2])
|
||||
ds = pt.datasets.NumpyDataset(data, targets)
|
||||
self.assertEqual(len(ds), 3)
|
||||
|
||||
|
||||
class TestCSVDataset(unittest.TestCase):
|
||||
|
||||
def setUp(self):
|
||||
data = np.random.rand(100, 4)
|
||||
targets = np.random.randint(2, size=(100, 1))
|
||||
arr = np.hstack([data, targets])
|
||||
if not os.path.exists("./artifacts"):
|
||||
os.mkdir("./artifacts")
|
||||
np.savetxt("./artifacts/test.csv", arr, delimiter=",")
|
||||
|
||||
def test_len(self):
|
||||
ds = pt.datasets.CSVDataset("./artifacts/test.csv")
|
||||
self.assertEqual(len(ds), 100)
|
||||
|
||||
def tearDown(self):
|
||||
os.remove("./artifacts/test.csv")
|
||||
|
||||
|
||||
class TestSpiral(unittest.TestCase):
|
||||
|
||||
def test_init(self):
|
||||
ds = pt.datasets.Spiral(num_samples=10)
|
||||
self.assertEqual(len(ds), 10)
|
||||
|
||||
|
||||
class TestIris(unittest.TestCase):
|
||||
|
||||
def setUp(self):
|
||||
self.ds = pt.datasets.Iris()
|
||||
|
||||
def test_size(self):
|
||||
self.assertEqual(len(self.ds), 150)
|
||||
|
||||
def test_dims(self):
|
||||
self.assertEqual(self.ds.data.shape[1], 4)
|
||||
|
||||
def test_dims_selection(self):
|
||||
ds = pt.datasets.Iris(dims=[0, 1])
|
||||
self.assertEqual(ds.data.shape[1], 2)
|
||||
|
||||
|
||||
class TestBlobs(unittest.TestCase):
|
||||
|
||||
def test_size(self):
|
||||
ds = pt.datasets.Blobs(num_samples=10)
|
||||
self.assertEqual(len(ds), 10)
|
||||
|
||||
|
||||
class TestRandom(unittest.TestCase):
|
||||
|
||||
def test_size(self):
|
||||
ds = pt.datasets.Random(num_samples=10)
|
||||
self.assertEqual(len(ds), 10)
|
||||
|
||||
|
||||
class TestCircles(unittest.TestCase):
|
||||
|
||||
def test_size(self):
|
||||
ds = pt.datasets.Circles(num_samples=10)
|
||||
self.assertEqual(len(ds), 10)
|
||||
|
||||
|
||||
class TestMoons(unittest.TestCase):
|
||||
|
||||
def test_size(self):
|
||||
ds = pt.datasets.Moons(num_samples=10)
|
||||
self.assertEqual(len(ds), 10)
|
||||
|
||||
|
||||
# class TestTecator(unittest.TestCase):
|
||||
# def setUp(self):
|
||||
# self.artifacts_dir = "./artifacts/Tecator"
|
||||
# self._remove_artifacts()
|
||||
|
||||
# def _remove_artifacts(self):
|
||||
# if os.path.exists(self.artifacts_dir):
|
||||
# shutil.rmtree(self.artifacts_dir)
|
||||
|
||||
# def test_download_false(self):
|
||||
# rootdir = self.artifacts_dir.rpartition("/")[0]
|
||||
# self._remove_artifacts()
|
||||
# with self.assertRaises(RuntimeError):
|
||||
# _ = pt.datasets.Tecator(rootdir, download=False)
|
||||
|
||||
# def test_download_caching(self):
|
||||
# rootdir = self.artifacts_dir.rpartition("/")[0]
|
||||
# _ = pt.datasets.Tecator(rootdir, download=True, verbose=False)
|
||||
# _ = pt.datasets.Tecator(rootdir, download=False, verbose=False)
|
||||
|
||||
# def test_repr(self):
|
||||
# rootdir = self.artifacts_dir.rpartition("/")[0]
|
||||
# train = pt.datasets.Tecator(rootdir, download=True, verbose=True)
|
||||
# self.assertTrue("Split: Train" in train.__repr__())
|
||||
|
||||
# def test_download_train(self):
|
||||
# rootdir = self.artifacts_dir.rpartition("/")[0]
|
||||
# train = pt.datasets.Tecator(root=rootdir,
|
||||
# train=True,
|
||||
# download=True,
|
||||
# verbose=False)
|
||||
# train = pt.datasets.Tecator(root=rootdir, download=True, verbose=False)
|
||||
# x_train, y_train = train.data, train.targets
|
||||
# self.assertEqual(x_train.shape[0], 144)
|
||||
# self.assertEqual(y_train.shape[0], 144)
|
||||
# self.assertEqual(x_train.shape[1], 100)
|
||||
|
||||
# def test_download_test(self):
|
||||
# rootdir = self.artifacts_dir.rpartition("/")[0]
|
||||
# test = pt.datasets.Tecator(root=rootdir, train=False, verbose=False)
|
||||
# x_test, y_test = test.data, test.targets
|
||||
# self.assertEqual(x_test.shape[0], 71)
|
||||
# self.assertEqual(y_test.shape[0], 71)
|
||||
# self.assertEqual(x_test.shape[1], 100)
|
||||
|
||||
# def test_class_to_idx(self):
|
||||
# rootdir = self.artifacts_dir.rpartition("/")[0]
|
||||
# test = pt.datasets.Tecator(root=rootdir, train=False, verbose=False)
|
||||
# _ = test.class_to_idx
|
||||
|
||||
# def test_getitem(self):
|
||||
# rootdir = self.artifacts_dir.rpartition("/")[0]
|
||||
# test = pt.datasets.Tecator(root=rootdir, train=False, verbose=False)
|
||||
# x, y = test[0]
|
||||
# self.assertEqual(x.shape[0], 100)
|
||||
# self.assertIsInstance(y, int)
|
||||
|
||||
# def test_loadable_with_dataloader(self):
|
||||
# rootdir = self.artifacts_dir.rpartition("/")[0]
|
||||
# test = pt.datasets.Tecator(root=rootdir, train=False, verbose=False)
|
||||
# _ = torch.utils.data.DataLoader(test, batch_size=64, shuffle=True)
|
||||
|
||||
# def tearDown(self):
|
||||
# self._remove_artifacts()
|
387
tests/test_functions.py
Normal file
387
tests/test_functions.py
Normal file
@ -0,0 +1,387 @@
|
||||
"""ProtoTorch functions test suite."""
|
||||
|
||||
import unittest
|
||||
|
||||
import numpy as np
|
||||
import torch
|
||||
|
||||
from prototorch.functions import (activations, competitions, distances,
|
||||
initializers)
|
||||
|
||||
|
||||
class TestDistances(unittest.TestCase):
|
||||
def setUp(self):
|
||||
self.nx, self.mx = 32, 2048
|
||||
self.ny, self.my = 8, 2048
|
||||
self.x = torch.randn(self.nx, self.mx)
|
||||
self.y = torch.randn(self.ny, self.my)
|
||||
|
||||
def test_manhattan(self):
|
||||
actual = distances.lpnorm_distance(self.x, self.y, p=1)
|
||||
desired = torch.empty(self.nx, self.ny)
|
||||
for i in range(self.nx):
|
||||
for j in range(self.ny):
|
||||
desired[i][j] = torch.nn.functional.pairwise_distance(
|
||||
self.x[i].reshape(1, -1),
|
||||
self.y[j].reshape(1, -1),
|
||||
p=1,
|
||||
keepdim=False,
|
||||
)
|
||||
mismatch = np.testing.assert_array_almost_equal(actual,
|
||||
desired,
|
||||
decimal=2)
|
||||
self.assertIsNone(mismatch)
|
||||
|
||||
def test_euclidean(self):
|
||||
actual = distances.euclidean_distance(self.x, self.y)
|
||||
desired = torch.empty(self.nx, self.ny)
|
||||
for i in range(self.nx):
|
||||
for j in range(self.ny):
|
||||
desired[i][j] = torch.nn.functional.pairwise_distance(
|
||||
self.x[i].reshape(1, -1),
|
||||
self.y[j].reshape(1, -1),
|
||||
p=2,
|
||||
keepdim=False,
|
||||
)
|
||||
mismatch = np.testing.assert_array_almost_equal(actual,
|
||||
desired,
|
||||
decimal=3)
|
||||
self.assertIsNone(mismatch)
|
||||
|
||||
def test_squared_euclidean(self):
|
||||
actual = distances.squared_euclidean_distance(self.x, self.y)
|
||||
desired = torch.empty(self.nx, self.ny)
|
||||
for i in range(self.nx):
|
||||
for j in range(self.ny):
|
||||
desired[i][j] = torch.nn.functional.pairwise_distance(
|
||||
self.x[i].reshape(1, -1),
|
||||
self.y[j].reshape(1, -1),
|
||||
p=2,
|
||||
keepdim=False,
|
||||
)**2
|
||||
mismatch = np.testing.assert_array_almost_equal(actual,
|
||||
desired,
|
||||
decimal=2)
|
||||
self.assertIsNone(mismatch)
|
||||
|
||||
def test_lpnorm_p0(self):
|
||||
actual = distances.lpnorm_distance(self.x, self.y, p=0)
|
||||
desired = torch.empty(self.nx, self.ny)
|
||||
for i in range(self.nx):
|
||||
for j in range(self.ny):
|
||||
desired[i][j] = torch.nn.functional.pairwise_distance(
|
||||
self.x[i].reshape(1, -1),
|
||||
self.y[j].reshape(1, -1),
|
||||
p=0,
|
||||
keepdim=False,
|
||||
)
|
||||
mismatch = np.testing.assert_array_almost_equal(actual,
|
||||
desired,
|
||||
decimal=4)
|
||||
self.assertIsNone(mismatch)
|
||||
|
||||
def test_lpnorm_p2(self):
|
||||
actual = distances.lpnorm_distance(self.x, self.y, p=2)
|
||||
desired = torch.empty(self.nx, self.ny)
|
||||
for i in range(self.nx):
|
||||
for j in range(self.ny):
|
||||
desired[i][j] = torch.nn.functional.pairwise_distance(
|
||||
self.x[i].reshape(1, -1),
|
||||
self.y[j].reshape(1, -1),
|
||||
p=2,
|
||||
keepdim=False,
|
||||
)
|
||||
mismatch = np.testing.assert_array_almost_equal(actual,
|
||||
desired,
|
||||
decimal=4)
|
||||
self.assertIsNone(mismatch)
|
||||
|
||||
def test_lpnorm_p3(self):
|
||||
actual = distances.lpnorm_distance(self.x, self.y, p=3)
|
||||
desired = torch.empty(self.nx, self.ny)
|
||||
for i in range(self.nx):
|
||||
for j in range(self.ny):
|
||||
desired[i][j] = torch.nn.functional.pairwise_distance(
|
||||
self.x[i].reshape(1, -1),
|
||||
self.y[j].reshape(1, -1),
|
||||
p=3,
|
||||
keepdim=False,
|
||||
)
|
||||
mismatch = np.testing.assert_array_almost_equal(actual,
|
||||
desired,
|
||||
decimal=4)
|
||||
self.assertIsNone(mismatch)
|
||||
|
||||
def test_lpnorm_pinf(self):
|
||||
actual = distances.lpnorm_distance(self.x, self.y, p=float('inf'))
|
||||
desired = torch.empty(self.nx, self.ny)
|
||||
for i in range(self.nx):
|
||||
for j in range(self.ny):
|
||||
desired[i][j] = torch.nn.functional.pairwise_distance(
|
||||
self.x[i].reshape(1, -1),
|
||||
self.y[j].reshape(1, -1),
|
||||
p=float('inf'),
|
||||
keepdim=False,
|
||||
)
|
||||
mismatch = np.testing.assert_array_almost_equal(actual,
|
||||
desired,
|
||||
decimal=4)
|
||||
self.assertIsNone(mismatch)
|
||||
|
||||
def test_omega_identity(self):
|
||||
omega = torch.eye(self.mx, self.my)
|
||||
actual = distances.omega_distance(self.x, self.y, omega=omega)
|
||||
desired = torch.empty(self.nx, self.ny)
|
||||
for i in range(self.nx):
|
||||
for j in range(self.ny):
|
||||
desired[i][j] = torch.nn.functional.pairwise_distance(
|
||||
self.x[i].reshape(1, -1),
|
||||
self.y[j].reshape(1, -1),
|
||||
p=2,
|
||||
keepdim=False,
|
||||
)**2
|
||||
mismatch = np.testing.assert_array_almost_equal(actual,
|
||||
desired,
|
||||
decimal=2)
|
||||
self.assertIsNone(mismatch)
|
||||
|
||||
def test_lomega_identity(self):
|
||||
omega = torch.eye(self.mx, self.my)
|
||||
omegas = torch.stack([omega for _ in range(self.ny)], dim=0)
|
||||
actual = distances.lomega_distance(self.x, self.y, omegas=omegas)
|
||||
desired = torch.empty(self.nx, self.ny)
|
||||
for i in range(self.nx):
|
||||
for j in range(self.ny):
|
||||
desired[i][j] = torch.nn.functional.pairwise_distance(
|
||||
self.x[i].reshape(1, -1),
|
||||
self.y[j].reshape(1, -1),
|
||||
p=2,
|
||||
keepdim=False,
|
||||
)**2
|
||||
mismatch = np.testing.assert_array_almost_equal(actual,
|
||||
desired,
|
||||
decimal=2)
|
||||
self.assertIsNone(mismatch)
|
||||
|
||||
def tearDown(self):
|
||||
del self.x, self.y
|
||||
|
||||
|
||||
class TestActivations(unittest.TestCase):
|
||||
def setUp(self):
|
||||
self.x = torch.randn(1024, 1)
|
||||
|
||||
def test_registry(self):
|
||||
self.assertIsNotNone(activations.ACTIVATIONS)
|
||||
|
||||
def test_funcname_deserialization(self):
|
||||
flist = ['identity', 'sigmoid_beta', 'swish_beta']
|
||||
for funcname in flist:
|
||||
f = activations.get_activation(funcname)
|
||||
iscallable = callable(f)
|
||||
self.assertTrue(iscallable)
|
||||
|
||||
def test_callable_deserialization(self):
|
||||
def dummy(x, **kwargs):
|
||||
return x
|
||||
|
||||
for f in [dummy, lambda x: x]:
|
||||
f = activations.get_activation(f)
|
||||
iscallable = callable(f)
|
||||
self.assertTrue(iscallable)
|
||||
self.assertEqual(1, f(1))
|
||||
|
||||
def test_unknown_deserialization(self):
|
||||
for funcname in ['blubb', 'foobar']:
|
||||
with self.assertRaises(NameError):
|
||||
_ = activations.get_activation(funcname)
|
||||
|
||||
def test_identity(self):
|
||||
actual = activations.identity(self.x)
|
||||
desired = self.x
|
||||
mismatch = np.testing.assert_array_almost_equal(actual,
|
||||
desired,
|
||||
decimal=5)
|
||||
self.assertIsNone(mismatch)
|
||||
|
||||
def test_sigmoid_beta1(self):
|
||||
actual = activations.sigmoid_beta(self.x, beta=1)
|
||||
desired = torch.sigmoid(self.x)
|
||||
mismatch = np.testing.assert_array_almost_equal(actual,
|
||||
desired,
|
||||
decimal=5)
|
||||
self.assertIsNone(mismatch)
|
||||
|
||||
def test_swish_beta1(self):
|
||||
actual = activations.swish_beta(self.x, beta=1)
|
||||
desired = self.x * torch.sigmoid(self.x)
|
||||
mismatch = np.testing.assert_array_almost_equal(actual,
|
||||
desired,
|
||||
decimal=5)
|
||||
self.assertIsNone(mismatch)
|
||||
|
||||
def tearDown(self):
|
||||
del self.x
|
||||
|
||||
|
||||
class TestCompetitions(unittest.TestCase):
|
||||
def setUp(self):
|
||||
pass
|
||||
|
||||
def test_wtac(self):
|
||||
d = torch.tensor([[2., 3., 1.99, 3.01], [2., 3., 2.01, 3.]])
|
||||
labels = torch.tensor([0, 1, 2, 3])
|
||||
actual = competitions.wtac(d, labels)
|
||||
desired = torch.tensor([2, 0])
|
||||
mismatch = np.testing.assert_array_almost_equal(actual,
|
||||
desired,
|
||||
decimal=5)
|
||||
self.assertIsNone(mismatch)
|
||||
|
||||
def test_wtac_one_hot(self):
|
||||
d = torch.tensor([[1.99, 3.01], [3., 2.01]])
|
||||
labels = torch.tensor([[0, 1], [1, 0]])
|
||||
actual = competitions.wtac(d, labels)
|
||||
desired = torch.tensor([[0, 1], [1, 0]])
|
||||
mismatch = np.testing.assert_array_almost_equal(actual,
|
||||
desired,
|
||||
decimal=5)
|
||||
self.assertIsNone(mismatch)
|
||||
|
||||
def test_knnc_k1(self):
|
||||
d = torch.tensor([[2., 3., 1.99, 3.01], [2., 3., 2.01, 3.]])
|
||||
labels = torch.tensor([0, 1, 2, 3])
|
||||
actual = competitions.knnc(d, labels, k=1)
|
||||
desired = torch.tensor([2, 0])
|
||||
mismatch = np.testing.assert_array_almost_equal(actual,
|
||||
desired,
|
||||
decimal=5)
|
||||
self.assertIsNone(mismatch)
|
||||
|
||||
def tearDown(self):
|
||||
pass
|
||||
|
||||
|
||||
class TestInitializers(unittest.TestCase):
|
||||
def setUp(self):
|
||||
self.x = torch.tensor(
|
||||
[[0, -1, -2], [10, 11, 12], [0, 0, 0], [2, 2, 2]],
|
||||
dtype=torch.float32)
|
||||
self.y = torch.tensor([0, 0, 1, 1])
|
||||
self.gen = torch.manual_seed(42)
|
||||
|
||||
def test_registry(self):
|
||||
self.assertIsNotNone(initializers.INITIALIZERS)
|
||||
|
||||
def test_funcname_deserialization(self):
|
||||
flist = [
|
||||
'zeros', 'ones', 'rand', 'randn', 'stratified_mean',
|
||||
'stratified_random'
|
||||
]
|
||||
for funcname in flist:
|
||||
f = initializers.get_initializer(funcname)
|
||||
iscallable = callable(f)
|
||||
self.assertTrue(iscallable)
|
||||
|
||||
def test_callable_deserialization(self):
|
||||
def dummy(x):
|
||||
return x
|
||||
|
||||
for f in [dummy, lambda x: x]:
|
||||
f = initializers.get_initializer(f)
|
||||
iscallable = callable(f)
|
||||
self.assertTrue(iscallable)
|
||||
self.assertEqual(1, f(1))
|
||||
|
||||
def test_unknown_deserialization(self):
|
||||
for funcname in ['blubb', 'foobar']:
|
||||
with self.assertRaises(NameError):
|
||||
_ = initializers.get_initializer(funcname)
|
||||
|
||||
def test_zeros(self):
|
||||
pdist = torch.tensor([1, 1])
|
||||
actual, _ = initializers.zeros(self.x, self.y, pdist)
|
||||
desired = torch.zeros(2, 3)
|
||||
mismatch = np.testing.assert_array_almost_equal(actual,
|
||||
desired,
|
||||
decimal=5)
|
||||
self.assertIsNone(mismatch)
|
||||
|
||||
def test_ones(self):
|
||||
pdist = torch.tensor([1, 1])
|
||||
actual, _ = initializers.ones(self.x, self.y, pdist)
|
||||
desired = torch.ones(2, 3)
|
||||
mismatch = np.testing.assert_array_almost_equal(actual,
|
||||
desired,
|
||||
decimal=5)
|
||||
self.assertIsNone(mismatch)
|
||||
|
||||
def test_rand(self):
|
||||
pdist = torch.tensor([1, 1])
|
||||
actual, _ = initializers.rand(self.x, self.y, pdist)
|
||||
desired = torch.rand(2, 3, generator=torch.manual_seed(42))
|
||||
mismatch = np.testing.assert_array_almost_equal(actual,
|
||||
desired,
|
||||
decimal=5)
|
||||
self.assertIsNone(mismatch)
|
||||
|
||||
def test_randn(self):
|
||||
pdist = torch.tensor([1, 1])
|
||||
actual, _ = initializers.randn(self.x, self.y, pdist)
|
||||
desired = torch.randn(2, 3, generator=torch.manual_seed(42))
|
||||
mismatch = np.testing.assert_array_almost_equal(actual,
|
||||
desired,
|
||||
decimal=5)
|
||||
self.assertIsNone(mismatch)
|
||||
|
||||
def test_stratified_mean_equal1(self):
|
||||
pdist = torch.tensor([1, 1])
|
||||
actual, _ = initializers.stratified_mean(self.x, self.y, pdist)
|
||||
desired = torch.tensor([[5., 5., 5.], [1., 1., 1.]])
|
||||
mismatch = np.testing.assert_array_almost_equal(actual,
|
||||
desired,
|
||||
decimal=5)
|
||||
self.assertIsNone(mismatch)
|
||||
|
||||
def test_stratified_random_equal1(self):
|
||||
pdist = torch.tensor([1, 1])
|
||||
actual, _ = initializers.stratified_random(self.x, self.y, pdist)
|
||||
desired = torch.tensor([[0., -1., -2.], [2., 2., 2.]])
|
||||
mismatch = np.testing.assert_array_almost_equal(actual,
|
||||
desired,
|
||||
decimal=5)
|
||||
self.assertIsNone(mismatch)
|
||||
|
||||
def test_stratified_mean_equal2(self):
|
||||
pdist = torch.tensor([2, 2])
|
||||
actual, _ = initializers.stratified_mean(self.x, self.y, pdist)
|
||||
desired = torch.tensor([[5., 5., 5.], [5., 5., 5.], [1., 1., 1.],
|
||||
[1., 1., 1.]])
|
||||
mismatch = np.testing.assert_array_almost_equal(actual,
|
||||
desired,
|
||||
decimal=5)
|
||||
self.assertIsNone(mismatch)
|
||||
|
||||
def test_stratified_mean_unequal(self):
|
||||
pdist = torch.tensor([1, 3])
|
||||
actual, _ = initializers.stratified_mean(self.x, self.y, pdist)
|
||||
desired = torch.tensor([[5., 5., 5.], [1., 1., 1.], [1., 1., 1.],
|
||||
[1., 1., 1.]])
|
||||
mismatch = np.testing.assert_array_almost_equal(actual,
|
||||
desired,
|
||||
decimal=5)
|
||||
self.assertIsNone(mismatch)
|
||||
|
||||
def test_stratified_random_unequal(self):
|
||||
pdist = torch.tensor([1, 3])
|
||||
actual, _ = initializers.stratified_random(self.x, self.y, pdist)
|
||||
desired = torch.tensor([[0., -1., -2.], [2., 2., 2.], [0., 0., 0.],
|
||||
[0., 0., 0.]])
|
||||
mismatch = np.testing.assert_array_almost_equal(actual,
|
||||
desired,
|
||||
decimal=5)
|
||||
self.assertIsNone(mismatch)
|
||||
|
||||
def tearDown(self):
|
||||
del self.x, self.y, self.gen
|
||||
_ = torch.seed()
|
129
tests/test_modules.py
Normal file
129
tests/test_modules.py
Normal file
@ -0,0 +1,129 @@
|
||||
"""ProtoTorch modules test suite."""
|
||||
|
||||
import unittest
|
||||
|
||||
import numpy as np
|
||||
import torch
|
||||
|
||||
from prototorch.modules import prototypes, losses
|
||||
|
||||
|
||||
class TestPrototypes(unittest.TestCase):
|
||||
def setUp(self):
|
||||
self.x = torch.tensor(
|
||||
[[0, -1, -2], [10, 11, 12], [0, 0, 0], [2, 2, 2]],
|
||||
dtype=torch.float32)
|
||||
self.y = torch.tensor([0, 0, 1, 1])
|
||||
self.gen = torch.manual_seed(42)
|
||||
|
||||
def test_addprototypes1d_init_without_input_dim(self):
|
||||
with self.assertRaises(NameError):
|
||||
_ = prototypes.AddPrototypes1D(nclasses=1)
|
||||
|
||||
def test_addprototypes1d_init_without_nclasses(self):
|
||||
with self.assertRaises(NameError):
|
||||
_ = prototypes.AddPrototypes1D(input_dim=1)
|
||||
|
||||
def test_addprototypes1d_init_without_pdist(self):
|
||||
p1 = prototypes.AddPrototypes1D(input_dim=6,
|
||||
nclasses=2,
|
||||
prototypes_per_class=4,
|
||||
prototype_initializer='ones')
|
||||
protos = p1.prototypes
|
||||
actual = protos.detach().numpy()
|
||||
desired = torch.ones(8, 6)
|
||||
mismatch = np.testing.assert_array_almost_equal(actual,
|
||||
desired,
|
||||
decimal=5)
|
||||
self.assertIsNone(mismatch)
|
||||
|
||||
def test_addprototypes1d_init_without_data(self):
|
||||
pdist = [2, 2]
|
||||
p1 = prototypes.AddPrototypes1D(input_dim=3,
|
||||
prototype_distribution=pdist,
|
||||
prototype_initializer='zeros')
|
||||
protos = p1.prototypes
|
||||
actual = protos.detach().numpy()
|
||||
desired = torch.zeros(4, 3)
|
||||
mismatch = np.testing.assert_array_almost_equal(actual,
|
||||
desired,
|
||||
decimal=5)
|
||||
self.assertIsNone(mismatch)
|
||||
|
||||
# def test_addprototypes1d_init_torch_pdist(self):
|
||||
# pdist = torch.tensor([2, 2])
|
||||
# p1 = prototypes.AddPrototypes1D(input_dim=3,
|
||||
# prototype_distribution=pdist,
|
||||
# prototype_initializer='zeros')
|
||||
# protos = p1.prototypes
|
||||
# actual = protos.detach().numpy()
|
||||
# desired = torch.zeros(4, 3)
|
||||
# mismatch = np.testing.assert_array_almost_equal(actual,
|
||||
# desired,
|
||||
# decimal=5)
|
||||
# self.assertIsNone(mismatch)
|
||||
|
||||
def test_addprototypes1d_init_with_ppc(self):
|
||||
p1 = prototypes.AddPrototypes1D(data=[self.x, self.y],
|
||||
prototypes_per_class=2,
|
||||
prototype_initializer='zeros')
|
||||
protos = p1.prototypes
|
||||
actual = protos.detach().numpy()
|
||||
desired = torch.zeros(4, 3)
|
||||
mismatch = np.testing.assert_array_almost_equal(actual,
|
||||
desired,
|
||||
decimal=5)
|
||||
self.assertIsNone(mismatch)
|
||||
|
||||
def test_addprototypes1d_init_with_pdist(self):
|
||||
p1 = prototypes.AddPrototypes1D(data=[self.x, self.y],
|
||||
prototype_distribution=[6, 9],
|
||||
prototype_initializer='zeros')
|
||||
protos = p1.prototypes
|
||||
actual = protos.detach().numpy()
|
||||
desired = torch.zeros(15, 3)
|
||||
mismatch = np.testing.assert_array_almost_equal(actual,
|
||||
desired,
|
||||
decimal=5)
|
||||
self.assertIsNone(mismatch)
|
||||
|
||||
def test_addprototypes1d_func_initializer(self):
|
||||
def my_initializer(*args, **kwargs):
|
||||
return torch.full((2, 99), 99), torch.tensor([0, 1])
|
||||
|
||||
p1 = prototypes.AddPrototypes1D(input_dim=99,
|
||||
nclasses=2,
|
||||
prototypes_per_class=1,
|
||||
prototype_initializer=my_initializer)
|
||||
protos = p1.prototypes
|
||||
actual = protos.detach().numpy()
|
||||
desired = 99 * torch.ones(2, 99)
|
||||
mismatch = np.testing.assert_array_almost_equal(actual,
|
||||
desired,
|
||||
decimal=5)
|
||||
self.assertIsNone(mismatch)
|
||||
|
||||
def test_addprototypes1d_forward(self):
|
||||
p1 = prototypes.AddPrototypes1D(data=[self.x, self.y])
|
||||
protos, _ = p1()
|
||||
actual = protos.detach().numpy()
|
||||
desired = torch.ones(2, 3)
|
||||
mismatch = np.testing.assert_array_almost_equal(actual,
|
||||
desired,
|
||||
decimal=5)
|
||||
self.assertIsNone(mismatch)
|
||||
|
||||
def tearDown(self):
|
||||
del self.x, self.y, self.gen
|
||||
_ = torch.seed()
|
||||
|
||||
|
||||
class TestLosses(unittest.TestCase):
|
||||
def setUp(self):
|
||||
pass
|
||||
|
||||
def test_glvqloss_init(self):
|
||||
_ = losses.GLVQLoss()
|
||||
|
||||
def tearDown(self):
|
||||
pass
|
@ -1,47 +0,0 @@
|
||||
"""ProtoTorch utils test suite"""
|
||||
|
||||
import numpy as np
|
||||
import torch
|
||||
|
||||
import prototorch as pt
|
||||
|
||||
|
||||
def test_mesh2d_without_input():
|
||||
mesh, xx, yy = pt.utils.mesh2d(border=2.0, resolution=10)
|
||||
assert mesh.shape[0] == 100
|
||||
assert mesh.shape[1] == 2
|
||||
assert xx.shape[0] == 10
|
||||
assert xx.shape[1] == 10
|
||||
assert yy.shape[0] == 10
|
||||
assert yy.shape[1] == 10
|
||||
assert np.min(xx) == -2.0
|
||||
assert np.max(xx) == 2.0
|
||||
assert np.min(yy) == -2.0
|
||||
assert np.max(yy) == 2.0
|
||||
|
||||
|
||||
def test_mesh2d_with_torch_input():
|
||||
x = 10 * torch.rand(5, 2)
|
||||
mesh, xx, yy = pt.utils.mesh2d(x, border=0.0, resolution=100)
|
||||
assert mesh.shape[0] == 100 * 100
|
||||
assert mesh.shape[1] == 2
|
||||
assert xx.shape[0] == 100
|
||||
assert xx.shape[1] == 100
|
||||
assert yy.shape[0] == 100
|
||||
assert yy.shape[1] == 100
|
||||
assert np.min(xx) == x[:, 0].min()
|
||||
assert np.max(xx) == x[:, 0].max()
|
||||
assert np.min(yy) == x[:, 1].min()
|
||||
assert np.max(yy) == x[:, 1].max()
|
||||
|
||||
|
||||
def test_hex_to_rgb():
|
||||
red_rgb = list(pt.utils.hex_to_rgb(["#ff0000"]))[0]
|
||||
assert red_rgb[0] == 255
|
||||
assert red_rgb[1] == 0
|
||||
assert red_rgb[2] == 0
|
||||
|
||||
|
||||
def test_rgb_to_hex():
|
||||
blue_hex = list(pt.utils.rgb_to_hex([(0, 0, 255)]))[0]
|
||||
assert blue_hex.lower() == "0000ff"
|
15
tox.ini
Normal file
15
tox.ini
Normal file
@ -0,0 +1,15 @@
|
||||
# tox (https://tox.readthedocs.io/) is a tool for running tests
|
||||
# in multiple virtualenvs. This configuration file will run the
|
||||
# test suite on all supported python versions. To use it, "pip install tox"
|
||||
# and then run "tox" from this directory.
|
||||
|
||||
[tox]
|
||||
envlist = py36
|
||||
|
||||
[testenv]
|
||||
deps =
|
||||
numpy
|
||||
unittest-xml-reporting
|
||||
commands =
|
||||
python -m xmlrunner -o reports
|
||||
|
Loading…
x
Reference in New Issue
Block a user